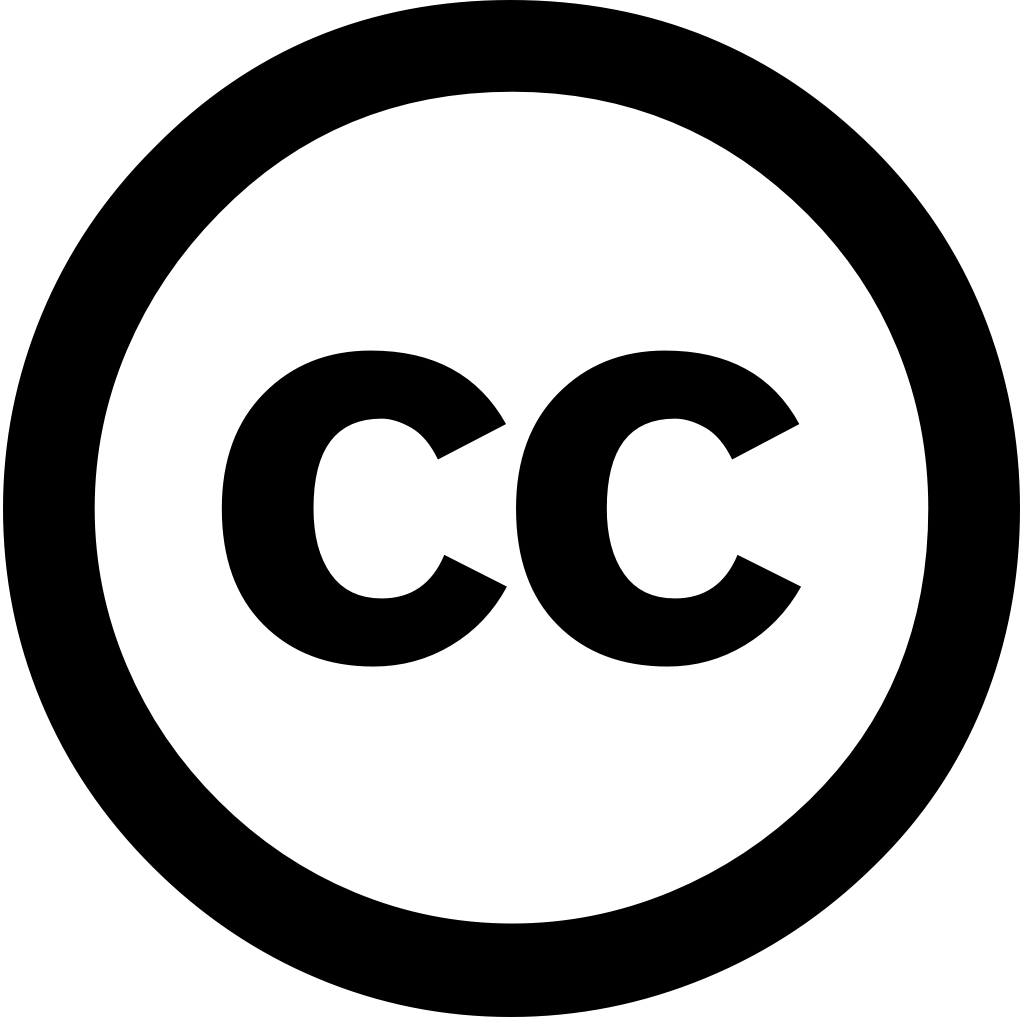
Research Square (Research Square), Год журнала: 2024, Номер unknown
Опубликована: Март 15, 2024
Язык: Английский
Research Square (Research Square), Год журнала: 2024, Номер unknown
Опубликована: Март 15, 2024
Язык: Английский
Knowledge-Based Systems, Год журнала: 2023, Номер 271, С. 110554 - 110554
Опубликована: Апрель 10, 2023
Язык: Английский
Процитировано
92Archives of Computational Methods in Engineering, Год журнала: 2023, Номер 31(1), С. 521 - 549
Опубликована: Авг. 26, 2023
Язык: Английский
Процитировано
28Expert Systems with Applications, Год журнала: 2024, Номер 247, С. 123262 - 123262
Опубликована: Янв. 25, 2024
Язык: Английский
Процитировано
17Computer Science Review, Год журнала: 2025, Номер 56, С. 100727 - 100727
Опубликована: Янв. 18, 2025
Язык: Английский
Процитировано
2Journal of Environmental Management, Год журнала: 2025, Номер 375, С. 124238 - 124238
Опубликована: Янв. 29, 2025
Язык: Английский
Процитировано
2Engineering Science and Technology an International Journal, Год журнала: 2025, Номер 63, С. 101982 - 101982
Опубликована: Фев. 2, 2025
Язык: Английский
Процитировано
2Computers & Electrical Engineering, Год журнала: 2024, Номер 116, С. 109142 - 109142
Опубликована: Фев. 29, 2024
Язык: Английский
Процитировано
8Proceedings of the Institution of Mechanical Engineers Part E Journal of Process Mechanical Engineering, Год журнала: 2025, Номер unknown
Опубликована: Фев. 9, 2025
Submerged friction stir welding (SFSW) under water is a relatively new solid state joining process, which combines heating and mechanical work for deformation to achieve high quality, defect-free joints. In the present research aluminum-6061 alloy has been welded by using SFSW. Tool rotational speed, feed temperature taken as important control variables estimate joint performance in terms of hardness tensile strength. It was observed that average grain size obtained zone around 3.5 μm, maximum 87 HV strength 175 MPa. Predictive models artificial neural networks (ANNs) were developed both strength, followed process optimization utilizing four distinct evolutionary techniques: arithmetic algorithm (AOA), Jaya Rao-3 algorithm. Among these, AOA demonstrated superior within manufacturing environment. As compared experimental values AOA, show improvement 6.33% 0.35% respectively.
Язык: Английский
Процитировано
1Archives of Computational Methods in Engineering, Год журнала: 2023, Номер 31(3), С. 1659 - 1700
Опубликована: Ноя. 30, 2023
Язык: Английский
Процитировано
13Wiley Interdisciplinary Reviews Data Mining and Knowledge Discovery, Год журнала: 2024, Номер 14(6)
Опубликована: Авг. 18, 2024
Abstract This paper reviews the integration of Q‐learning with meta‐heuristic algorithms (QLMA) over last 20 years, highlighting its success in solving complex optimization problems. We focus on key aspects QLMA, including parameter adaptation, operator selection, and balancing global exploration local exploitation. QLMA has become a leading solution industries like energy, power systems, engineering, addressing range mathematical challenges. Looking forward, we suggest further integration, transfer learning strategies, techniques to reduce state space. article is categorized under: Technologies > Computational Intelligence Artificial
Язык: Английский
Процитировано
5