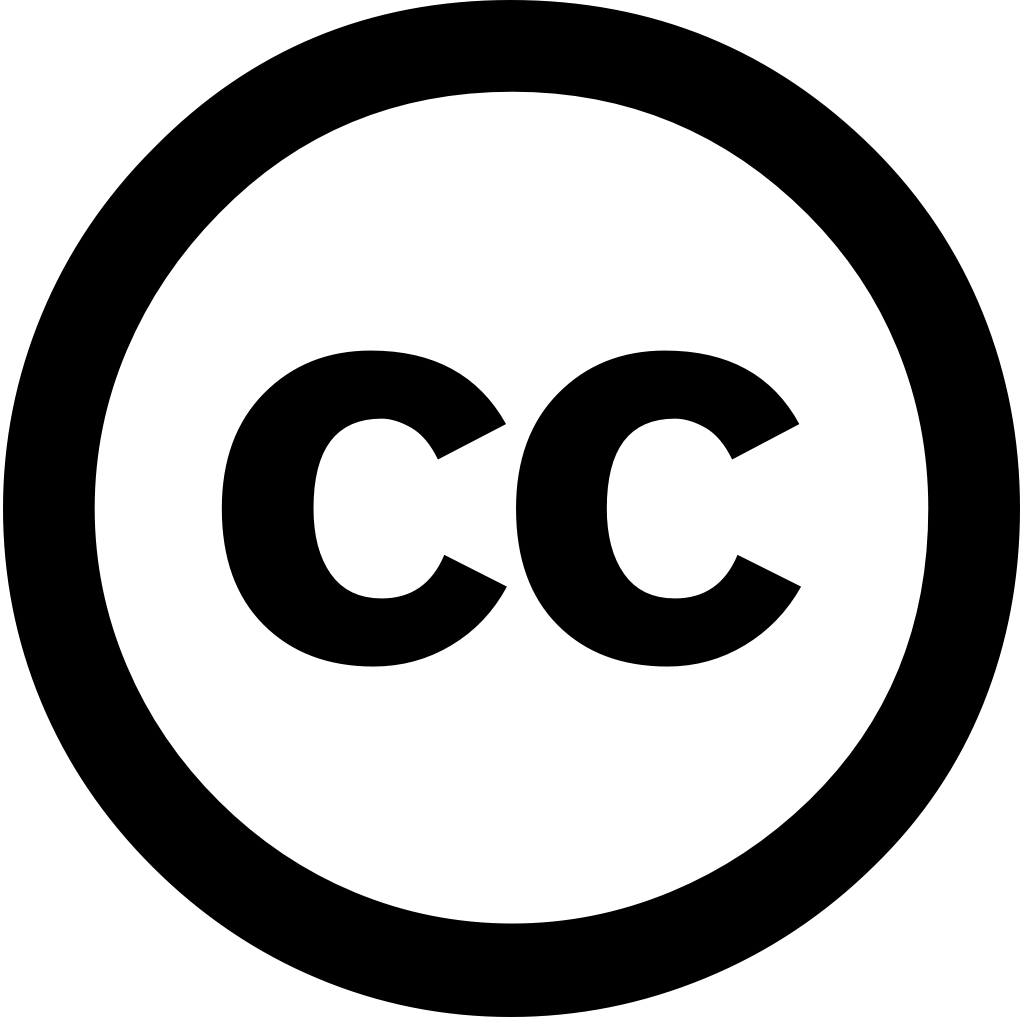
Journal Of Big Data, Год журнала: 2024, Номер 11(1)
Опубликована: Окт. 29, 2024
Mechanical equipment is a vital foundational support for promoting national economic development and widely utilized in key sectors such as aerospace, shipping, construction machinery, energy, petrochemicals, robotics. With the advancement of artificial intelligence industrial intelligence, big data its intelligent analysis provide robust fault prediction health management equipment. Building on existing research, diagnostics mechanical based deep learning have gained significant attention application. However, success relies comprehensive data, which challenging to obtain practical applications where continuous operation essential. Moreover, often operates under varying conditions, leading different distributions training testing. This discrepancy can result low diagnostic accuracy or even failure methods. Deep Transfer Learning (DTL) an emerging machine paradigm that not only leverages advantages (DL) feature representation but also harnesses strengths transfer (TL) knowledge transfer. Consequently, DTL techniques make learning-based diagnosis methods more reliable, robust, applicable, extensive research field diagnosis. paper primarily introduces adversarial-based (ADTL) models, are fundamentally Generative Adversarial Network (GAN). We detailed discussion main ADTL recent developments diagnosis, along with some future challenges prospects.
Язык: Английский