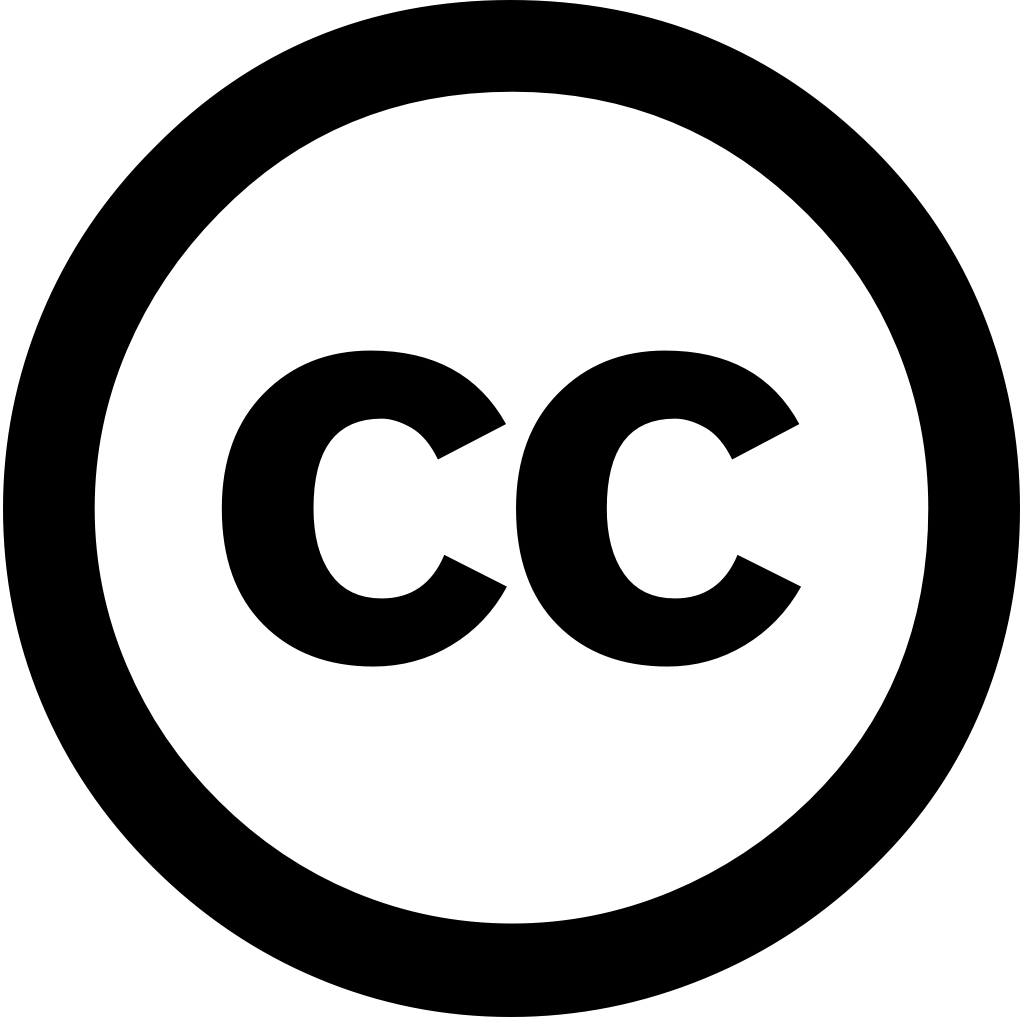
Briefings in Bioinformatics, Год журнала: 2024, Номер 26(1)
Опубликована: Ноя. 22, 2024
Large language models (LLMs) leverage factual knowledge from pretraining. Yet this remains incomplete and sometimes challenging to retrieve-especially in scientific domains not extensively covered pretraining datasets where information is still evolving. Here, we focus on genomics bioinformatics. We confirm expand upon issues with plain ChatGPT functioning as a bioinformatics assistant. Poor data retrieval hallucination lead err, do incorrect sequence manipulations. To address this, propose system basing LLM outputs up-to-date, authoritative facts facilitating LLM-guided analysis. Specifically, introduce NagGPT, middleware tool insert between LLMs databases, designed bridge gaps usage of database application programming interfaces. NagGPT proxies LLM-generated queries, special handling queries. It acts gatekeeper query responses the prompt, redirecting large files but providing synthesized snippet injecting comments steer LLM. A companion OpenAI custom GPT, Genomics Fetcher-Analyzer, connects NagGPT. steers generate run Python code, performing tasks dynamically retrieved dozen common databases (e.g. NCBI, Ensembl, UniProt, WormBase, FlyBase). implement partial mitigations for encountered challenges: detrimental interactions code generation style analysis, confusion identifiers, both actions taken. Our results identify avenues augment assistant and, more broadly, improve accuracy instruction following unmodified LLMs.
Язык: Английский