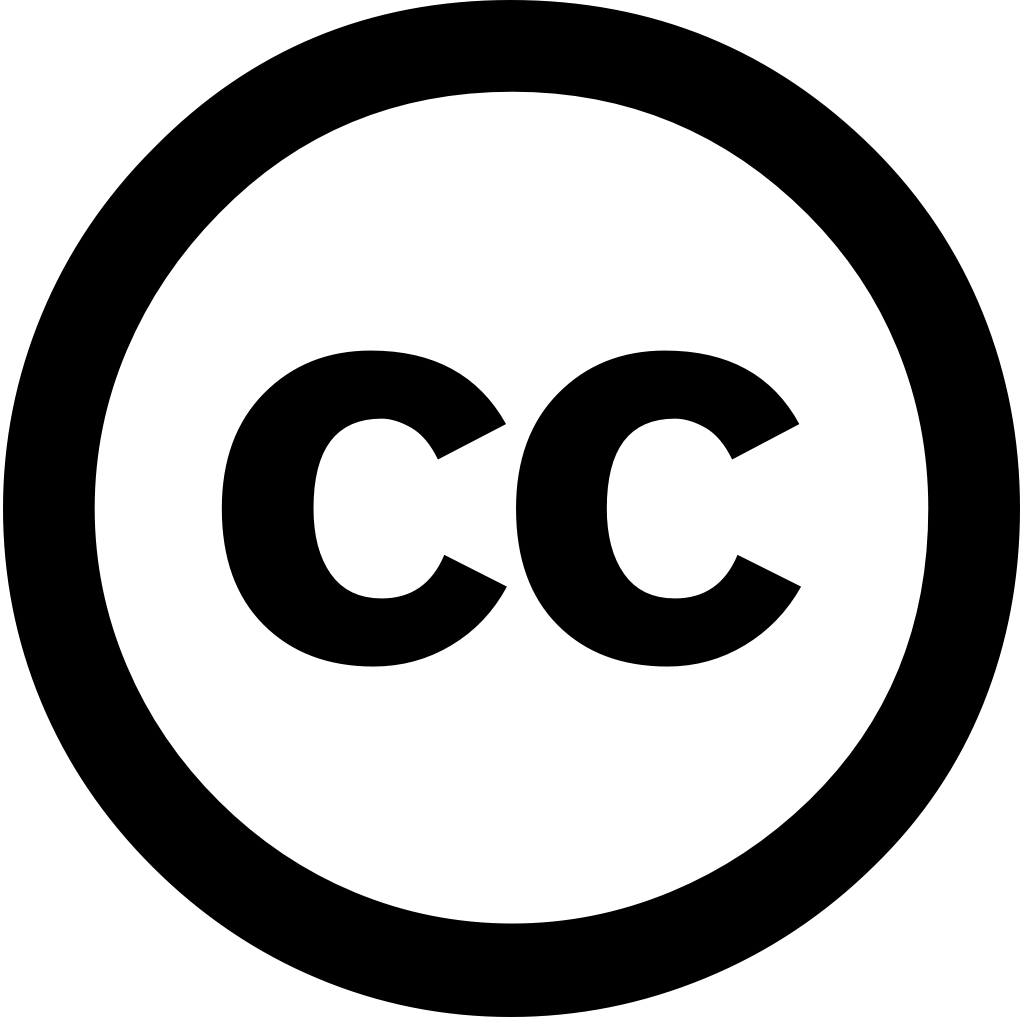
Foods, Год журнала: 2025, Номер 14(8), С. 1306 - 1306
Опубликована: Апрель 9, 2025
Advancements in artificial intelligence, particularly computer vision, have driven the research and development of visual food analysis systems focused primarily on enhancing people’s well-being. Food can be performed at various levels granularity, with segmentation being a major component numerous real-world applications. Deep learning-based methodologies demonstrated promising results segmentation; however, many these approaches demand high computational resources, making them impractical for low-performance devices. In this research, novel, lightweight, deep method semantic is proposed. To achieve this, state-of-the-art DeepLabv3+ model was adapted by optimizing backbone lightweight network EfficientNet-B1, replacing Atrous Spatial Pyramid Pooling (ASPP) neck Cascade Waterfall ASPP (CWASPP), refining encoder output using squeeze-and-excitation attention mechanism. validate method, four publicly available datasets were selected. Additionally, new dataset consisting self-acquired images introduced included validation. The demonstrate that performance achieved significantly lower cost. proposed yields are either better than or comparable to those techniques while requiring costs. conclusion, demonstrates potential learning perform image stand-alone devices, paving way more efficient, cost-effective, scalable
Язык: Английский