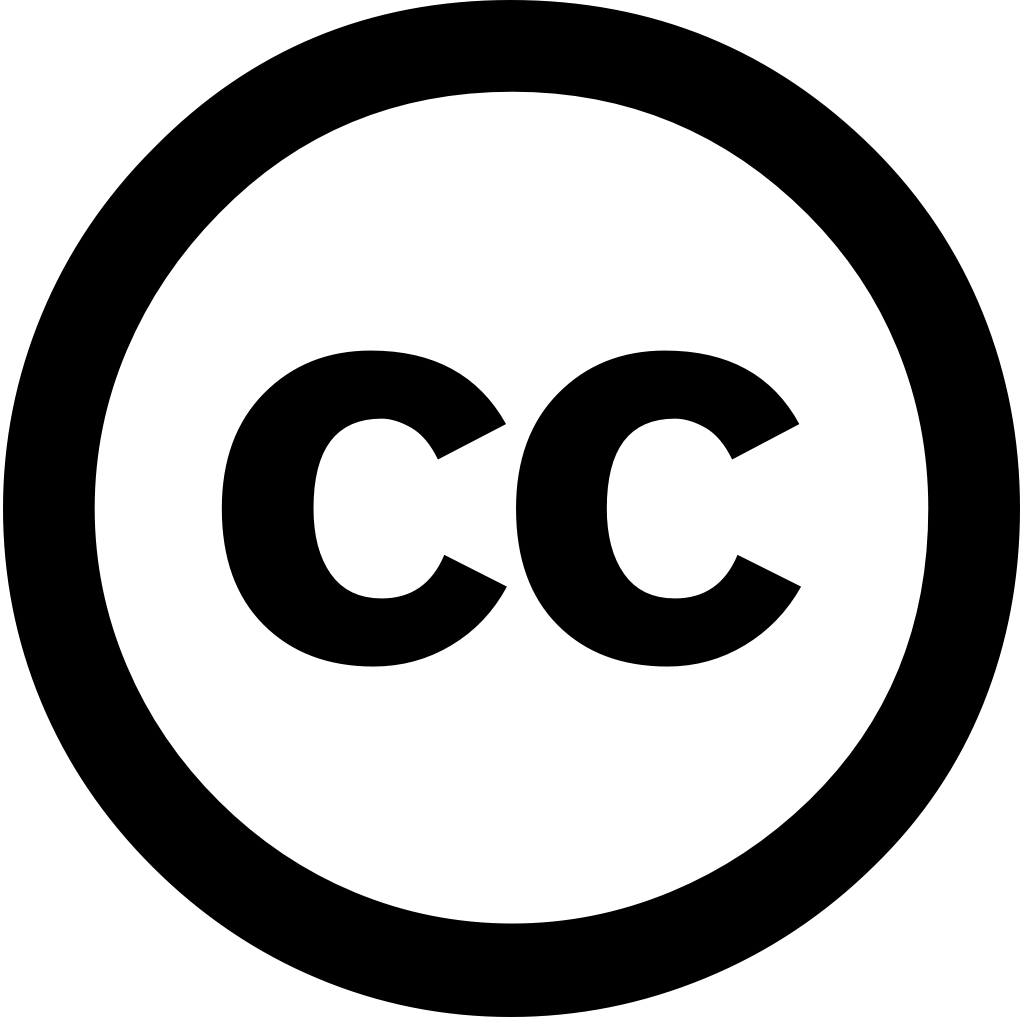
Intelligent Systems with Applications, Год журнала: 2024, Номер 25, С. 200463 - 200463
Опубликована: Дек. 7, 2024
Язык: Английский
Intelligent Systems with Applications, Год журнала: 2024, Номер 25, С. 200463 - 200463
Опубликована: Дек. 7, 2024
Язык: Английский
Frontiers in Neuroscience, Год журнала: 2025, Номер 18
Опубликована: Янв. 7, 2025
To assist in the rapid clinical identification of brain tumor types while achieving segmentation detection, this study investigates feasibility applying deep learning YOLOv5s algorithm model to magnetic resonance images and optimizes upgrades it on basis. The research institute utilized two public datasets meningioma glioma imaging from Kaggle. Dataset 1 contains a total 3,223 images, 2 216 images. From 1, we randomly selected 3,000 used Labelimg tool annotate cancerous regions within These were then divided into training validation sets 7:3 ratio. remaining 223 along with 2, ultimately as internal test set external set, respectively, evaluate model's effect. A series optimizations made original YOLOv5 algorithm, introducing Atrous Spatial Pyramid Pooling (ASPP), Convolutional Block Attention Module (CBAM), Coordinate (CA) for structural improvement, resulting several optimized versions, namely YOLOv5s-ASPP, YOLOv5s-CBAM, YOLOv5s-CA, YOLOv5s-ASPP-CBAM, YOLOv5s-ASPP-CA. input model, five models, YOLOv8s 100 rounds iterative training. best weight file evaluation index six trained models was final set. After training, seven can segment recognize Their precision rates are 92.5, 93.5, 91.2, 91.8, 89.6, 90.8, 93.1%, respectively. corresponding recall 84, 85.3, 85.4, 84.7, 87.3, 91.9%. among tested improved significantly enhanced image ability compared model. Compared YOLOv5s-ASPP which is helpful assisting diagnosis treatment planning.
Язык: Английский
Процитировано
1Scientific Reports, Год журнала: 2025, Номер 15(1)
Опубликована: Янв. 17, 2025
This study develops the you only look once segmentation (YOLOSeg), an end-to-end instance model, with applications to segment small particle defects embedded on a wafer die. YOLOSeg uses YOLOv5s as basis and extends UNet-like structure form head. can predict not bounding boxes of but also corresponding polygons. Furthermore, attempts obtain set better weights by combining several training tricks such freezing layers, switching mask loss, using auto-anchor introducing denoising diffusion probabilistic models (DDPM) image augmentation. The experiment results testing show that YOLOSeg's average precision (AP) intersection over union (IoU) are high 0.821 0.732 respectively. Even when sizes extremely small, performance is far superior current R-CNN, YOLACT, YUSEG, Ultralytics's YOLOv5s-segmentation. Additionally, preparing for time-saving because it needs neither collect large number defective samples, nor annotate pseudo defects, design hand-craft features.
Язык: Английский
Процитировано
0Frontiers in Veterinary Science, Год журнала: 2025, Номер 12
Опубликована: Фев. 6, 2025
The facial coloration of sheep is not only a critical characteristic for breed and individual identification but also serves as significant indicator assessing genetic diversity guiding selective breeding efforts. In this study, 201 Ujumqin were used research objects 1713 head image data collected. We delineated feature points related to the successfully developed color recognition model (YOLOv8-CBAM) utilizing YOLOv8 architecture in conjunction with CBAM attention mechanism. demonstrated impressive performance recognizing four categories, achieving an average precision (mAP) 97.7% F1 score 0.94. comparison YOLOv8n, YOLOv8l, YOLOv8m, YOLOv8s, YOLOv8x, YOLOv8-CBAM enhances accuracy by 0.5%, 1%, 0.7%, 1.6%, respectively. Furthermore, when compared YOLOv3, improvement while YOLOv5n YOLOv10n show increases 1.4% 2.4%, findings indicate that smaller exhibited superior task sheep. Overall, achieved high task, providing reliable technical support automated management systems.
Язык: Английский
Процитировано
0Materials Today Communications, Год журнала: 2025, Номер unknown, С. 112050 - 112050
Опубликована: Фев. 1, 2025
Язык: Английский
Процитировано
0Geoenergy Science and Engineering, Год журнала: 2025, Номер unknown, С. 213844 - 213844
Опубликована: Март 1, 2025
Язык: Английский
Процитировано
0Sensors, Год журнала: 2025, Номер 25(7), С. 2199 - 2199
Опубликована: Март 31, 2025
With global climate change and the deterioration of ecological environment, safety hydraulic engineering faces severe challenges, among which soil-dwelling termite damage has become an issue that cannot be ignored. Reservoirs embankments in China, primarily composed earth rocks, are often affected by termites, such as Odontotermes formosanus Macrotermes barneyi. Identifying is crucial for implementing monitoring, early warning, control strategies. This study developed improved YOLOv8 model, named MCD-YOLOv8, identifying traces activity, based on Monte Carlo random sampling algorithm a lightweight module. The attention (MCA) module was introduced backbone part to generate maps through pooling operations, addressing cross-scale issues improving recognition accuracy small targets. A module, known dimension-aware selective integration (DASI), added neck reduce computation time memory consumption, enhancing detection speed. model verified using dataset 2096 images from survey within Hubei Province 2024, along with captured drone. results showed outperformed four traditional or enhanced models terms precision mean average detecting damage, while also exhibiting fewer parameters, reduced redundancy boxes, Specifically, MCD-YOLOv8 achieved increases 6.4% 2.4%, respectively, compared simultaneously reducing number parameters 105,320. suitable intelligent identification complex environments, thereby monitoring activity providing strong technical support development technologies.
Язык: Английский
Процитировано
0International Journal of Architectural Heritage, Год журнала: 2025, Номер unknown, С. 1 - 24
Опубликована: Апрель 1, 2025
Язык: Английский
Процитировано
0Neural Computing and Applications, Год журнала: 2025, Номер unknown
Опубликована: Апрель 26, 2025
Язык: Английский
Процитировано
0Agriculture, Год журнала: 2024, Номер 14(9), С. 1632 - 1632
Опубликована: Сен. 18, 2024
The separation of walnut kernels and shells has long been regarded as a bottleneck, limiting processing efficiency, product quality, industry advancement. In response to the challenges improving accuracy inadequacy existing equipment for meeting demands, this paper proposes an innovative shell–kernel device based on machine vision technology. An experimental system was constructed, key parameters were optimized enhance its performance. comprises five main modules: material conveyance, image acquisition, control module, sorting frame. Differential technology is used convert group into stable particle flow, addressing issue missed selections due blockages. enhanced YOLOv8n algorithm improves small object detection interference resistance, enabling accurate identification kernels. Box–Behnken Design Artificial Neural Network prediction model determine optimal operating device. Experimental results showed that effective differential achieved when dual-stage conveyor operated at speeds 0.2 m/s 1 m/s. improved I-YOLOv8n reached 98.8%. Using neural network model, operational determined: air pressure 0.72 MPa, jetting component angle 10.16°, height 105.12 cm. Under these conditions, actual cleaning rate 93.56%, demonstrating outstanding Compared traditional methods, offers significant advantages in terms ease operation, providing new technological pathways support automation intelligent transformation industry. future, expected undergo further improvements meet broader market demand serve reference other agricultural products.
Язык: Английский
Процитировано
1Journal of Pipeline Science and Engineering, Год журнала: 2024, Номер unknown, С. 100246 - 100246
Опубликована: Дек. 1, 2024
Язык: Английский
Процитировано
1