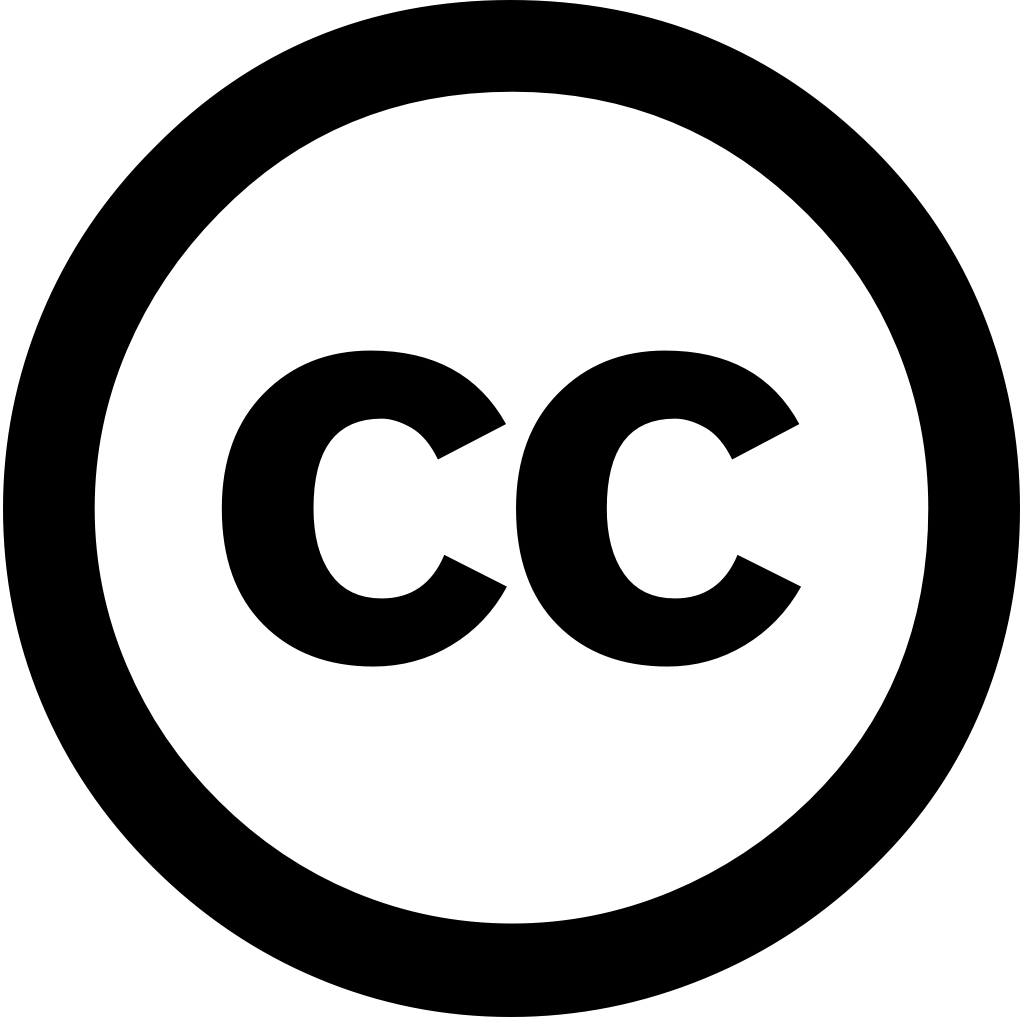
Applied Sciences, Год журнала: 2025, Номер 15(7), С. 3618 - 3618
Опубликована: Март 26, 2025
The identification of the most pertinent site parameters to classify soils in terms their amplification seismic ground motions is still prime interest earthquake engineering and codes. This study investigates many options for improving soil classifications order reduce deviation between “exact” predictions using wave propagation method used codes based on (site) factors. To this end, an exhaustive parametric carried out obtain nonlinear responses sets 324 clay sand columns constitute database neuronal network methods predict regression equations factors parameters. A wide variety combinations are considered study, namely, depth, shear velocity, stiffness underlaying bedrock, intensity frequency content excitation. AFs profiles under multiple records with different intensities contents obtained by propagation, where nonlinearity accounted through equivalent linear model iterative procedure. Then, a Generalized Regression Neural Network (GRNN) determine significant affecting AFs. second neural network, Radial Basis Function (RBF) develop simple practical prediction equations. Both whole period range specific short-, mid-, long-period ranges associated AFs, Fa, Fv, Fl, respectively, considered. results indicate that factor arbitrary profile can be satisfactorily approximated limited number sites record (two six). best parameter pair (PGA; resonance frequency, f0), which leads standard reduction at least 65%. For improved performance, we propose triplet PGA;Vs30;f0 Vs30 being average velocity within upper 30 m below foundation. Most other relevant include fact long periods (Fl) significantly higher than those short or mid soft soils. Finally, it recommended further refine including additional such as spatial configuration adopting more refined models.
Язык: Английский