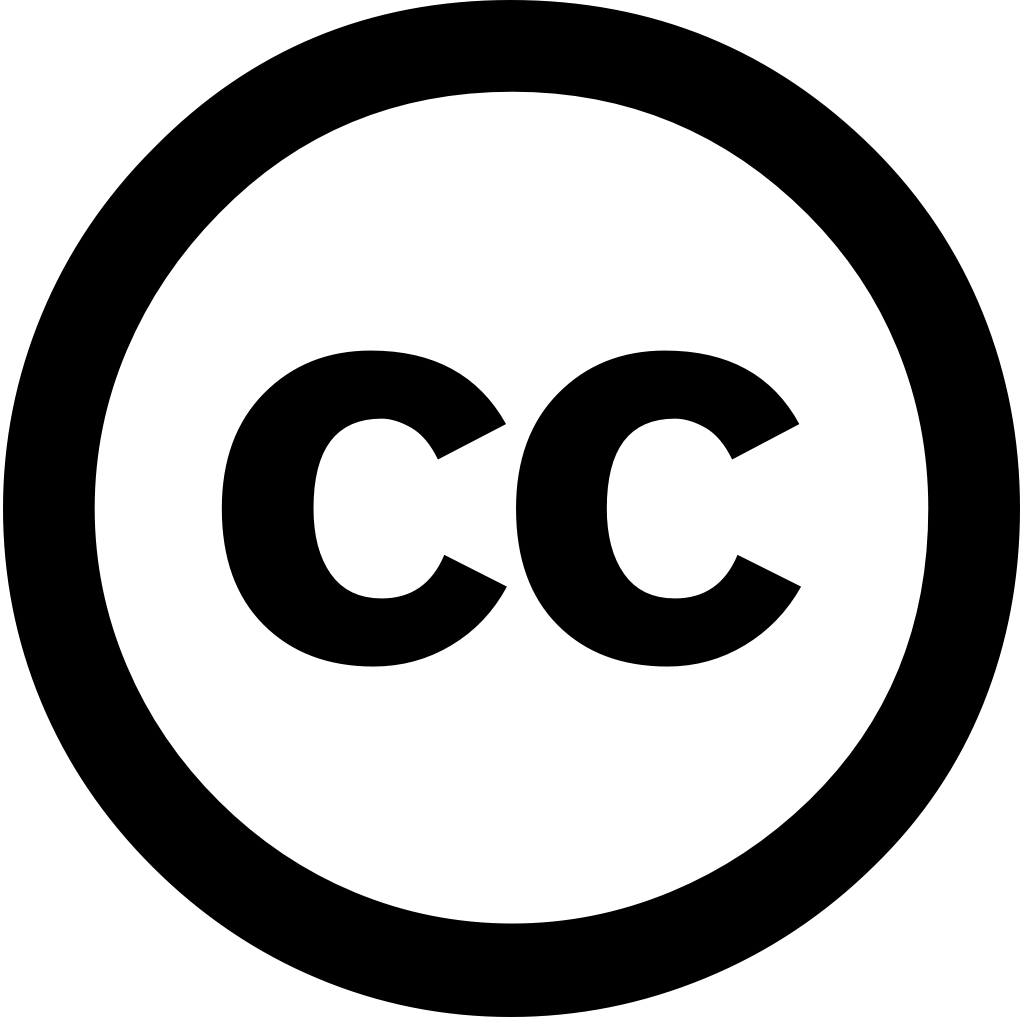
JMIR Medical Informatics, Год журнала: 2024, Номер unknown
Опубликована: Ноя. 17, 2024
Язык: Английский
JMIR Medical Informatics, Год журнала: 2024, Номер unknown
Опубликована: Ноя. 17, 2024
Язык: Английский
Frontiers in Medicine, Год журнала: 2025, Номер 12
Опубликована: Апрель 8, 2025
Modern healthcare depends fundamentally on clinical biochemistry for disease diagnosis and therapeutic guidance. The discipline encounters operational constraints, including sampling inefficiencies, precision limitations, expansion difficulties. Recent advancements in established technologies, such as mass spectrometry the development of high-throughput screening point-of-care are revolutionizing industry. biosensor technology wearable monitors facilitate continuous health tracking, Artificial Intelligence (AI)/machine learning (ML) applications enhance analytical capabilities, generating predictive insights individualized treatment protocols. However, concerns regarding algorithmic bias, data privacy, lack transparency decision-making (“black box” models), over-reliance automated systems pose significant challenges that must be addressed responsible AI integration. limitations remain—substantial implementation expenses, system incompatibility issues, information security vulnerabilities intersect with ethical considerations fairness protected information. Addressing these demands coordinated efforts between clinicians, scientists, technical specialists. This review discusses current biochemistry, explicitly addressing reference intervals barriers to implementing innovative biomarkers medical settings. discussion evaluates how advanced technologies multidisciplinary collaboration can overcome constraints while identifying research priorities diagnostic accessibility better delivery.
Язык: Английский
Процитировано
0Current Opinion in Ophthalmology, Год журнала: 2024, Номер unknown
Опубликована: Окт. 24, 2024
Purpose of review As the surge in large language models (LLMs) and generative artificial intelligence (AI) applications ophthalmology continue to expand, this seeks update physicians current progress, catalyze further work harness its capabilities enhance healthcare delivery ophthalmology. Recent findings Generative AI have shown promising performance Ophthalmology. Beyond native LLMs question-answering based tasks, there has been increasing employing novel LLM techniques exploring wider use case applications. Summary In review, we first look at existing specific Ophthalmology, followed by an overview commonly used techniques. We finally focus on emerging trends space with angle from
Язык: Английский
Процитировано
1College & Undergraduate Libraries, Год журнала: 2024, Номер unknown, С. 1 - 25
Опубликована: Ноя. 11, 2024
This study assesses the artificial intelligence (AI) literacy and perceptions among Master of Library Science (MLS) students in India. It aims to identify most relevant AI knowledge areas for library science, examine students' attitudes toward AI's potential impact on services, explores importance professional practice. A quantitative survey administered 118 final-year MLS via WhatsApp groups personal networks collected data levels, perceptions, attitudes. The findings reveal that natural language processing (NLP) is considered transformative technology, with significant interest other like recommender systems mining. Students express optimism about enhance services but raise concerns ethical implications job displacement. highlights need increased training integration into LIS programs, emphasizing its future career competitiveness. results provide valuable insights how can be fostered align evolving user needs.
Язык: Английский
Процитировано
0Опубликована: Ноя. 17, 2024
Язык: Английский
Процитировано
0JMIR Medical Informatics, Год журнала: 2024, Номер unknown
Опубликована: Ноя. 17, 2024
Язык: Английский
Процитировано
0