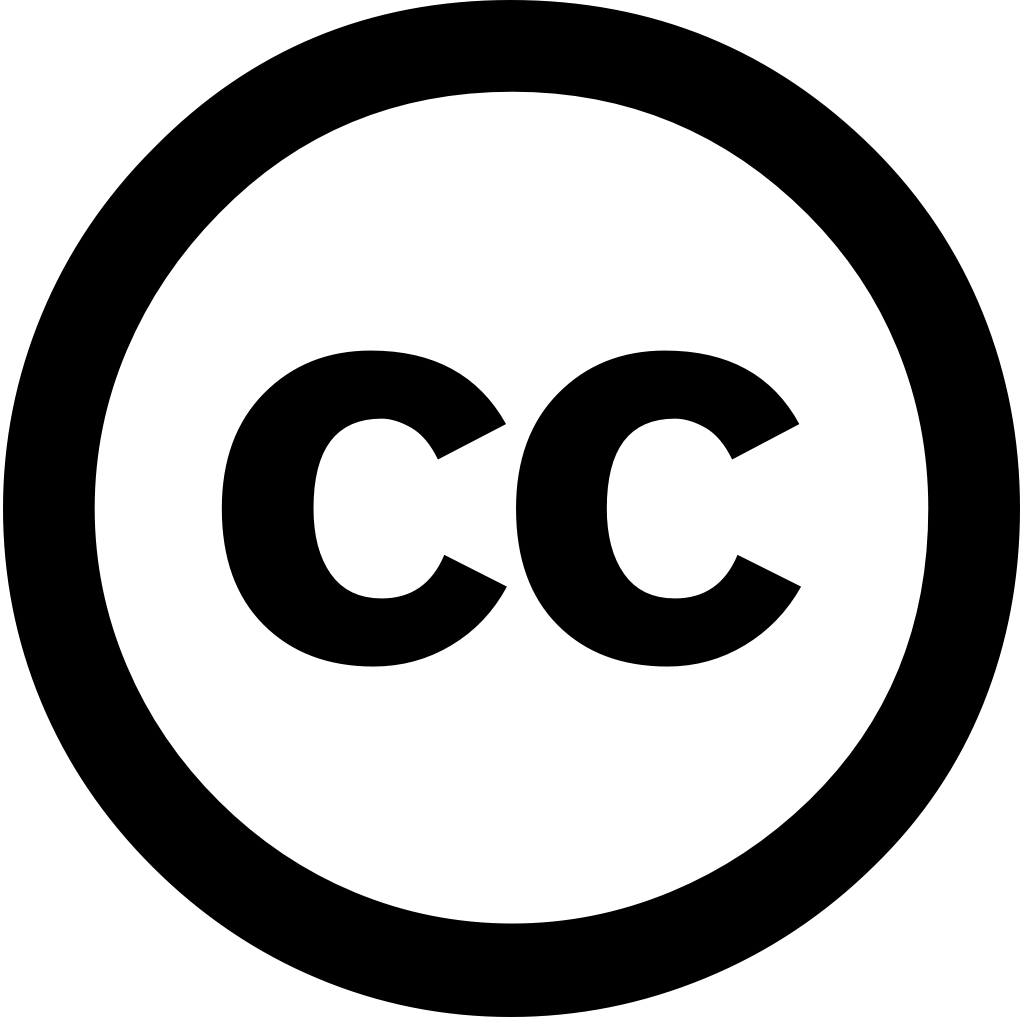
Опубликована: Март 18, 2025
Язык: Английский
Опубликована: Март 18, 2025
Язык: Английский
Remote Sensing, Год журнала: 2025, Номер 17(5), С. 760 - 760
Опубликована: Фев. 22, 2025
Arable land is fundamental to agricultural production and a crucial component of ecosystems. However, its complex texture distribution in remote sensing images make it susceptible interference from other cover types, such as water bodies, roads, buildings, complicating accurate identification. Building on previous research, this study proposes an efficient lightweight CNN-based network, U-MGA, address the challenges feature similarity between arable non-arable areas, insufficient fine-grained extraction, underutilization multi-scale information. Specifically, Multi-Scale Adaptive Segmentation (MSAS) designed during extraction phase provide multi-feature information, supporting model’s reconstruction stage. In phase, introduction Contextual Module (MCM) Group Aggregation Bridge (GAB) significantly enhances efficiency accuracy utilization. The experiments conducted dataset based GF-2 imagery publicly available show that U-MGA outperforms mainstream networks (Unet, A2FPN, Segformer, FTUnetformer, DCSwin, TransUnet) across six evaluation metrics (Overall Accuracy (OA), Precision, Recall, F1-score, Intersection-over-Union (IoU), Kappa coefficient). Thus, provides precise solution for recognition task, which significant importance resource monitoring ecological environmental protection.
Язык: Английский
Процитировано
0Опубликована: Янв. 1, 2025
Язык: Английский
Процитировано
0Artificial Intelligence Review, Год журнала: 2025, Номер 58(5)
Опубликована: Март 3, 2025
Язык: Английский
Процитировано
0Electronics, Год журнала: 2025, Номер 14(5), С. 1040 - 1040
Опубликована: Март 5, 2025
The state monitoring of power load equipment plays a crucial role in ensuring its normal operation. However, densely deployed environments, the target often exhibits low clarity, making real-time warnings challenging. In this study, segmentation and assessment method based on multi-source tensor feature fusion (LSA-MT) is proposed. First, lightweight residual block attention mechanism introduced into backbone network to emphasize key features devices enhance efficiency. Second, 3D edge detail perception module designed facilitate multi-scale while preserving boundary different devices, thereby improving local recognition accuracy. Finally, decomposition reorganization are employed guide visual reconstruction conjunction with images, mapping data utilized for automated fault classification. experimental results demonstrate that LSE-MT produces visually clearer segmentations compared models such as classic UNet++ more recent EGE-UNet when segmenting multiple achieving Dice mIoU scores 92.48 92.90, respectively. Regarding classification across four datasets, average accuracy can reach 92.92%. These findings fully effectiveness LSA-MT alarms grid operation maintenance.
Язык: Английский
Процитировано
0Опубликована: Март 18, 2025
Язык: Английский
Процитировано
0