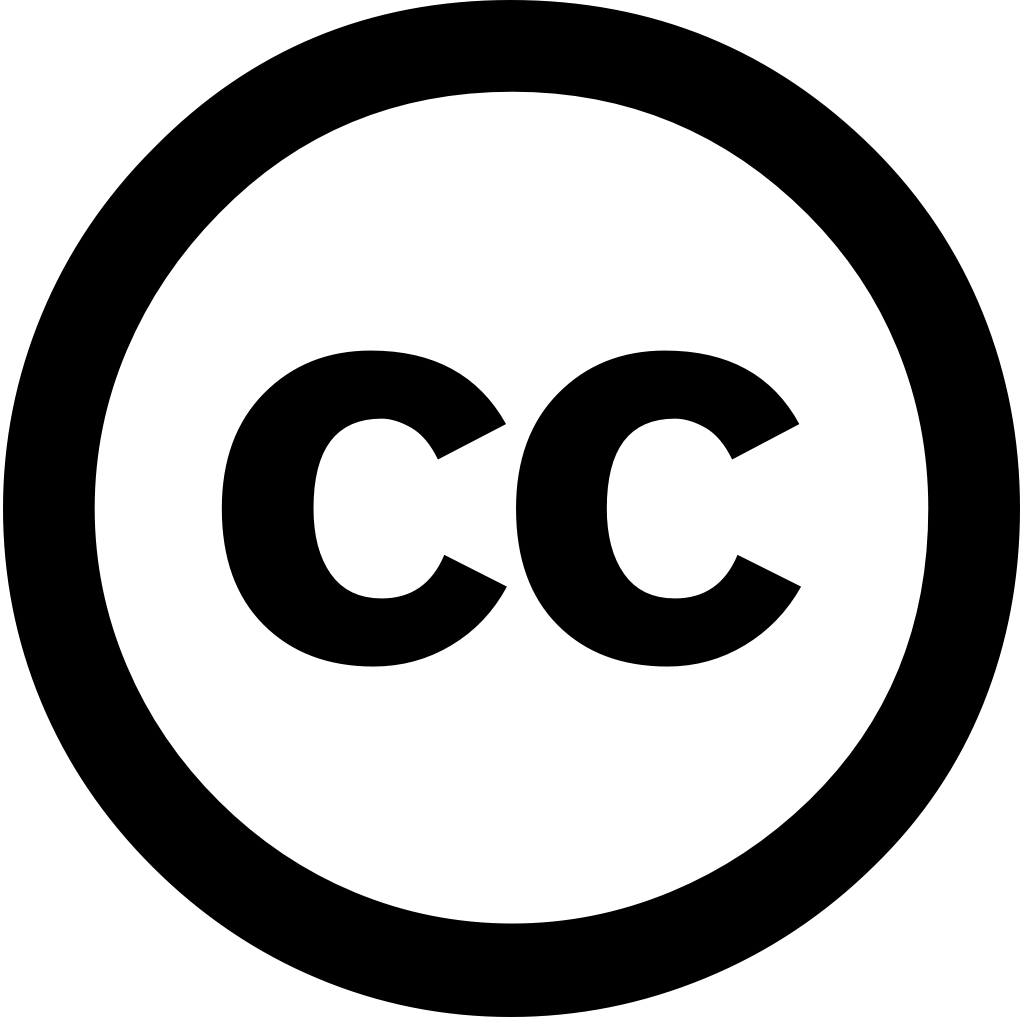
Agricultural Water Management, Год журнала: 2024, Номер 307, С. 109268 - 109268
Опубликована: Дек. 24, 2024
Язык: Английский
Agricultural Water Management, Год журнала: 2024, Номер 307, С. 109268 - 109268
Опубликована: Дек. 24, 2024
Язык: Английский
Journal of Hydrology, Год журнала: 2024, Номер 634, С. 131117 - 131117
Опубликована: Март 24, 2024
Global climate change has led to an increase in the frequency and scale of extreme weather events worldwide, there is urgent need develop better-performing hydrological models improve accuracy streamflow simulations facilitate water resource planning management. The Soil Water Assessment Tool (SWAT) a notable physical foundation widely used research. However, it uses simplified vegetation growth model, introducing uncertainty into simulation results. This study focused on improving model based remotely sensed phenological leaf area index (LAI) data. Phenological data were define dormancy, LAI replaced corresponding simulated by original model. approach improved describing dynamics. Then, enhanced SWAT was coupled with bidirectional long short-term memory (BiLSTM) validate processes upstream Hei River. During validation, performance simulating (R2 = 0.835, NSE 0.819) better than that 0.821, 0.805). In terms evapotranspiration, demonstrated even greater advantages. verification period, compared those R2 values for daily-scale increased from 0.196 −0.269 0.777 0.732, respectively. monthly-scale 0.782 0.678 0.906 0.851, Simultaneously, levels two coupling approaches prediction compared, i.e., direct BiLSTM (SWAT-BiLSTM) (enhanced SWAT-BiLSTM). results showed SWAT-BiLSTM always performed during entire especially which could more accurately predict peak changes. deep learning accuracy.
Язык: Английский
Процитировано
18Applied Soft Computing, Год журнала: 2024, Номер unknown, С. 112352 - 112352
Опубликована: Окт. 1, 2024
Язык: Английский
Процитировано
8Agricultural Water Management, Год журнала: 2024, Номер 296, С. 108798 - 108798
Опубликована: Март 29, 2024
Deficit drip irrigation is an advanced agricultural technology widely promoted and applied around the world to maintain/increase yield quality while simultaneously minimizing water consumption. However, there still a lack of comprehensive research on effects deficit at different stages apple yield, productivity, quality. The aim was investigate treatments (DDITs) quality, productivity (WP), optimize strategy for apples in Loess Plateau China. two-year field experiment conducted with 17 treatments, including control treatment (CK, full (FI)) four DDITs (D15%, D30%, D45%, D60%) during bud burst leafing stage (I), flowering fruit set (II), expansion (III), maturation (IV). optimal management determined using fuzzy Borda combined model, which based single evaluation models TOPSIS method, principal component analysis (PCA), grey correlation (GCA), membership function (MFA). IV decreased midday leaf potential (Ψs) by 2.57–20.00% compared CK, difference Ψs between IV-D30%, IV-D45%, IV-D60%, CK were significant two years (P<0.05). I, II, improved weight (FW), shape index (FSI), firmness (FN), but did not significantly affect content (FWC). III enhanced total soluble solids (TSS), sugars (SS), vitamin C (Vc) 2021 2022, notable decrease titratable acidity (TA) observed. exhibited highest benefit apple, followed I had lowest values. D15%, D15% III, identified as improve WP. Our findings can guide development appropriate strategies cultivation China, promoting efficient sustainable production.
Язык: Английский
Процитировано
6The Science of The Total Environment, Год журнала: 2024, Номер 947, С. 174480 - 174480
Опубликована: Июль 5, 2024
Язык: Английский
Процитировано
4Computers, Год журнала: 2024, Номер 13(8), С. 191 - 191
Опубликована: Авг. 7, 2024
In recent advancements in agricultural technology, quantum mechanics and deep learning integration have shown promising potential to revolutionize rice yield forecasting methods. This research introduces a novel Hybrid Quantum Deep Learning model that leverages the intricate processing capabilities of computing combined with robust pattern recognition prowess algorithms such as Extreme Gradient Boosting (XGBoost) Bidirectional Long Short-Term Memory (Bi-LSTM). Bi-LSTM networks are used for temporal feature extraction circuits processing. leverage superposition entanglement enhance data representation by capturing interactions. These enriched features extracted fed into an XGBoost regressor. By synthesizing classical machine techniques, our aims improve prediction accuracy significantly. Based on measurements mean square error (MSE), coefficient determination (R2), average (MAE), results 1.191621 × 10−5, 0.999929482, 0.001392724, respectively. value is so close perfect it helps make essential decisions global planning management.
Язык: Английский
Процитировано
4International Journal of Computational Intelligence Systems, Год журнала: 2025, Номер 18(1)
Опубликована: Фев. 10, 2025
Язык: Английский
Процитировано
0Scientific Reports, Год журнала: 2025, Номер 15(1)
Опубликована: Фев. 24, 2025
Abstract Oral carcinoma (OC) is a toxic illness among the most general malignant cancers globally, and it has developed gradually significant public health concern in emerging low-to-middle-income states. Late diagnosis, high incidence, inadequate treatment strategies remain substantial challenges. Analysis at an initial phase for good treatment, prediction, existence. Despite current growth perception of molecular devices, late analysis methods near precision medicine OC patients challenge. A machine learning (ML) model was employed to improve early detection medicine, aiming reduce cancer-specific mortality disease progression. Recent advancements this approach have significantly enhanced extraction diagnosis critical information from medical images. This paper presents Deep Structured Learning with Vision Intelligence Carcinoma Lesion Segmentation Classification (DSLVI-OCLSC) imaging. Using imaging, DSLVI-OCLSC aims enhance OC’s classification recognition outcomes. To accomplish this, utilizes wiener filtering (WF) as pre-processing technique eliminate noise. In addition, ShuffleNetV2 method used group higher-level deep features input image. The convolutional bidirectional long short-term memory network multi-head attention mechanism (MA-CNN‐BiLSTM) utilized oral identification. Moreover, Unet3 + segment abnormal regions classified Finally, sine cosine algorithm (SCA) hyperparameter-tune DL model. wide range simulations implemented ensure performance under images dataset. experimental portrayed superior accuracy value 98.47% over recent approaches.
Язык: Английский
Процитировано
0Agriculture, Год журнала: 2025, Номер 15(5), С. 544 - 544
Опубликована: Март 3, 2025
This study addresses the challenge of estimating reference crop evapotranspiration (ETO) in Xizang Plateau irrigation districts with limited meteorological data by proposing a coupled LASSO-BP model that integrates LASSO regression BP neural network. The was applied to three districts: Moda (MD), Jiangbei (JB), and Manla (ML). Using ETO values calculated FAO-56 Penman–Monteith (FAO-56PM) as benchmark, performance applicability were assessed. Short-term predictions for also conducted using mean-generating function optimal subset algorithm. results revealed significant multicollinearity among six factors (maximum temperature, minimum average relative humidity, sunshine duration, wind speed), identified through tolerance, variance inflation factor (VIF), eigenvalue analysis. effectively captured interannual variation ETO, accurately identifying peaks troughs, trends closely aligned FAO-56PM model. demonstrated strong across all districts, evaluation metrics showing MAE, RMSE, NSE, R2 ranging from 4.26 9.48 mm·a−1, 5.91 11.78 0.92 0.96, 0.82 0.94, respectively. Prediction indicated statistically insignificant declining trend annual over period. Overall, is reliable accurate tool data.
Язык: Английский
Процитировано
0Irrigation and Drainage, Год журнала: 2025, Номер unknown
Опубликована: Март 10, 2025
ABSTRACT Agricultural productivity is crucial for population growth, but 83% of India's water usage due to unplanned consumption. To address this, smart agriculture, which uses Internet Things (IoT) technologies efficient management, essential. Smart irrigation control addressing waste and promoting agricultural growth. make agriculture smart, automation IoT emerged with the deep learning algorithms used in research. Despite advancements, existing research models have drawbacks, including limited accuracy predicting moisture content inefficient resource utilization. drawbacks techniques, a novel approach named distributed bidirectional long short‐term memory optimized Jubatus classifier algorithm (JCA‐DiBiLSTM) automatic proposed. The proposed method integrates BiLSTM networks computing architecture, enhancing predictive by capturing complex temporal dependencies data. Additionally, (JCA) optimizes model parameters, improving overall prediction performance. By leveraging JCA‐DiBiLSTM, significant advantages are observed land. demonstrates enhanced accuracy, outperforming traditional methods mitigating over‐ or under‐irrigation risks. Furthermore, JCA‐DiBiLSTM ensures an specificity 94% minimizes mean square error (MSE) 5.5 while maximizing sensitivity 94%.
Язык: Английский
Процитировано
0Journal of Hydrology, Год журнала: 2024, Номер 641, С. 131787 - 131787
Опубликована: Авг. 13, 2024
Язык: Английский
Процитировано
2