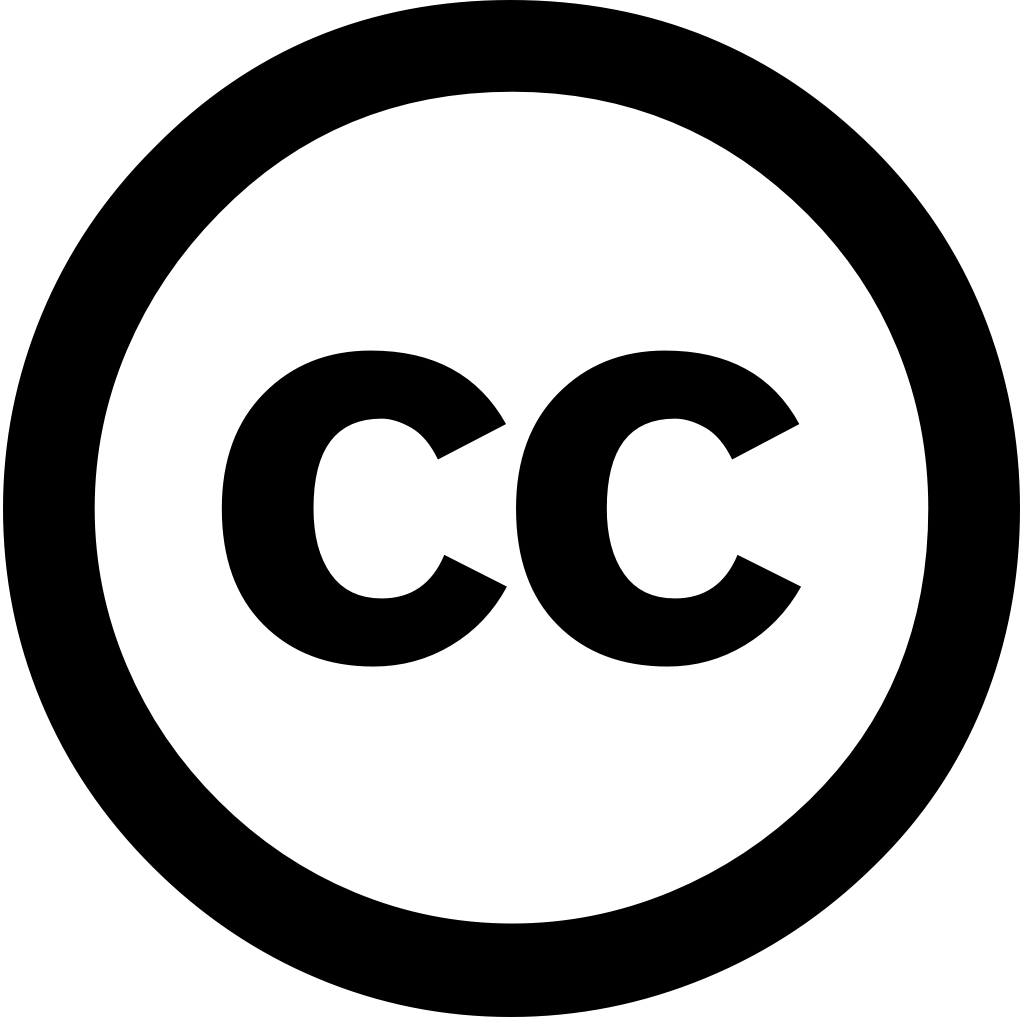
Research Square (Research Square), Год журнала: 2024, Номер unknown
Опубликована: Май 10, 2024
Язык: Английский
Research Square (Research Square), Год журнала: 2024, Номер unknown
Опубликована: Май 10, 2024
Язык: Английский
Knowledge-Based Systems, Год журнала: 2024, Номер 304, С. 112489 - 112489
Опубликована: Сен. 7, 2024
Язык: Английский
Процитировано
3Journal of King Saud University - Computer and Information Sciences, Год журнала: 2024, Номер 36(2), С. 101924 - 101924
Опубликована: Янв. 21, 2024
Edge computing has gained widespread adoption for time-sensitive applications by offloading a portion of IoT system workloads from the cloud to edge nodes. However, limited resources devices hinder service deployment, making auto-scaling crucial improving resource utilization in response dynamic workloads. Recent solutions aim make proactive predicting future and overcoming limitations reactive approaches. These often rely on time-series data analysis machine learning techniques, especially Long Short-Term Memory (LSTM), thanks its accuracy prediction speed. existing suffer oscillation issues, even when using cooling-down strategy. Consequently, efficiency depends model degree scaling actions. This paper proposes novel approach improve deal with issues. Our involves an automatic featurization phase that extracts features workload data, prediction's accuracy. extracted also serve as grid controlling generated experimental results demonstrate effectiveness our accuracy, mitigating phenomena, enhancing overall performance.
Язык: Английский
Процитировано
2IEEE Access, Год журнала: 2024, Номер 12, С. 55248 - 55263
Опубликована: Янв. 1, 2024
Cloud computing has become the cornerstone of modern technology, propelling industries to unprecedented heights with its remarkable and recent advances. However, fundamental challenge for cloud service providers is real-time workload prediction management optimal resource allocation. workloads are characterized by their heterogeneous, unpredictable, fluctuating nature, making this task even more challenging. As a result achievements deep learning (DL) algorithms across diverse fields, scholars have begun embrace approach addressing such challenges. It defacto standard prediction. Unfortunately, DL been widely recognized vulnerability adversarial examples, which poses significant DL-based forecasting models. In study, we utilize established white-box attack generation methods from field computer vision construct examples four cutting-edge regression models, including Recurrent Neural Network (RNN), Long Short-Term Memory (LSTM), Gated Unit (GRU), 1D Convolutional (1D-CNN) attention-based We evaluate our study three benchmark datasets: Google trace, Alibaba Bitbrain. The findings analysis unequivocally indicate that models highly vulnerable attacks. To best knowledge, first conduct systematic research exploring in data center, highlighting inherent hazards both security cost-effectiveness centers. By raising awareness these vulnerabilities, advocate urgent development robust defensive mechanisms enhance constantly evolving technical landscape.
Язык: Английский
Процитировано
2Logistics, Год журнала: 2024, Номер 8(2), С. 56 - 56
Опубликована: Июнь 3, 2024
Background: Efficient inventory management is critical for sustainability in supply chains. However, maintaining adequate levels becomes challenging the face of unpredictable demand patterns. Furthermore, need to disseminate demand-related information throughout a company often relies on cloud services. this method sometimes encounters issues such as limited bandwidth and increased latency. Methods: To address these challenges, our study introduces system that incorporates machine learning algorithm inventory-related uncertainties arising from fluctuations. Our approach involves use an attention mechanism accurate prediction. We combine it with Newsvendor model determine optimal levels. The integrated fog computing facilitate rapid dissemination company. Results: In experiments, we compare proposed conventional estimation based historical data observe consistently outperformed approach. Conclusions: This research novel deep architecture integrates problem. Experiments demonstrate better accuracy comparison existing methods. More studies should be conducted explore its applicability other modeling scenarios.
Язык: Английский
Процитировано
2Electronics, Год журнала: 2024, Номер 13(18), С. 3588 - 3588
Опубликована: Сен. 10, 2024
Cloud infrastructures are designed to provide highly scalable, pay-as-per-use services meet the performance requirements of users. The workload prediction cloud plays a crucial role in proactive auto-scaling and dynamic management resources move toward fine-grained load balancing job scheduling due its ability estimate upcoming workloads. However, users’ diverse usage demands, changing characteristics workloads have become more complex, including not only short-term irregular fluctuation but also long-term variations. This prevents existing workload-prediction methods from fully capturing above characteristics, leading degradation accuracy. To deal with problems, this paper proposes framework based on dual-channel temporal convolutional network transformer (referred as DuCFF) perform prediction. Firstly, DuCFF introduces data preprocessing technology decouple different components implied by combine original form new model inputs. Then, parallel manner, adopts convolution (TCN) channel capture local fluctuations time series Finally, features extracted two channels further fused, is achieved. proposed DuCFF’s was verified various benchmark datasets (i.e., ClarkNet Google) compared nine competitors. Experimental results show that can achieve average improvements 65.2%, 70%, 64.37%, 15%, respectively, terms Mean Absolute Error (MAE), Root Square (RMSE), Percentage (MAPE) R-squared (R2) baseline CNN-LSTM.
Язык: Английский
Процитировано
2Tsinghua Science & Technology, Год журнала: 2024, Номер 30(1), С. 34 - 54
Опубликована: Сен. 11, 2024
Язык: Английский
Процитировано
2Advances in computer and electrical engineering book series, Год журнала: 2024, Номер unknown, С. 105 - 134
Опубликована: Янв. 25, 2024
In a cloud framework, conveyed figuring is flexible and modest area. It permits the development of strong environment that supports pay-per-view while taking client demands into account. The grouping replicated approaches collaborate as one computing system with constrained scope. Spread management's main goal to make it simple provide consent distant geographically distributed resources. Cloud little steps in direction turn dealing massive array issues, among them organizing. There are many methods for determining how correspond volume work PC structure expected complete. According evolving scenario such an effort, scheduler modifies occupations' coordinating situation. suggestion thinking Improvements assignment movement combination planning estimate have been made assessment FCFS least fulfillment time booking expert execution initiatives.
Язык: Английский
Процитировано
1Electronics, Год журнала: 2024, Номер 13(14), С. 2834 - 2834
Опубликована: Июль 18, 2024
In multivariate and multistep time series prediction research, we often face the problems of insufficient spatial feature extraction time-dependent mining historical data, which also brings great challenges to analysis prediction. Inspired by attention mechanism residual module, this study proposes a method based on convolutional-residual gated recurrent hybrid model (CNN-DA-RGRU) with two-layer solve problem in these two stages. Specifically, convolution module proposed is used extract relational features among sequences, can pay more relevant variables give them higher weights eliminate irrelevant features, while loop time-varying block achieve direct connectivity enhance expressive power model, gradient explosion vanishing scenarios, facilitate propagation. Experiments were conducted public datasets using determine hyperparameters, ablation experiments verify effectiveness model; comparing it several models, was found good results series-forecasting tasks.
Язык: Английский
Процитировано
1Lecture notes in computer science, Год журнала: 2024, Номер unknown, С. 47 - 61
Опубликована: Дек. 12, 2024
Язык: Английский
Процитировано
1Research Square (Research Square), Год журнала: 2023, Номер unknown
Опубликована: Июль 7, 2023
Abstract Using cloud computing for Internet of Things (IoT) applications necessitates the transmission all data to centralized structure cloud, thereby leading an increase in network traffic and service time. Consequently, proves impractical latency-sensitive IoT applications. Fog computing, acting as intermediate layer between IoT, ensures low latency such The placement problem, NP-hard problem that determines which node should host each service, represents one major challenges fog paradigm. While lightweight containers have emerged a highly efficient virtualization approach, prior research predominantly employed traditional VM-based architecture computing. Therefore, this study introduces multi-objective optimization approach dynamic container-based accounting cost, latency, energy consumption. Specifically, we propose two-tier framework resource management based on Kubernetes. non-dominated sorting genetic algorithm II (NSGA-II) balances conflicting performance objectives, Empirical results demonstrate proposed method outperforms existing state-of-the-art methods.
Язык: Английский
Процитировано
2