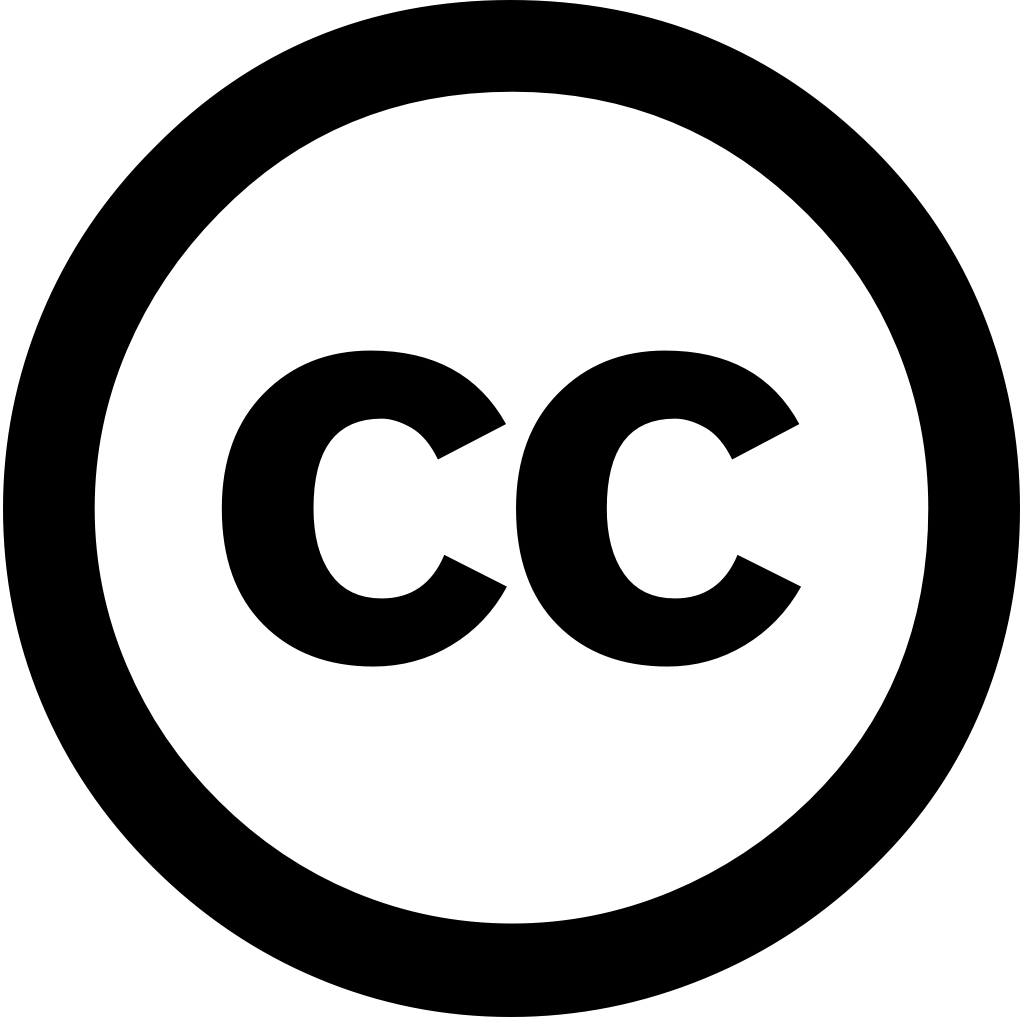
Current Opinion in Electrochemistry, Год журнала: 2024, Номер unknown, С. 101629 - 101629
Опубликована: Дек. 1, 2024
Язык: Английский
Current Opinion in Electrochemistry, Год журнала: 2024, Номер unknown, С. 101629 - 101629
Опубликована: Дек. 1, 2024
Язык: Английский
Physical Chemistry Chemical Physics, Год журнала: 2025, Номер unknown
Опубликована: Янв. 1, 2025
This review discusses machine learning-assisted research on alkali metal-based battery materials, exploring ML processes, models, and applications for designing materials predicting performance.
Язык: Английский
Процитировано
0The Journal of Physical Chemistry C, Год журнала: 2025, Номер unknown
Опубликована: Март 6, 2025
Язык: Английский
Процитировано
0Advanced Functional Materials, Год журнала: 2025, Номер unknown
Опубликована: Март 16, 2025
Abstract High‐entropy nanoparticles (HENPs) present a vast opportunity for the development of advanced electrocatalysts. The optimization their chemical compositions, including careful selection and combination elements, is critical to tailoring HENPs specific catalytic processes. To reduce extensive experimental effort involved in composition optimization, active learning techniques can be utilized predict suggest materials with enhanced electrocatalytic activity. In this study, sub‐2 nm high‐entropy catalysts incorporating eight transition metal elements are developed through an workflow aimed at identifying optimal compositions. Using initial data, approach successfully guided discovery new octonary HENP catalyst state‐of‐the‐art performance hydrogen evolution reaction (HER). Catalyst improved within prediction uncertainty machine model. For oxygen (OER), however, model demonstrated limited predictive accuracy, leading assessment workflow's boundaries. These findings underscore how integration curated data accelerate electrocatalyst discovery, while also highlighting areas further refinement.
Язык: Английский
Процитировано
0ACS Catalysis, Год журнала: 2025, Номер unknown, С. 6067 - 6077
Опубликована: Март 31, 2025
Язык: Английский
Процитировано
0Catalysis Letters, Год журнала: 2025, Номер 155(5)
Опубликована: Апрель 9, 2025
Язык: Английский
Процитировано
0Materials Today Communications, Год журнала: 2025, Номер unknown, С. 112603 - 112603
Опубликована: Апрель 1, 2025
Язык: Английский
Процитировано
0Materials Science and Engineering R Reports, Год журнала: 2025, Номер 165, С. 101010 - 101010
Опубликована: Май 3, 2025
Язык: Английский
Процитировано
0Small Methods, Год журнала: 2024, Номер unknown
Опубликована: Ноя. 30, 2024
Abstract Metal–organic frameworks (MOFs) are highly studied for solar H 2 production from O due to their abundant active sites and open pore channels. Titanium (Ti) Zirconium (Zr) MOFs particularly noted stability optoelectronic properties, resembling conventional metal oxide semiconductors. These allow molecular‐level tuning alter creating opportunities enhance catalytic activity. Introducing defects in the MOF's structure is a versatile strategy modifying molecular topology, morphology, optical electronic properties. This review compiles essential methods synthesizing defect‐oriented MOFs, discussing characterization techniques structural modifications boost It also highlights connection between photocatalytic MOF exploring strategies address current limitations using defective Ti Zr‐based MOFs. Additionally, role of machine learning (ML) predicting properties faster material discovery optimization emphasized. aims identify challenges propose ideas designing future photocatalysts.
Язык: Английский
Процитировано
1Advanced Functional Materials, Год журнала: 2024, Номер unknown
Опубликована: Дек. 18, 2024
Abstract Single‐atom catalysts (SACs) are becoming increasingly recognized as highly promising catalytic materials, distinguished by their exceptional atomic efficiency, superior selectivity, and elevated activity levels. This review offers a detailed comprehensive overview of the recent advancements in SACs, focusing on synthesis strategies, photocatalytic energy conversion applications, advanced characterization techniques. Various synthetic approaches for fabricating atomically dispersed elaborated concisely, emphasizing importance achieving precise regulation compatible supports to ensure strong metal–support interactions. Furthermore, techniques analytical tools illustrated deep exploration mechanistic insights into uniformly SACs. Specifically, different kinds support materials such metal–organic frameworks (MOFs), subset zeolitic imidazolate frameworks, graphitic carbon nitride (g‐C 3 N 4 ) with diverse coordination electronic environments examined. Further, advances computational machine learning transforming SACs development improving predictive accuracy reducing trial‐and‐error methods, thereby accelerating discovery stable active catalysts. Finally, current challenges prospects based MOFs, g‐C addressed, providing valuable continued application these various industrial processes environmental remediation efforts.
Язык: Английский
Процитировано
1Current Opinion in Electrochemistry, Год журнала: 2024, Номер unknown, С. 101629 - 101629
Опубликована: Дек. 1, 2024
Язык: Английский
Процитировано
0