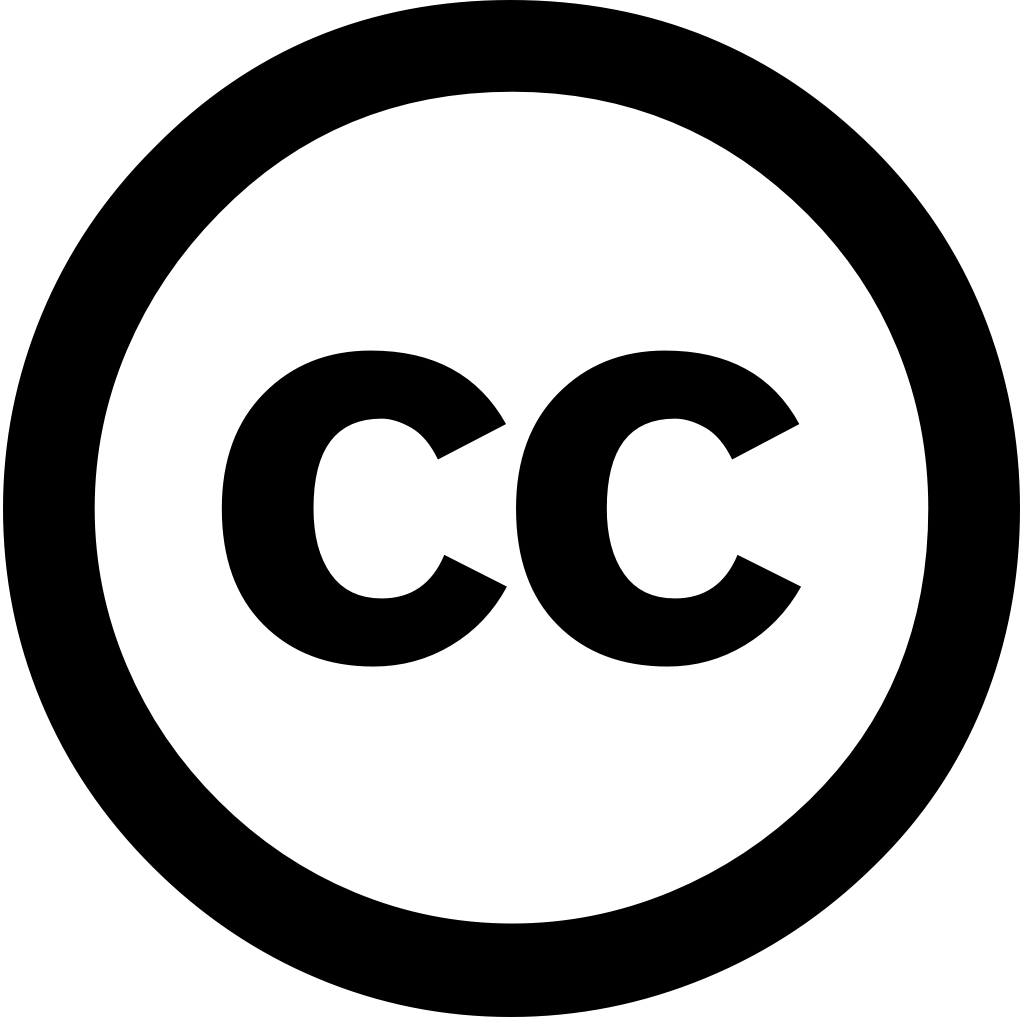
Agronomy, Год журнала: 2024, Номер 14(10), С. 2389 - 2389
Опубликована: Окт. 16, 2024
Breeding high-photosynthetic-efficiency wheat varieties is a crucial link in safeguarding national food security. Traditional identification methods necessitate laborious on-site observation and measurement, consuming time effort. Leveraging unmanned aerial vehicle (UAV) remote sensing technology to forecast photosynthetic indices opens up the potential for swiftly discerning varieties. The objective of this research develop multi-stage predictive model encompassing nine indicators at field scale breeding. These include soil plant analyzer development (SPAD), leaf area index (LAI), net rate (Pn), transpiration (Tr), intercellular CO2 concentration (Ci), stomatal conductance (Gsw), photochemical quantum efficiency (PhiPS2), PSII reaction center excitation energy capture (Fv’/Fm’), quenching coefficient (qP). ultimate goal differentiate through model-based predictions. This gathered red, green, blue spectrum (RGB) multispectral (MS) images eleven stages jointing, heading, flowering, filling. Vegetation (VIs) texture features (TFs) were extracted as input variables. Three machine learning regression models (Support Vector Machine Regression (SVR), Random Forest (RF), BP Neural Network (BPNN)) employed construct across multiple growth stages. Furthermore, conducted principal component analysis (PCA) membership function on predicted values optimal each indicator, established comprehensive evaluation high efficiency, cluster screen test materials. categorized into three groups, with SH06144 Yannong 188 demonstrating higher efficiency. moderately efficient group comprises Liangxing 19, SH05604, SH06085, Chaomai 777, SH05292, Jimai 22, Guigu 820, totaling seven Xinmai 916 Jinong 114 fall category lower aligning closely results clustering based actual measurements. findings suggest that employing UAV-based multi-source identify feasible. study provide theoretical basis winter phenotypic monitoring breeding using sensing, offering valuable insights advancement smart practices
Язык: Английский