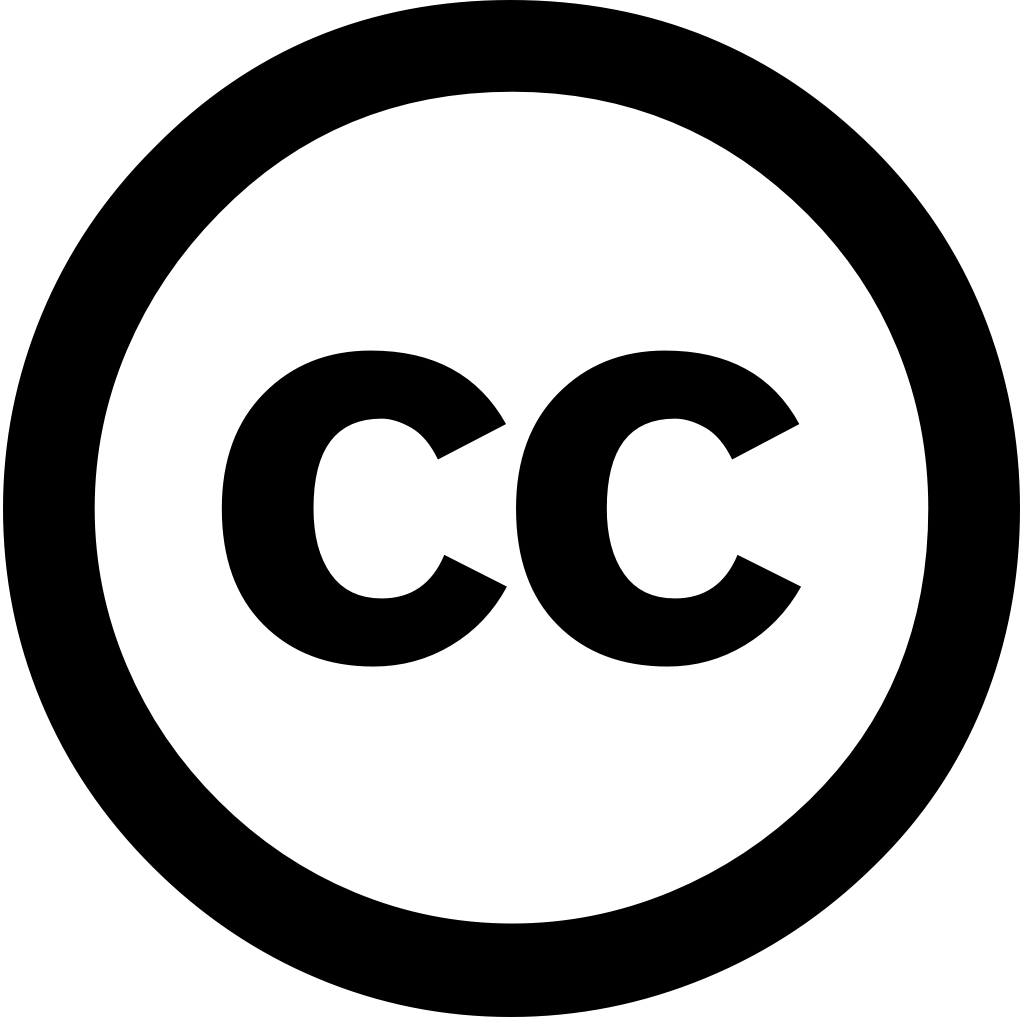
Journal of Medical Internet Research, Год журнала: 2024, Номер unknown
Опубликована: Ноя. 26, 2024
Язык: Английский
Journal of Medical Internet Research, Год журнала: 2024, Номер unknown
Опубликована: Ноя. 26, 2024
Язык: Английский
Diagnostics, Год журнала: 2025, Номер 15(4), С. 434 - 434
Опубликована: Фев. 11, 2025
Artificial intelligence (AI) has emerged as a transformative force in psychiatry, improving diagnostic precision, treatment personalization, and early intervention through advanced data analysis techniques. This review explores recent advancements AI applications within focusing on EEG ECG analysis, speech natural language processing (NLP), blood biomarker integration, social media utilization. EEG-based models have significantly enhanced the detection of disorders such depression schizophrenia spectral connectivity analyses. ECG-based approaches provided insights into emotional regulation stress-related conditions using heart rate variability. Speech frameworks, leveraging large (LLMs), improved cognitive impairments psychiatric symptoms nuanced linguistic feature extraction. Meanwhile, analyses deepened our understanding molecular underpinnings mental health disorders, analytics demonstrated potential for real-time surveillance. Despite these advancements, challenges heterogeneity, interpretability, ethical considerations remain barriers to widespread clinical adoption. Future research must prioritize development explainable models, regulatory compliance, integration diverse datasets maximize impact care.
Язык: Английский
Процитировано
3Frontiers in Public Health, Год журнала: 2025, Номер 13
Опубликована: Янв. 29, 2025
Background Web-based medical services have significantly improved access to healthcare by enabling remote consultations, streamlining scheduling, and improving information. However, providing personalized physician recommendations remains a challenge, often relying on manual triage schedulers, which can be limited scalability availability. Objective This study aimed develop validate Retrieval-Augmented Generation-Based Physician Recommendation (RAGPR) model for better performance. Methods utilizes comprehensive dataset consisting of 646,383 consultation records from the Internet Hospital First Affiliated Xiamen University. The research primarily evaluates performance various embedding models, including FastText, SBERT, OpenAI, purposes clustering classifying condition labels. Additionally, assesses effectiveness large language models (LLMs) comparing Mistral, GPT-4o-mini, GPT-4o. Furthermore, includes participation three staff members who contributed evaluation efficiency RAGPR through questionnaires. Results results highlight different levels in text tasks. FastText has an F 1 -score 46%, while SBERT OpenAI outperform it, achieving -scores 95 96%, respectively. analysis highlights LLMs, with GPT-4o highest 95%, followed Mistral GPT-4o-mini 94 92%, In addition, ratings are as follows: 4.56, 4.45 4.67. Among these, identified optimal choices due their balanced performance, cost effectiveness, ease implementation. Conclusion improve accuracy personalization web-based services, scalable solution patient-physician matching.
Язык: Английский
Процитировано
0npj Digital Medicine, Год журнала: 2025, Номер 8(1)
Опубликована: Март 22, 2025
Abstract While generative artificial intelligence (AI) has shown potential in medical diagnostics, comprehensive evaluation of its diagnostic performance and comparison with physicians not been extensively explored. We conducted a systematic review meta-analysis studies validating AI models for tasks published between June 2018 2024. Analysis 83 revealed an overall accuracy 52.1%. No significant difference was found ( p = 0.10) or non-expert 0.93). However, performed significantly worse than expert 0.007). Several demonstrated slightly higher compared to non-experts, although the differences were significant. Generative demonstrates promising capabilities varying by model. Although it yet achieved expert-level reliability, these findings suggest enhancing healthcare delivery education when implemented appropriate understanding limitations.
Язык: Английский
Процитировано
0Asian Journal of Psychiatry, Год журнала: 2025, Номер unknown, С. 104499 - 104499
Опубликована: Апрель 1, 2025
Язык: Английский
Процитировано
0medRxiv (Cold Spring Harbor Laboratory), Год журнала: 2024, Номер unknown
Опубликована: Дек. 2, 2024
Diagnosing rare genetic disorders relies on precise phenotypic and genotypic analysis, with the Human Phenotype Ontology (HPO) providing a standardized language for capturing clinical phenotypes. Traditional HPO tools, such as Doc2HPO ClinPhen, employ concept recognition to automate phenotype extraction but struggle incomplete assignment, often requiring intensive manual review. While large models (LLMs) hold promise more context-driven extraction, they are prone errors "hallucinations," making them less reliable without further refinement. We present RAG-HPO, Python-based tool that leverages Retrieval-Augmented Generation (RAG) elevate LLM accuracy in term bypassing limitations of baseline while avoiding time resource process fine-tuning. RAG-HPO integrates dynamic vector database, allowing real-time retrieval contextual matching.
Язык: Английский
Процитировано
1medRxiv (Cold Spring Harbor Laboratory), Год журнала: 2024, Номер unknown
Опубликована: Дек. 16, 2024
Abstract Background The rapid development of large language model chatbots, such as ChatGPT, has created new possibilities for healthcare support. This study investigates the feasibility integrating self-monitoring hearing (via a mobile app) with ChatGPT’s decision-making capabilities to assess whether specialist consultation is required. In particular, evaluated how accuracy make recommendation changed over periods up 12 months. Methods ChatGPT-4.0 was tested on dataset 1,000 simulated cases, each containing monthly threshold measurements Its recommendations were compared opinions 5 experts using percent agreement and Cohen’s Kappa. A multiple-response strategy, selecting most frequent from trials, also analyzed. Results ChatGPT aligned strongly experts’ judgments, scores ranging 0.80 0.84. Accuracy improved 0.87 when multiple-query strategy employed. those cases where all unanimously agreed, achieved near-perfect score 0.99. It adapted its criteria extended observation periods, seemingly accounting potential random fluctuations in thresholds. Conclusions significant decision-support tool monitoring hearing, able match expert adapting effectively time-series data. Existing self-testing apps lack tracking evaluating changes time; could fill this gap. While not without limitations, offers promising complement self-monitoring. can enhance processes potentially encourage patients seek clinical expertise needed. Graphical abstract
Язык: Английский
Процитировано
0Journal of Medical Internet Research, Год журнала: 2024, Номер unknown
Опубликована: Ноя. 26, 2024
Язык: Английский
Процитировано
0