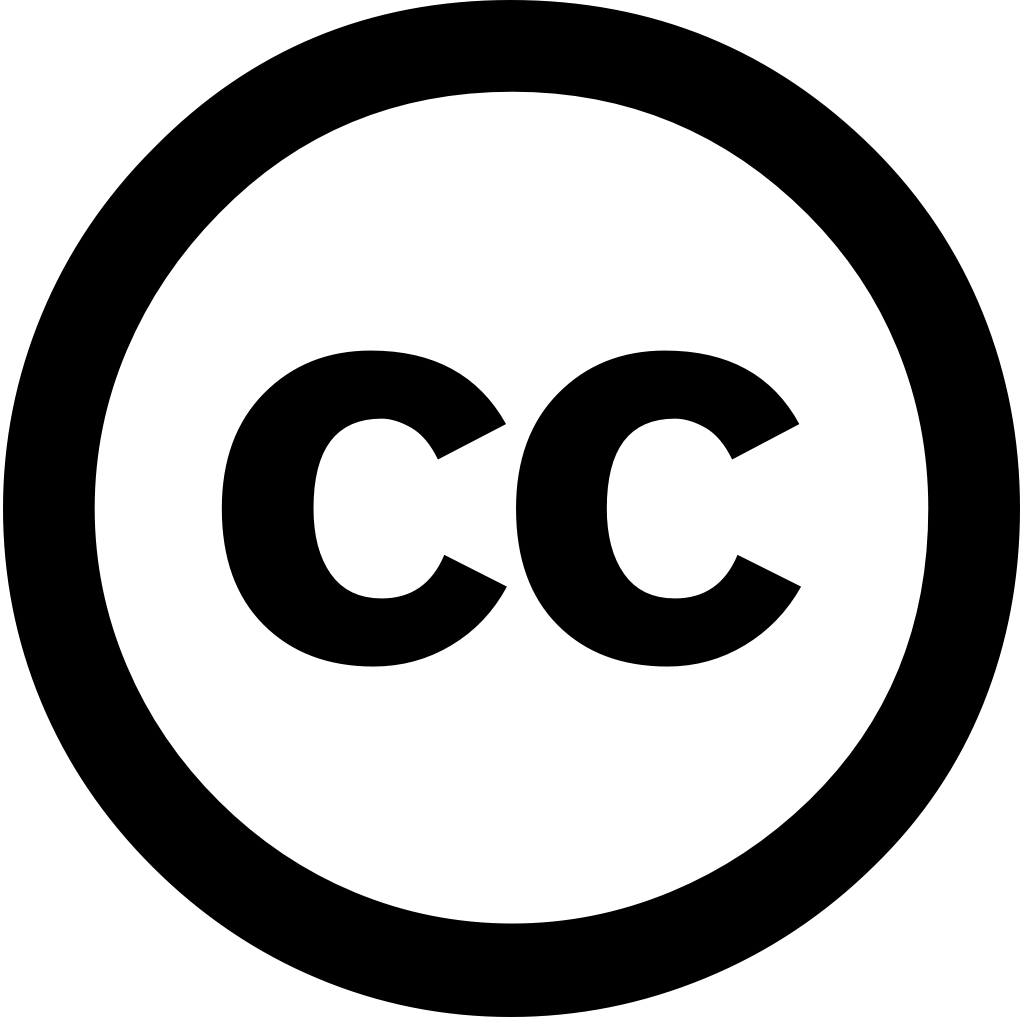
Diagnostics, Год журнала: 2025, Номер 15(8), С. 976 - 976
Опубликована: Апрель 11, 2025
Background and Objective: Using echocardiogram data for cardiovascular disease (CVD) can lead to difficulties due imbalanced datasets, leading biased predictions. Machine learning models enhance prognosis accuracy, but their effectiveness is influenced by optimal feature selection robust classification techniques. This study introduces an event-based self-similarity approach automatic data. Critical features correlated with progression were identified leveraging patterns. used dataset, visual presentations of high-frequency sound wave signals, patients heart who are treated using three treatment methods: catheter ablation, ventricular defibrillator, drug control-over the course years. Methods: The dataset was classified into nine categories Recursive Feature Elimination (RFE) applied identify most relevant features, reducing model complexity while maintaining diagnostic accuracy. models, including XGBoost CATBoost, trained evaluated. Results: Both achieved comparable accuracy values, 84.3% 88.4%, respectively, under different normalization To further optimize performance, combined a voting ensemble, improving predictive Four essential features-age, aorta (AO), left (LV), atrium (LA)-were as critical found in Random Forest (RF)-voting ensemble classifier. results underscore importance techniques handling robustness, bias automated systems. Conclusions: Our findings highlight potential machine learning-driven analysis patient care providing accurate, data-driven assessments.
Язык: Английский