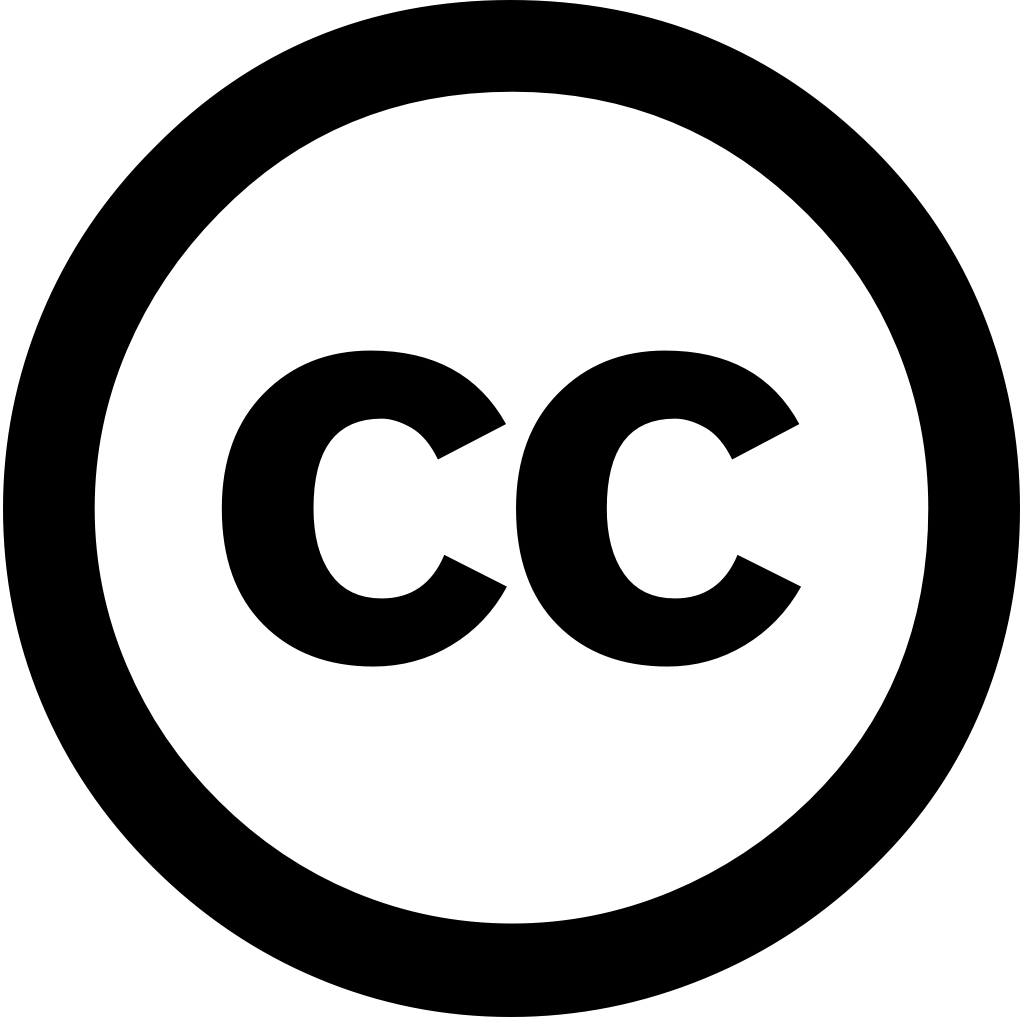
Heliyon, Год журнала: 2024, Номер 10(21), С. e39770 - e39770
Опубликована: Окт. 24, 2024
Язык: Английский
Heliyon, Год журнала: 2024, Номер 10(21), С. e39770 - e39770
Опубликована: Окт. 24, 2024
Язык: Английский
Energy Conversion and Management, Год журнала: 2025, Номер 326, С. 119484 - 119484
Опубликована: Янв. 13, 2025
Язык: Английский
Процитировано
2Expert Systems with Applications, Год журнала: 2024, Номер 246, С. 123086 - 123086
Опубликована: Янв. 13, 2024
Язык: Английский
Процитировано
14Discover Sustainability, Год журнала: 2024, Номер 5(1)
Опубликована: Ноя. 12, 2024
An accurate renewable energy output forecast is essential for efficiency and power system stability. Long Short-Term Memory(LSTM), Bidirectional LSTM(BiLSTM), Gated Recurrent Unit(GRU), Convolutional Neural Network-LSTM(CNN-LSTM) Deep Network (DNN) topologies are tested solar wind production forecasting in this study. ARIMA was compared to the models. This study offers a unique architecture Networks (DNNs) that specifically tailored forecasting, optimizing accuracy by advanced hyperparameter tuning incorporation of meteorological temporal variables. The optimized LSTM model outperformed others, with MAE (0.08765), MSE (0.00876), RMSE (0.09363), MAPE (3.8765), R2 (0.99234) values. GRU, CNN-LSTM, BiLSTM models predicted well. Meteorological time-based factors enhanced accuracy. addition sun data improved its prediction. results show deep neural network can predict energy, highlighting importance carefully selecting characteristics fine-tuning model. work improves estimates promote more reliable environmentally sustainable electricity system.
Язык: Английский
Процитировано
8Expert Systems with Applications, Год журнала: 2025, Номер unknown, С. 126624 - 126624
Опубликована: Янв. 1, 2025
Язык: Английский
Процитировано
1Applied Soft Computing, Год журнала: 2024, Номер 164, С. 112007 - 112007
Опубликована: Июль 17, 2024
Язык: Английский
Процитировано
5Applied Intelligence, Год журнала: 2025, Номер 55(4)
Опубликована: Янв. 13, 2025
Язык: Английский
Процитировано
0Earth Science Informatics, Год журнала: 2025, Номер 18(2)
Опубликована: Фев. 1, 2025
Язык: Английский
Процитировано
0Expert Systems with Applications, Год журнала: 2025, Номер unknown, С. 126706 - 126706
Опубликована: Фев. 1, 2025
Язык: Английский
Процитировано
0PLoS ONE, Год журнала: 2025, Номер 20(2), С. e0317148 - e0317148
Опубликована: Фев. 20, 2025
Cancer, the second-leading cause of mortality, kills 16% people worldwide. Unhealthy lifestyles, smoking, alcohol abuse, obesity, and a lack exercise have been linked to cancer incidence mortality. However, it is hard. Cancer lifestyle correlation analysis mortality prediction in next several years are used guide people's healthy lives target medical financial resources. Two key research areas this paper Data preprocessing sample expansion design Using experimental comparison, study chooses best cubic spline interpolation technology on original data from 32 entry points 420 converts annual into monthly solve problem insufficient prediction. Factor possible because sources indicate changing factors. TSA-LSTM Two-stage attention popular tool with advanced visualization functions, Tableau, simplifies paper's study. Tableau's testing findings cannot analyze predict time series data. LSTM utilized by optimization model. By commencing input feature attention, model technique guarantees that encoder converges subset sequence features during output features. As result, model's natural learning trend quality enhanced. The second step, performance maintains We can choose network improve forecasts based real-time performance. Validating source factor using Most cancers overlapping risk factors, excessive drinking, exercise, obesity breast, colorectal, colon cancer. A poor directly promotes lung, laryngeal, oral cancers, according visual tests. expected climb 18-21% between 2020 2025, 2021. Long-term projection accuracy 98.96 percent, smoking may be main causes.
Язык: Английский
Процитировано
0Scientific Reports, Год журнала: 2025, Номер 15(1)
Опубликована: Фев. 21, 2025
As a high-risk production unit, natural gas supply enterprises are increasingly recognizing the need to enhance safety management. Traditional process warning methods, which rely on fixed alarm values, often fail adequately account for dynamic changes in process. To address this issue, study utilizes deep learning techniques accuracy and reliability of load forecasting. By considering benefits feasibility integrating multiple models, VMD-CNN-LSTM-Self-Attention interval prediction method was innovatively proposed developed. Empirical research conducted using data from field station outgoing loads. The primary model constructed is loads, implements graded mechanism based 85%, 90%, 95% confidence intervals real-time observations. This approach represents novel strategy enhancing enterprise Experimental results demonstrate that outperforms traditional reducing MAE, MAPE, MESE, REMS by 1.13096 m3/h, 1.3504%, 7.6363 1.6743 respectively, while improving R2 0.04698. These findings expected offer valuable insights safe management industry provide new perspectives industry's digital intelligent transformation.
Язык: Английский
Процитировано
0