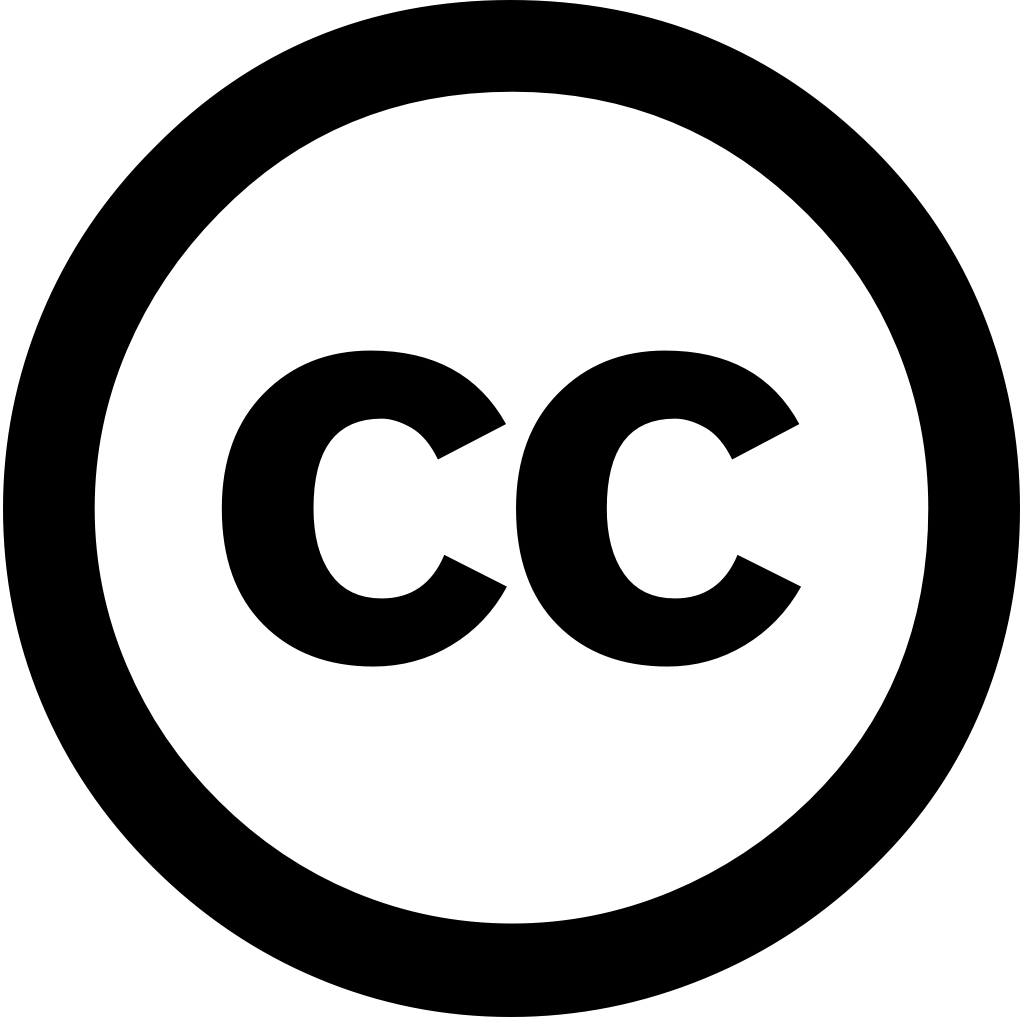
Ain Shams Engineering Journal, Год журнала: 2024, Номер unknown, С. 103127 - 103127
Опубликована: Окт. 1, 2024
Язык: Английский
Ain Shams Engineering Journal, Год журнала: 2024, Номер unknown, С. 103127 - 103127
Опубликована: Окт. 1, 2024
Язык: Английский
Environmental Science and Pollution Research, Год журнала: 2025, Номер unknown
Опубликована: Фев. 13, 2025
Язык: Английский
Процитировано
0Earth Science Informatics, Год журнала: 2025, Номер 18(2)
Опубликована: Май 3, 2025
Язык: Английский
Процитировано
0Archives of Computational Methods in Engineering, Год журнала: 2025, Номер unknown
Опубликована: Март 19, 2025
Язык: Английский
Процитировано
0World Environmental and Water Resources Congress 2011, Год журнала: 2023, Номер unknown, С. 1092 - 1112
Опубликована: Май 18, 2023
Groundwater contamination is a severe problem that deteriorates ecosystems, human health, and plant/animal life. Assessment modeling of groundwater quality possible solution to tackle this problem. In study, 449 samples during the year 2018 in Haryana State, India, were analyzed for 13 water parameters such as pH, electrical conductivity (EC), total hardness (TH), calcium (Ca2+), magnesium (Mg2+), sodium (Na+), potassium (K+), carbonate (CO32–) bicarbonate (HCO3–), nitrate (NO3–), chloride (Cl–), sulfate (SO42–), fluoride (F–). Three machine learning techniques, say generalized linear model (GLM), distributed random trees (DRF), extremely (XRT), applied estimate index (WQI) drinking purposes. The prediction performances these three models are determined by using four error metrics, namely, coefficient determination (R2), root mean square (RMSE), maximum absolute (MAE), squared logarithmic (RMSLE). GLM has shown accuracy (R2 = 0.999964, RMSE 0.759963, MAE 0.525975, RMSLE 0.005606) best estimating WQI compared DRF XRT models. Further, results suggested approximately 53% fall under excellent good category drinking. For better assessment parameters, spatial distribution map also been plotted ArcGIS. expected will contribute effective management worldwide.
Язык: Английский
Процитировано
9Journal of Coastal Conservation, Год журнала: 2023, Номер 27(5)
Опубликована: Сен. 6, 2023
Язык: Английский
Процитировано
8Heliyon, Год журнала: 2024, Номер 10(11), С. e31493 - e31493
Опубликована: Май 17, 2024
Groundwater pollution can occur due to both anthropogenic and natural causes, leading a decline in water quality posing threat human health the environment. The of ground resources with chemical pollutants is often considered. To manage sustainably, ensuring their quantity crucial. Yet, testing groundwater be expensive time-consuming. So, using modeling predict parameters considered an efficient economical method. In this study, we examined three models dry regions by R programming language. random forest (RF) outperformed other developing predictive for quality. Also, multiple linear regression (MLR) model demonstrated strong performance, particularly predicting total hardness (TH) Aran Va Bidgol resources. decision tree (DT) did well but had lower performance than RF parameters. This approach efficacious field effective management protection enables assessment risks related
Язык: Английский
Процитировано
2Groundwater for Sustainable Development, Год журнала: 2024, Номер 27, С. 101329 - 101329
Опубликована: Сен. 6, 2024
Язык: Английский
Процитировано
2Journal of Environmental Management, Год журнала: 2024, Номер 370, С. 122535 - 122535
Опубликована: Сен. 26, 2024
Язык: Английский
Процитировано
2Groundwater for Sustainable Development, Год журнала: 2024, Номер 25, С. 101098 - 101098
Опубликована: Янв. 21, 2024
Язык: Английский
Процитировано
1Acta Geophysica, Год журнала: 2024, Номер unknown
Опубликована: Май 16, 2024
Язык: Английский
Процитировано
0