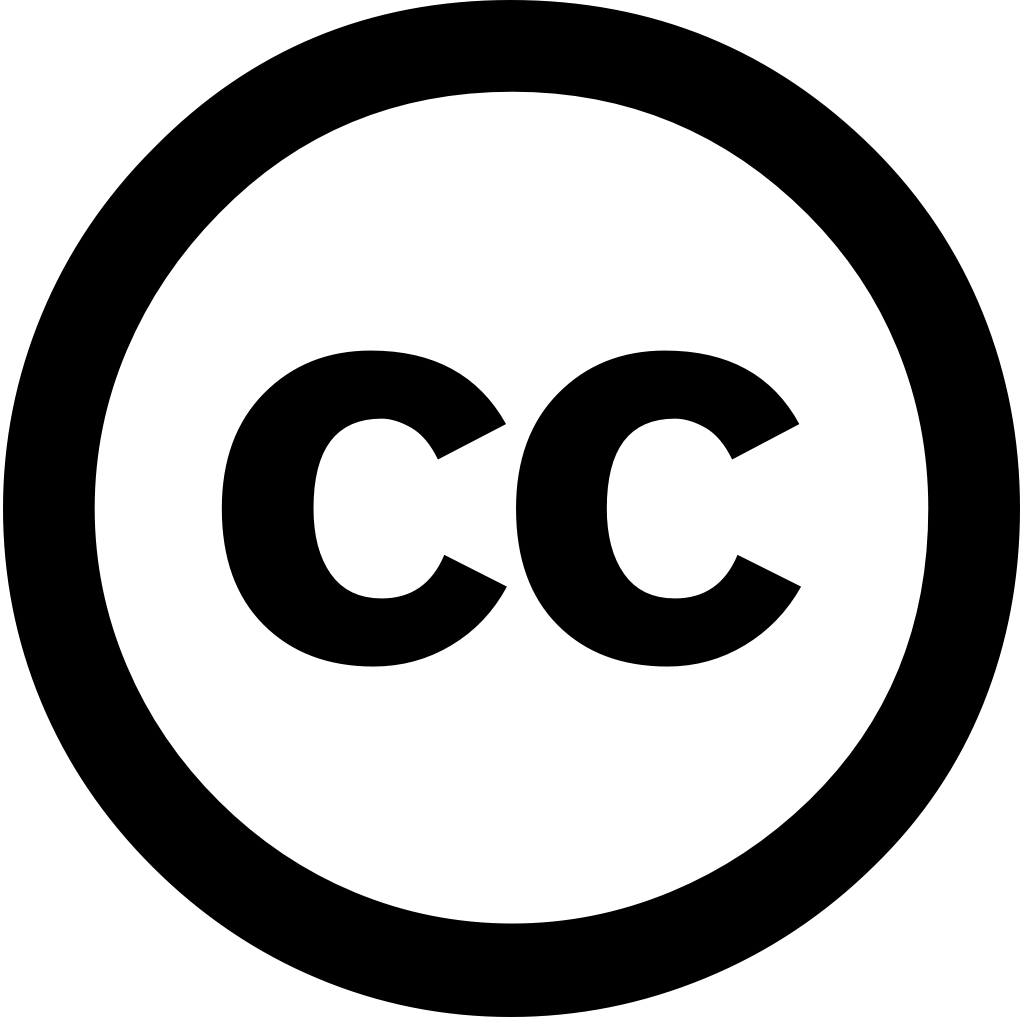
Abdominal Radiology, Год журнала: 2025, Номер unknown
Опубликована: Март 6, 2025
To explore the predictive value of radiomics features extracted from anatomical ROIs in differentiating International Society Urological Pathology (ISUP) grading prostate cancer patients. This study included 1,500 patients a multi-center study. The peripheral zone (PZ) and central gland (CG, transition + zone) were segmented using deep learning algorithms defined as regions interest (ROI) this A total 12,918 image-based T2-weighted imaging (T2WI), apparent diffusion coefficient (ADC), diffusion-weighted (DWI) images these two ROIs. Synthetic minority over-sampling technique (SMOTE) algorithm was used to address class imbalance problem. Feature selection performed Pearson correlation analysis random forest regression. prediction model built classification algorithm. Kruskal-Wallis H test, ANOVA, Chi-Square Test for statistical analysis. 20 ISUP grading-related selected, including 10 PZ ROI CG ROI. On test set, combined exhibited better performance, with an AUC 0.928 (95% CI: 0.872, 0.966), compared alone (AUC: 0.838; 95% 0.722, 0.920) 0.904; 0.851, 0.945). demonstrates that radiomic based on sub-region can contribute enhanced grade prediction. combination GG provide more comprehensive information improved accuracy. Further validation strategy future will enhance its prospects improving decision-making clinical settings.
Язык: Английский