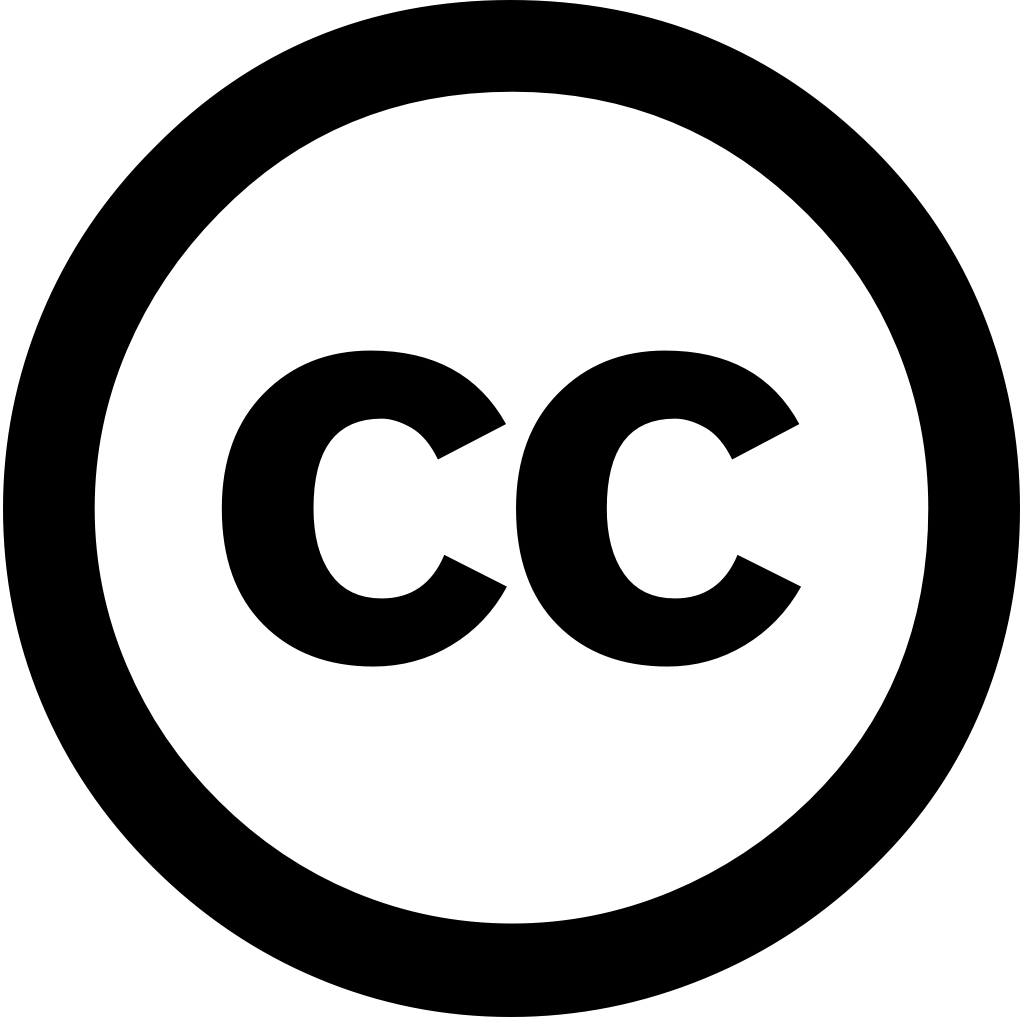
iScience, Год журнала: 2024, Номер 27(12), С. 111230 - 111230
Опубликована: Окт. 23, 2024
Язык: Английский
iScience, Год журнала: 2024, Номер 27(12), С. 111230 - 111230
Опубликована: Окт. 23, 2024
Язык: Английский
Engineering Applications of Artificial Intelligence, Год журнала: 2024, Номер 133, С. 108382 - 108382
Опубликована: Апрель 24, 2024
Язык: Английский
Процитировано
2Sensors, Год журнала: 2024, Номер 24(13), С. 4315 - 4315
Опубликована: Июль 2, 2024
Breathing is one of the body’s most basic functions and abnormal breathing can indicate underlying cardiopulmonary problems. Monitoring respiratory abnormalities help with early detection reduce risk diseases. In this study, a 77 GHz frequency-modulated continuous wave (FMCW) millimetre-wave (mmWave) radar was used to detect different types signals from human body in non-contact manner for monitoring (RM). To solve problem noise interference daily environment on recognition patterns, system utilised captured by radar. Firstly, we filtered out static using signal superposition method designed an elliptical filter obtain more accurate image waveforms between 0.1 Hz 0.5 Hz. Secondly, combined histogram oriented gradient (HOG) feature extraction algorithm, K-nearest neighbours (KNN), convolutional neural network (CNN), HOG support vector machine (G-SVM) were classify four modes, namely, normal breathing, slow deep quick meningitic breathing. The overall accuracy reached up 94.75%. Therefore, study effectively supports medical monitoring.
Язык: Английский
Процитировано
2Sensors, Год журнала: 2024, Номер 24(21), С. 6887 - 6887
Опубликована: Окт. 27, 2024
Respiratory disorders are commonly regarded as complex to diagnose due their multi-factorial nature, encompassing the interplay between hereditary variables, comorbidities, environmental exposures, and therapies, among other contributing factors. This study presents a Clinical Decision Support System (CDSS) for early detection of respiratory using one-dimensional convolutional neural network (1D-CNN) model. The ICBHI 2017 Breathing Sound Database, which contains samples different breathing sounds, was used in this research. During pre-processing, audio clips were resampled uniform rate, cycles segmented into individual instances lung sound. A One-Dimensional Convolutional Neural Network consisting layers, max pooling dropout fully connected designed classify processed four categories: normal, crackles, wheezes, combined crackles wheezes. To address class imbalance, Synthetic Minority Over-sampling Technique (SMOTE) applied training data. Hyperparameters optimized grid search with k−fold cross-validation. model achieved an overall accuracy 0.95, outperforming state-of-the-art methods. Particularly, normal categories attained highest F1-scores 0.97 respectively. model’s robustness further validated through 5−fold 10−fold cross-validation experiments. research highlighted essential aspect diagnosing sounds artificial intelligence utilized 1D-CNN accurately. proposed advancement technology shall enable medical care practitioners improved manner, leading better patient care.
Язык: Английский
Процитировано
2British Poultry Science, Год журнала: 2023, Номер 64(3), С. 343 - 356
Опубликована: Янв. 25, 2023
1. In a non-cage environment, goose eggs are buried in litter and feathers, leading to contamination discolouration. Such random distribution of poses great challenge the recognition location for intelligent picking by robot systems on farm.2. order assist environments, novel method was proposed which used three-channel convolutional neural network (T-CNN), composed improved AlexNet, combined with 'you only look once' (YOLOv5), egg contour curve creation support vector machine (SVM).3. Using this method, original images were put into YOLOv5 model target detection segmentation. parallel, median filter maximum interclass variance (OTSU) applied segmentation obtain main pixels each, Kirsch operator edge extraction curves fitting designing equation curves.4. further enrich differences between background, divided three colour components: R, G B, T-CNN feature extraction. Then classified stitching SVM, adding images.5. The results showed that about 95.65% pixel blocks segmented recognised correctly. About 3.81% incorrectly, centre mass offset 4.45 pixels.6. This study demonstrated accurate using environment. highlighted its application prospect as well possible use other species laying outside nest (floor eggs) example hens non cage systems.
Язык: Английский
Процитировано
5Frontiers in Digital Health, Год журнала: 2024, Номер 6
Опубликована: Дек. 23, 2024
The evolution of artificial intelligence (AI) has revolutionised numerous aspects our daily lives, with profound implications across various sectors, including healthcare. Although the concept AI in healthcare was introduced early 1970s, integration this technology is still phase. Despite barriers, current decade witnessing an increased utility into diverse specialities medical field to enhance precision medicine, predict diagnosis, therapeutic results, and prognosis; includes respiratory critical care, their allied specialties. algorithms are widely studied areas like mechanical ventilation, sleep lung ultrasound, pulmonary function diagnostics results found be promising. quality patient care safety can greatly enhanced if professionals fully understand importance AI, as they already incorporating clinical practice. Awareness essential during phase; hence, it desirable establish accepted standards presented a clear accessible language. This article aims describe existing prospective role areas.
Язык: Английский
Процитировано
1Journal of Intelligent & Fuzzy Systems, Год журнала: 2023, Номер 45(2), С. 1923 - 1937
Опубликована: Май 23, 2023
We studied China’s Common Prosperity process by assessing and comparing the level of in different regions China made some beneficial recommendations to government departments. The research data comes from Statistical Yearbook, which includes 31 provinces cities 2015 2020. According relevant research, eleven evaluation indicators were selected. combined GRA with TOPSIS method for scoring K-means clustering algorithm dividing GRA-TOPSIS results into three levels. Then, convolutional neural network model was used predict simulate common prosperity. Taking 2020 as an example, show: (1) From 2020, reached its highest point Due impact COVID-19 2019, scores are generally lower than previous four years. situation changed 2020; (2) In terms regional distribution, economic development Beijing, Shanghai, other eastern is relatively good, a higher degree that regions; (3) average prediction accuracy high our model. It can be close 100%, indicating has good effect. addition, we based on results, have references actively promoting
Язык: Английский
Процитировано
2Electronics, Год журнала: 2023, Номер 12(21), С. 4421 - 4421
Опубликована: Окт. 27, 2023
Audio signals play a crucial role in our perception of surroundings. People rely on sound to assess motion, distance, direction, and environmental conditions, aiding danger avoidance decision making. However, real-world environments, during the acquisition transmission audio signals, we often encounter various types noises that interfere with intended signals. As result, essential features become significantly obscured. Under interference strong noise, identifying noise segments or segments, distinguishing becomes pivotal for detecting specific events patterns isolating abnormal sounds. This study analyzes characteristics Mel’s acoustic spectrogram, explores application deep learning ECAPA-TDNN method type recognition, substantiates its effectiveness through experiments. Ultimately, experimental results demonstrate utilizing spectrogram as features, achieves notably high recognition accuracy.
Язык: Английский
Процитировано
22021 International Conference on Computational Science and Computational Intelligence (CSCI), Год журнала: 2022, Номер unknown, С. 1429 - 1435
Опубликована: Дек. 1, 2022
Respiratory diseases are one of the most prevailing which can be limited by ensuring continuous monitoring and treatment through automating lung auscultations. In this paper, an attentional deep learning model has been proposed with a new data augmentation technique named as homogeneous padding to predict respiratory using audio ICBHI-2017 dataset. Since dataset is imbalanced over classes well devices used collect data, we have performed ablation studies loss functions, reported appropriate function performs better for We also experimental analysis report position attention, observed that attention mechanism on high-level features worked in comparison low-level features. found our developed outperformed recent convolutional neural network (CNN)-based models, inclusion contributed significantly improve accuracy model.
Язык: Английский
Процитировано
3Опубликована: Июнь 7, 2023
We propose LungNet; a deep learning architecture for the diagnosis of respiratory pathologies using multi-dimensional representations lung sounds. The model is comprised two independent branches, denoted CNN and LSTM. have worked on three databases conducted experiments to evaluate performance our model. In first experiment, were merged into single dataset. second we studied distribution shift between by training one database testing it other two. Our outperformed baselines both experiments. results suggest that LungNet has potential be valuable tool diagnosing pathologies, could integrated clinical practice.
Язык: Английский
Процитировано
12022 IEEE 10th International Conference on Healthcare Informatics (ICHI), Год журнала: 2023, Номер unknown, С. 65 - 71
Опубликована: Июнь 26, 2023
Early diagnosis, treatment and regular monitoring can limit the spread adverse effects of respiratory diseases. Shortage trained physicians is one main obstacles to ensure early diagnosis which be overcome by making lung auscultations automated. To automate identify anomalies like crackles, wheezes and/or both, in this work, we propose a hybrid deep learning model combining Convolutional Neural Network (CNN) model, ResNet34 as feature extractor, Long Short-Term Memory (LSTM) predictor, along with novel augmentation technique called homogeneous padding over ICBHI-2017 dataset. We have also added an attention layer extractor allow learn important region vector. The proposed has outperformed recent state-of-the-art models regard. found that inclusion layer, LSTM predictor improved performance our 2-class 4-class anomaly predictions.
Язык: Английский
Процитировано
1