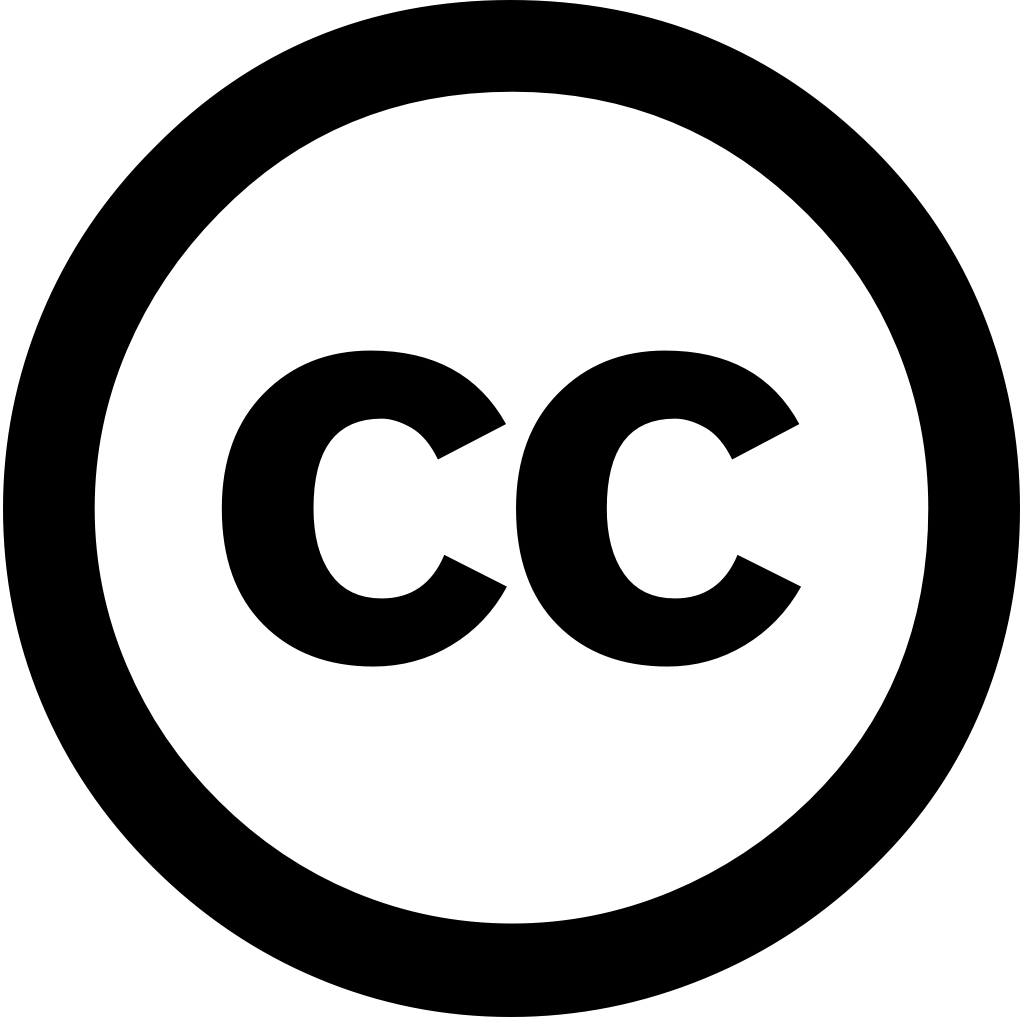
Journal of Groundwater Science and Engineering, Год журнала: 2024, Номер 12(4), С. 428 - 452
Опубликована: Дек. 1, 2024
Язык: Английский
Journal of Groundwater Science and Engineering, Год журнала: 2024, Номер 12(4), С. 428 - 452
Опубликована: Дек. 1, 2024
Язык: Английский
Journal of Hydrology Regional Studies, Год журнала: 2025, Номер 59, С. 102297 - 102297
Опубликована: Март 30, 2025
Язык: Английский
Процитировано
1Hydrological Processes, Год журнала: 2024, Номер 38(10)
Опубликована: Окт. 1, 2024
ABSTRACT Accurate prediction of groundwater recharge is crucial for the sustainable management water resources. Existing models, while effective, still have potential improved accuracy. This study proposed a novel deep forest model—the Feature Selection‐based Deep Forest model (FSDF)—for enhanced prediction. consists three key essential components: feature selection layer, cascade enhancement layer and decision output all designed to enhance accuracy rates. The effectively filtered out redundant features, ensuring that only relevant features are fed into subsequent layer. cascaded was jointly constructed by random forests completely forests, processing data layer‐by‐layer. Finally, predictions rates were produced through an averaging strategy in To further FSDF model's predictive capabilities, Bayesian optimization applied fine‐tuning hyperparameters. performance evaluated compared with existing models using dataset comprising from 1549 wells New South Wales, Australia. exhibited exceptional performance, achieving training 95.91% testing 89.65%. It outperformed adaptive boosting, categorical extreme gradient multiple linear regression 2.02%, 6.98%, 9.05%, 17.02% 2.74% respectively. contributes both hydrological processes identifying factors such as rainfall, surface geology PET, refining greater offers powerful tool accurately forecasting recharge, outperforming traditional models. adaptability makes it applicable different geographical regions managing resources face challenges scarcity climate change.
Язык: Английский
Процитировано
5Journal of Hydrology, Год журнала: 2025, Номер unknown, С. 132668 - 132668
Опубликована: Янв. 1, 2025
Язык: Английский
Процитировано
0Agricultural Water Management, Год журнала: 2025, Номер 309, С. 109347 - 109347
Опубликована: Фев. 2, 2025
Язык: Английский
Процитировано
0Geoscience Frontiers, Год журнала: 2025, Номер unknown, С. 102033 - 102033
Опубликована: Фев. 1, 2025
Язык: Английский
Процитировано
0Journal of Hydrology Regional Studies, Год журнала: 2025, Номер 58, С. 102271 - 102271
Опубликована: Фев. 27, 2025
Язык: Английский
Процитировано
0Sustainability, Год журнала: 2025, Номер 17(5), С. 2250 - 2250
Опубликована: Март 5, 2025
Hydrology relates to many complex challenges due climate variability, limited resources, and especially, increased demands on sustainable management of water soil. Conventional approaches often cannot respond the integrated complexity continuous change inherent in system; hence, researchers have explored advanced data-driven solutions. This review paper revisits how artificial intelligence (AI) is dramatically changing most important facets hydrological research, including soil land surface modeling, streamflow, groundwater forecasting, quality assessment, remote sensing applications resources. In AI techniques could further enhance accuracy texture analysis, moisture estimation, erosion prediction for better management. Advanced models also be used as a tool forecast streamflow levels, therefore providing valuable lead times flood preparedness resource planning transboundary basins. quality, AI-driven methods improve contamination risk enable detection anomalies, track pollutants assist treatment processes regulatory practices. combined with open new perspectives monitoring resources at spatial scale, from forecasting storage variations. paper’s synthesis emphasizes AI’s immense potential hydrology; it covers latest advances future prospects field ensure
Язык: Английский
Процитировано
0Water Resources Management, Год журнала: 2025, Номер unknown
Опубликована: Апрель 2, 2025
Abstract The excessive exploitation of groundwater has led to a significant decline in water levels recent years, emphasizing the need for sustainable resource management strategies. Artificial recharge emerged as an effective solution address this challenge. This study integrates Geographic Information System (GIS) and Multi-Criteria Decision Analysis (MCDA) techniques identify suitable areas artificial Ardabil plain, located northwest Iran. Key parameters, including geology, slope, unsaturated zone thickness, soil texture, specific yield, drainage density, land use, were analyzed. These parameters weighted using three methodologies: Analytic Network Process (ANP), Hierarchy (AHP), Fuzzy (FAHP). final suitability map was developed by overlaying combining information layers. analysis revealed that 53.3%, 6%, 42% plain area classified “very good” according AHP, FAHP, ANP methods, respectively. southern part consistently identified across all characterized pasture lands with young alluvial sediments, deep zone, gentle slopes, low high yield. To evaluate performance these results cross-validated against natural estimates, considering factors influencing level fluctuations rates. Among demonstrated highest consistency making it preferred approach.
Язык: Английский
Процитировано
0Environmental Science and Pollution Research, Год журнала: 2024, Номер unknown
Опубликована: Ноя. 25, 2024
Abstract Managed aquifer recharge (MAR) replenishes groundwater by artificially entering water into subsurface aquifers. This technology improves storage, reduces over-extraction, and ensures security in water-scarce or variable environments. MAR systems are complex, encompassing various components such as soil, meteorological factors, management (GWM), receiving bodies. Over the past decade, utilization of machine learning (ML) methodologies for modeling prediction has increased significantly. review evaluates all supervised, semi-supervised, unsupervised, ensemble ML models employed to predict factors parameters, rendering it most comprehensive contemporary on this subject. study presents a concise integrated overview MAR’s effective approaches, focusing design, suitability quality (WQ) applications, GWM. The paper examines performance measures, input specifications, variety functions GWM, highlights prospects. It also offers suggestions utilizing MAR, addressing issues related physical aspects, technical advancements, case studies. Additionally, previous research ML-based data-driven soft sensing techniques is critically evaluated. concludes that integrating holds significant promise optimizing WQ enhancing efficiency replenishment strategies.
Язык: Английский
Процитировано
2Remote Sensing in Earth Systems Sciences, Год журнала: 2024, Номер unknown
Опубликована: Сен. 28, 2024
Язык: Английский
Процитировано
0