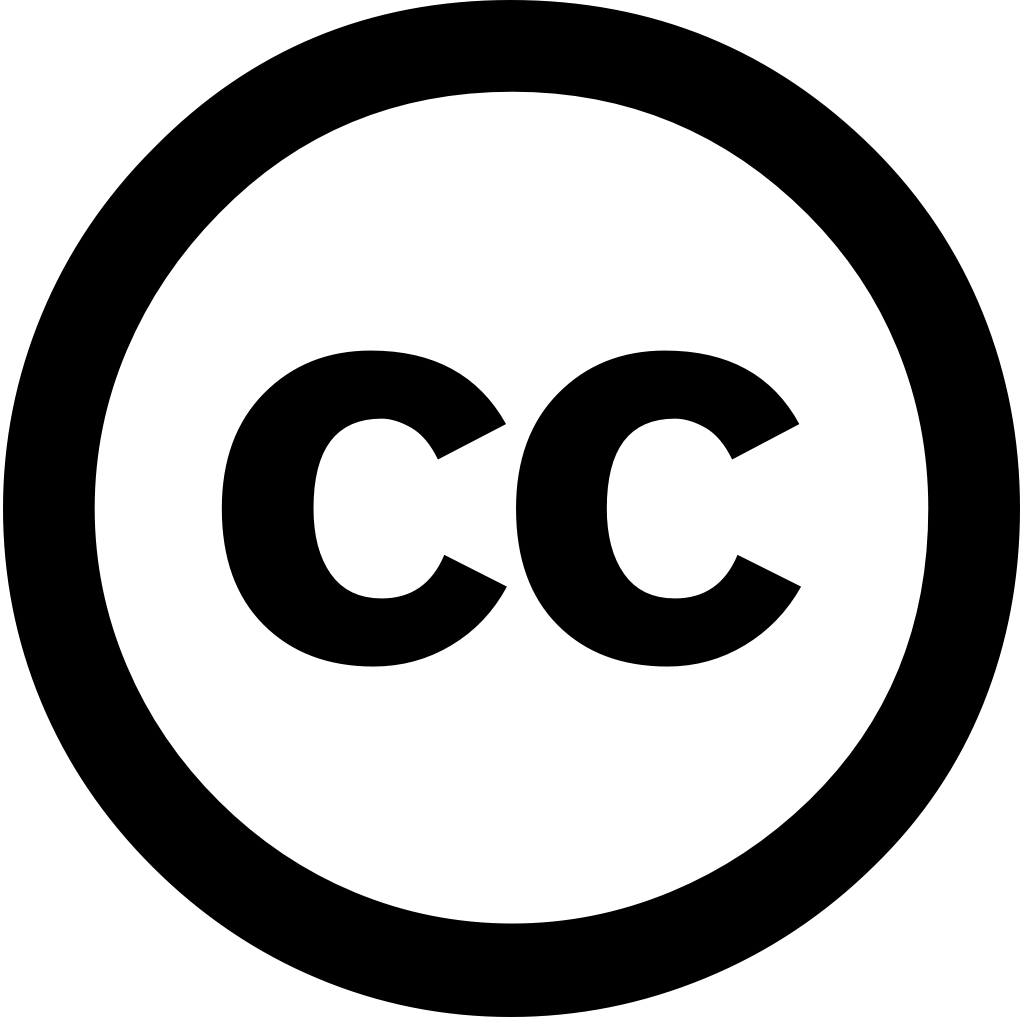
Sensors, Год журнала: 2025, Номер 25(7), С. 2039 - 2039
Опубликована: Март 25, 2025
The Vehicular Edge-Cloud Computing (VECC) paradigm has gained traction as a promising solution to mitigate the computational constraints through offloading resource-intensive tasks distributed edge and cloud networks. However, conventional computation mechanisms frequently induce network congestion service delays, stemming from uneven workload distribution across spatial Roadside Units (RSUs). Moreover, ensuring data security optimizing energy usage within this framework remain significant challenges. To end, study introduces deep reinforcement learning-enabled for multi-tier VECC First, dynamic load-balancing algorithm is developed optimize balance among RSUs, incorporating real-time analysis of heterogeneous parameters, including RSU load, channel capacity, proximity-based latency. Additionally, alleviate in static deployments, proposes deploying UAVs high-density zones, dynamically augmenting both storage processing resources. an Advanced Encryption Standard (AES)-based mechanism, secured with one-time encryption key generation, implemented fortify confidentiality during transmissions. Further, context-aware caching strategy preemptively store processed tasks, reducing redundant computations associated overheads. Subsequently, mixed-integer optimization model formulated that simultaneously minimizes consumption guarantees latency constraint. Given combinatorial complexity large-scale vehicular networks, equivalent learning form given. Then learning-based designed learn close-optimal solutions under conditions. Empirical evaluations demonstrate proposed significantly outperforms existing benchmark techniques terms savings. These results underscore framework's efficacy advancing sustainable, secure, scalable intelligent transportation systems.
Язык: Английский