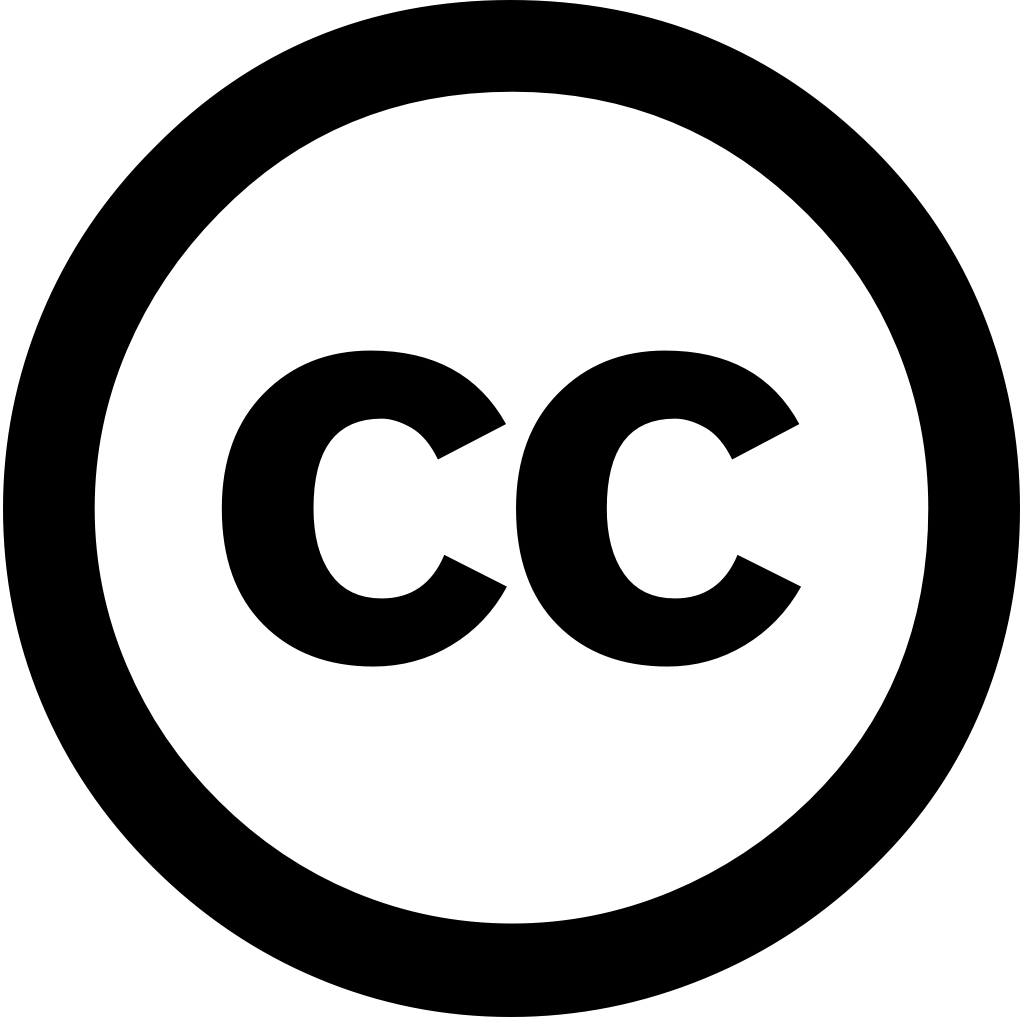
Physics of Fluids, Год журнала: 2025, Номер 37(1)
Опубликована: Янв. 1, 2025
Qualitatively evaluating the fundamental mechanical characteristics of square-fractal-grid (SFG)-generated turbulent flow using piezoelectric thin-film flapping velocimetry (PTFV) is rather time-consuming. More importantly, its sensitivity in detecting high-frequency, fine-scale fluctuations constrained by high-speed camera specifications. To reduce dependency on imaging future PTFV implementations, regression models are trained with supervised machine learning to determine correlation between piezoelectric-generated voltage V and corresponding local equivalent velocity fluctuation. Using tip deflection δ data as predictors responses, respectively, Trilayered Neural Network (TNN) emerges best-performing model compared linear regression, trees, support vector machines, Gaussian process ensembles trees. TNN from (i) lower quarter, (ii) bottom left corner, (iii) central opening SFG-grid provide accurate predictions insert-induced centerline streamwise cross-sectional lateral turbulence intensity root mean square-δ, average errors not exceeding 5%. The output predicted response, which considers small-scale across entire surface, better expresses integral length scale (38% smaller) forcing (270% greater), particularly at corner SFG where eddies significant. Furthermore, effectively captures occasional extensive excitation forces large-scale eddies, resulting a more balanced force distribution. In short, this study paves path for comprehensive expedited dynamics characterization detection via PTFV, potential deployment high Reynolds number flows generated various grid configurations.
Язык: Английский