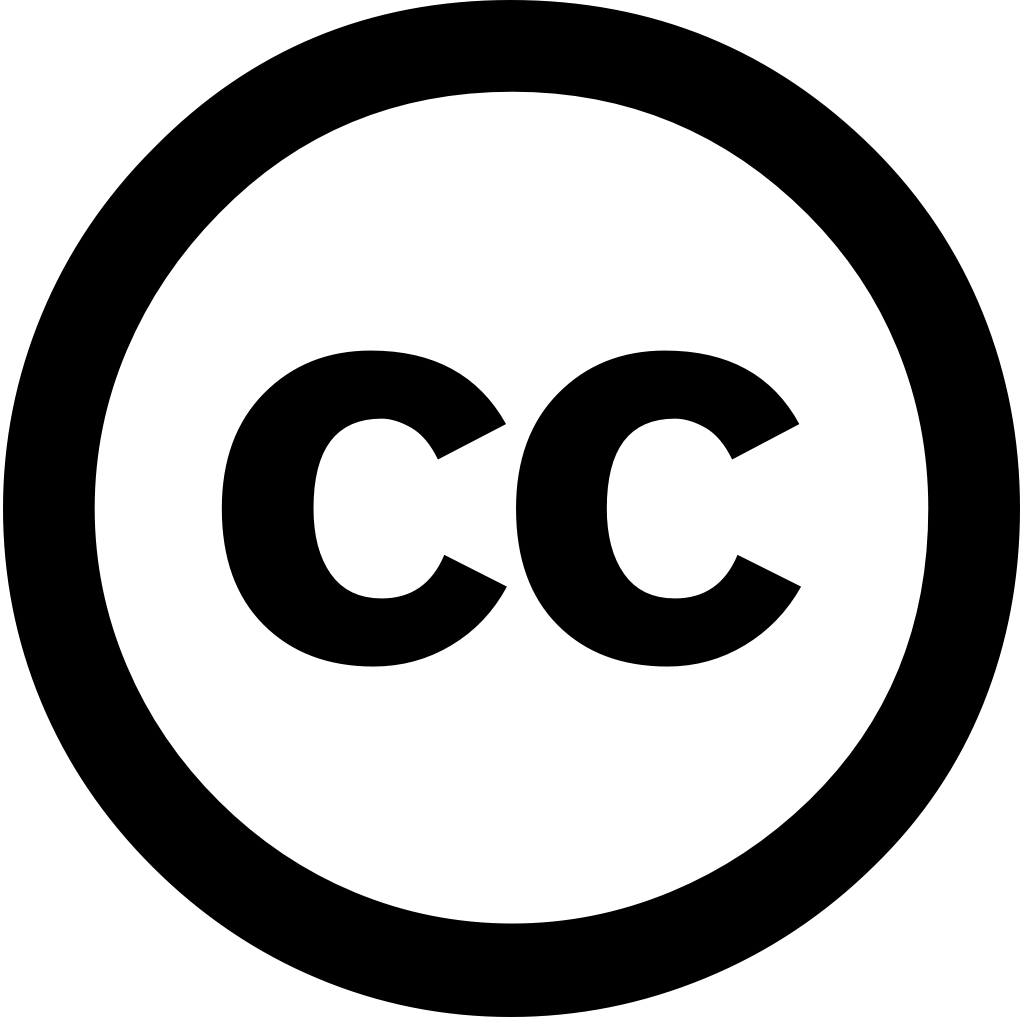
Research Square (Research Square), Год журнала: 2024, Номер unknown
Опубликована: Дек. 17, 2024
Язык: Английский
Research Square (Research Square), Год журнала: 2024, Номер unknown
Опубликована: Дек. 17, 2024
Язык: Английский
European Radiology, Год журнала: 2025, Номер unknown
Опубликована: Янв. 31, 2025
To assess the impact of acquisition and reconstruction factors on robustness radiomics within photon-counting detector CT (PCD-CT). A phantom with twenty-eight texture materials was scanned different including reposition, scan mode (standard vs high-pitch), tube voltage (120 kVp 140 kVp), slice thickness (1.0 mm 0.4 mm), radiation dose level (0.5 mGy, 1.0 3.0 5.0 10.0 mGy), quantum iterative (0/4, 2/4, 4/4), kernel (Qr40, Qr44, Qr48). Thirteen sets virtual monochromatic images at 70-keV were reconstructed. The regions interest drawn rigid registrations. Ninety-three features extracted from each material. reproducibility evaluated using intraclass correlation coefficient (ICC) concordance (CCC). variability assessed by variation (CV) quartile dispersion (QCD). percentage ICC > 0.90 CCC high when repositioned (88.2% 88.2%) changed (87.1% 87.1%), but none high-pitch used. CV < 10% QCD (47.3% 68.8%) (64.2% 71.0%), that low between standard scans (16.1% 26.9%) (19.4% 29.0%). PCD-CT robust to voltage, dose, strength level, kernel, brittle thickness. Question stability against should be fully determined before academic research clinical application. Findings are Clinical relevance influence voxel size set careful attention PCD-CT, allow a higher implementation analysis in routine.
Язык: Английский
Процитировано
1European Radiology, Год журнала: 2025, Номер unknown
Опубликована: Фев. 26, 2025
Язык: Английский
Процитировано
0Cancers, Год журнала: 2025, Номер 17(5), С. 812 - 812
Опубликована: Фев. 26, 2025
Introduction: Following the rapid advances in minimally invasive surgery, there are a multitude of surgical modalities available for resecting rectal cancers. Robotic resections represent current pinnacle approaches. Currently, decisions on modality depend local resources and expertise team. Given limited access to robotic developing tools based pre-operative data that can predict difficulty surgery would streamline efficient utilisation resources. This systematic review aims appraise existing literature artificial intelligence (AI)-driven preoperative MRI analysis prediction identify knowledge gaps promising models warranting further clinical evaluation. Methods: A narrative synthesis were undertaken accordance with PRISMA SWiM guidelines. Systematic searches performed Medline, Embase, CENTRAL Trials register. Studies published between 2012 2024 included where AI was applied imaging adult cancer patients undergoing surgeries, any approach, purpose stratifying difficulty. Data extracted according pre-specified protocol capture study characteristics design; objectives performance outcome metrics summarised. Results: database returned 568 articles, 40 ultimately this review. support assessments identified across eight domains (direct grading, extramural vascular invasion (EMVI), lymph node metastasis (LNM), lymphovascular (LVI), perineural (PNI), T staging, requirement multiple linear stapler firings. For each, at least one model very good (AUC scores >0.80), several showing excellent considerably above threshold. Conclusions: assessment surgeries emerging, progressing development strong many models. These warrant evaluation, which aid personalised approaches ensure adequate
Язык: Английский
Процитировано
0European Radiology Experimental, Год журнала: 2025, Номер 9(1)
Опубликована: Март 22, 2025
Abstract Background Nonenlarged lymph node metastasis (NELNM) of rectal cancer is easily overlooked because these apparently normal nodes are sometimes too small to measure directly using imaging techniques. Radiomic-based multiparametric sequences could predict NELNM based on the primary lesion cancer. We aimed study performance magnetic resonance (MRI) radiomics derived from reduced field-of-view diffusion-weighted (rDWI) and conventional DWI (cDWI) for prediction NELNM. Methods A total 86 patients (60 26 in training test cohorts, respectively), underwent MRI. Radiomic features were extracted whole segmented T2-weighted (T2WI), rDWI, cDWI, both with b -value 800 s/mm 2 apparent diffusion coefficient (ADC) maps (rADC cADC). The radiomic models above methods built assessment status. Their diagnostic performances evaluated comparison subjective evaluation by radiologists. Results rADC demonstrated a significant advantage over predicting cohorts ( p ≤ 0.002). In cohort, exhibited significantly higher area under receiver operating characteristics curve than cADC, cDWIb800, T2WI 0.020) assessing region-of-interest (ROI) delineation while excelling rDWIb800 = 0.0498). Conclusion outperformed those fDWI status cancer, was more advantageous Relevance statement Advanced rDWI excelled cDWI best observed rADC, contrast rDWIb800, T2WI. Key Points help assess For radiomics, ADC map accurate reliable region interest delineation. Graphical
Язык: Английский
Процитировано
0European Radiology, Год журнала: 2025, Номер unknown
Опубликована: Апрель 12, 2025
Язык: Английский
Процитировано
0Academic Radiology, Год журнала: 2024, Номер unknown
Опубликована: Дек. 1, 2024
PurposeThis study aimed to develop and evaluate a machine learning model combining clinical, radiomics, deep features derived from PET/CT imaging predict lymph node metastasis (LNM) in patients with non-small cell lung cancer (NSCLC). The model's interpretability was enhanced using Shapley additive explanations (SHAP).MethodsA total of 248 NSCLC who underwent preoperative scans were included divided into training, test, external validation sets. Radiomics extracted segmented tumor regions on images, generated the ResNet50 architecture. Feature selection performed minimum-redundancy maximum-relevance (mRMR), least absolute shrinkage operator (LASSO) algorithm. Four models—clinical, radiomics (DL_radiomics), combined model—were constructed XGBoost algorithm evaluated based diagnostic performance metrics, including area under receiver operating characteristic curve (AUC), accuracy, F1 score, sensitivity, specificity. Additive exPlanations (SHAP) used for interpretability.ResultsThe achieved highest AUC test set (AUC=0.853), outperforming clinical (AUC=0.758), (AUC=0.831), DL_radiomics (AUC=0.834) models. Decision analysis (DCA) demonstrated that offered greater net benefits. SHAP global interpretation, summary plot indicated ct_original_glrlm_LongRunHighGrayLevelEmphasis, pet_gradient_glcm_lmc1 most important predictions.ConclusionThe model, PET/CT, significantly improved accuracy LNM prediction patients. SHAP-based provided valuable insights decision-making process, enhancing its potential application NSCLC.
Язык: Английский
Процитировано
1Research Square (Research Square), Год журнала: 2024, Номер unknown
Опубликована: Дек. 17, 2024
Язык: Английский
Процитировано
0