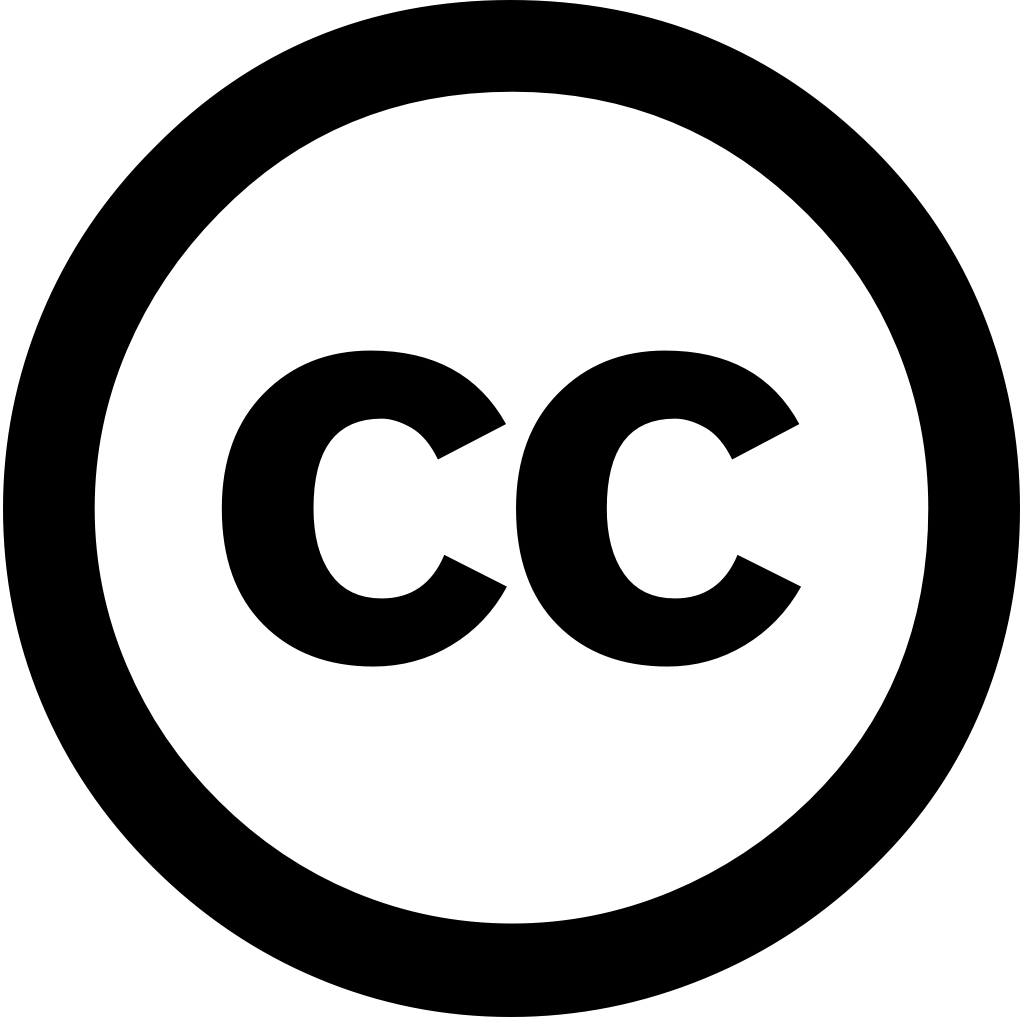
Biosensors, Год журнала: 2025, Номер 15(4), С. 255 - 255
Опубликована: Апрель 16, 2025
Diabetes is a growing global health concern, affecting millions and leading to severe complications if not properly managed. The primary challenge in diabetes management maintaining blood glucose levels (BGLs) within safe range prevent such as renal failure, cardiovascular disease, neuropathy. Traditional methods, finger-prick testing, often result low patient adherence due discomfort, invasiveness, inconvenience. Consequently, there an increasing need for non-invasive techniques that provide accurate BGL measurements. Photoplethysmography (PPG), photosensitive method detects volume variations, has shown promise monitoring. Deep neural networks (DNNs) applied PPG signals can predict BGLs with high accuracy. However, training DNN models requires large diverse datasets, which are typically distributed across multiple healthcare institutions. Privacy concerns regulatory restrictions further limit data sharing, making conventional centralized machine learning (ML) approaches less effective. To address these challenges, this study proposes federated (FL)-based solution enables organizations collaboratively train model without sharing raw data, thereby enhancing performance while ensuring privacy security. In the preprocessing stage, continuous wavelet transform (CWT) smooth remove baseline drift. Adaptive cycle-based segmentation (ACBS) then used signal segmentation, followed by particle swarm optimization (PSO) feature selection, optimizing classification proposed system was evaluated on including VitalDB MUST, under various conditions collected during surgery anesthesia. achieved root mean square error (RMSE) of 19.1 mg/dL, demonstrating superior predictive Clarke grid analysis (CEGA) confirmed model’s clinical reliability, 99.31% predictions falling clinically acceptable limits. FL-based approach outperformed deep models, it promising non-invasive, privacy-preserving
Язык: Английский