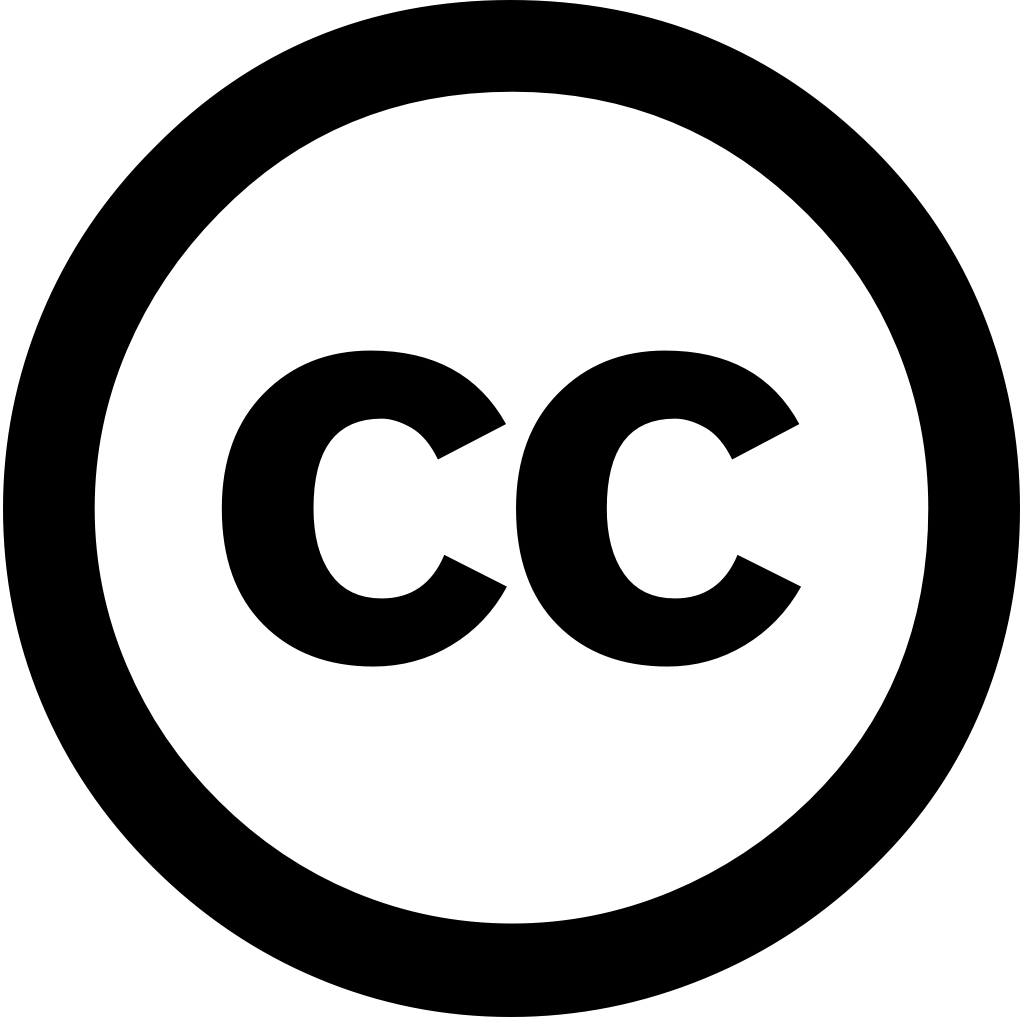
International Journal on Interactive Design and Manufacturing (IJIDeM), Год журнала: 2025, Номер unknown
Опубликована: Янв. 9, 2025
Язык: Английский
International Journal on Interactive Design and Manufacturing (IJIDeM), Год журнала: 2025, Номер unknown
Опубликована: Янв. 9, 2025
Язык: Английский
Materials & Design, Год журнала: 2025, Номер 251, С. 113644 - 113644
Опубликована: Янв. 31, 2025
Язык: Английский
Процитировано
1Materials, Год журнала: 2025, Номер 18(2), С. 408 - 408
Опубликована: Янв. 16, 2025
Additive manufacturing technology, also known as 3D printing, has emerged a viable alternative in modern processes. Unlike traditional methods, which often involve complex mechanical operations that can lead to errors and inconsistencies the final product, additive technology offers new approach enables precise layer-by-layer production with improved geometric accuracy, reduced material consumption increased design flexibility. Geometrical accuracy is critical issue industries such aerospace, automotive, medicine consumer goods, hence importance of following question: dimensional optimisation FDM-manufactured parts be solution for correct design? This paper presents study model printed from four common polymers used fused deposition modelling (FDM) namely ABS (acrylonitrile–butadiene–styrene), PLA (polylactic acid), HIPS (high-impact polystyrene) PETG (polyethylene terephthalate glycol). The results methodology highlight changes need made at stage, depending on direction printing type elements part.
Язык: Английский
Процитировано
0Sensors, Год журнала: 2025, Номер 25(5), С. 1327 - 1327
Опубликована: Фев. 21, 2025
Ensuring the reliability and safety of electrical power systems requires efficient detection defects in high-voltage transmission line insulators, which play a critical role isolation mechanical support. Environmental factors often lead to insulator defects, highlighting need for accurate methods. This paper proposes an enhanced defect approach based on lightweight neural network derived from YOLOv11n architecture. Key innovations include redesigned C3k2 module that incorporates multidimensional dynamic convolutions (ODConv) improved feature extraction, introduction Slimneck reduce model complexity computational cost, application WIoU loss function optimize anchor box handling accelerate convergence. Experimental results demonstrate proposed method outperforms existing models like YOLOv8 YOLOv10 precision, recall, mean average precision (mAP), while maintaining low complexity. provides promising solution real-time, high-accuracy detection, enhancing systems.
Язык: Английский
Процитировано
0Опубликована: Янв. 10, 2025
Язык: Английский
Процитировано
0International Journal on Interactive Design and Manufacturing (IJIDeM), Год журнала: 2025, Номер unknown
Опубликована: Янв. 9, 2025
Язык: Английский
Процитировано
0