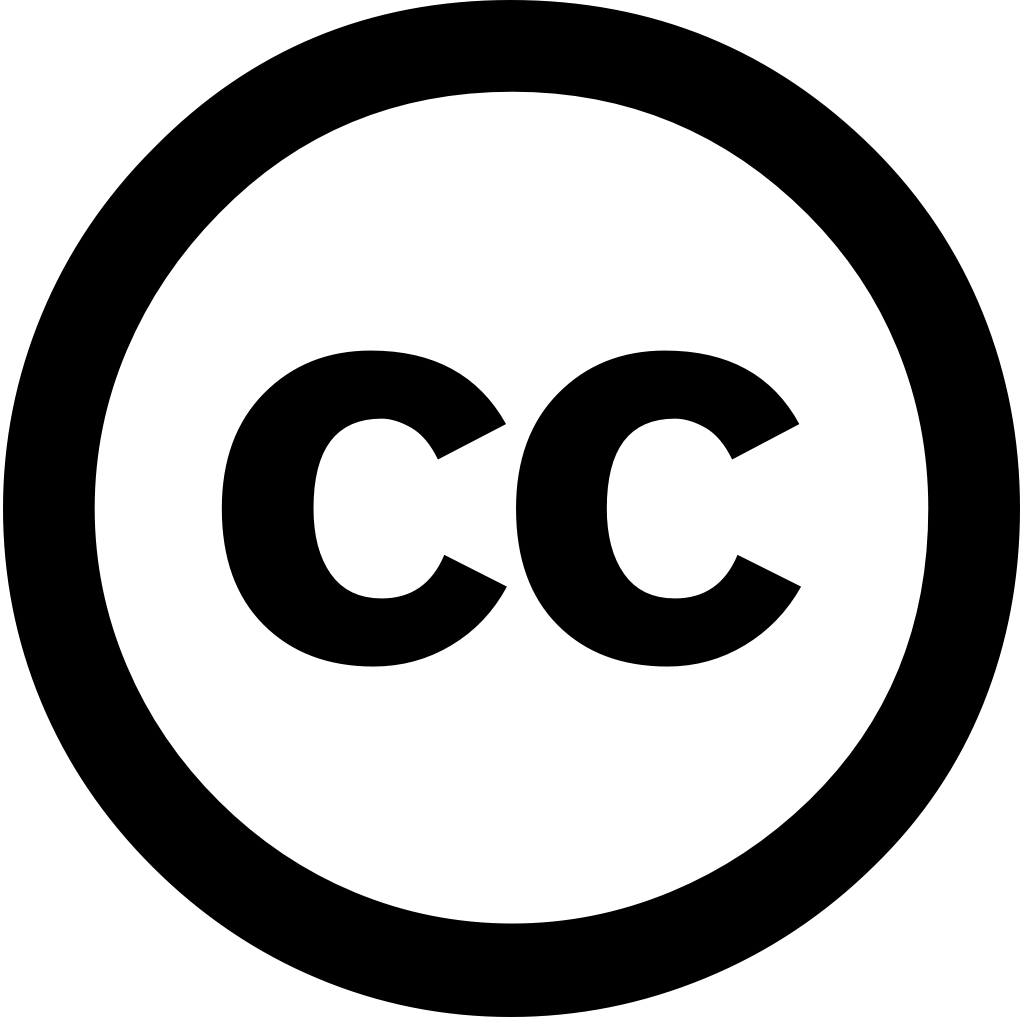
Automation, Год журнала: 2024, Номер 6(1), С. 2 - 2
Опубликована: Дек. 27, 2024
This study presents an automated control system for wastewater treatment, developed using machine learning (ML) models integrated into a Supervisory Control and Data Acquisition (SCADA) framework. The experimental setup focused on laboratory-scale Aerobic Granular Sludge (AGS) reactor, which utilized synthetic to model real-world conditions. models, specifically N-BEATS Temporal Fusion Transformers (TFTs), were trained predict Biological Oxygen Demand (BOD5) values historical data real-time influent contaminant concentrations obtained from online sensors. predictive approach proved essential due the absence of direct BOD5 measurements inconsistent relationship between Chemical (COD), with correlation approximately 0.4. Evaluation results showed that demonstrated highest accuracy, achieving Mean Absolute Error (MAE) 0.988 R2 0.901. integration SCADA enabled precise, adjustments reactor parameters, including sludge dose aeration intensity, leading significant improvements in granulation stability. effectively reduced standard deviation organic load fluctuations by 2.6 times, 0.024 0.006, thereby stabilizing process within AGS reactor. Residual analysis suggested minor bias, likely limited number features model, indicating potential through additional inputs. research demonstrates value learning-driven offering resilient solution dynamic environments. By facilitating proactive management, this supports scalability treatment technologies while enhancing efficiency operational sustainability.
Язык: Английский