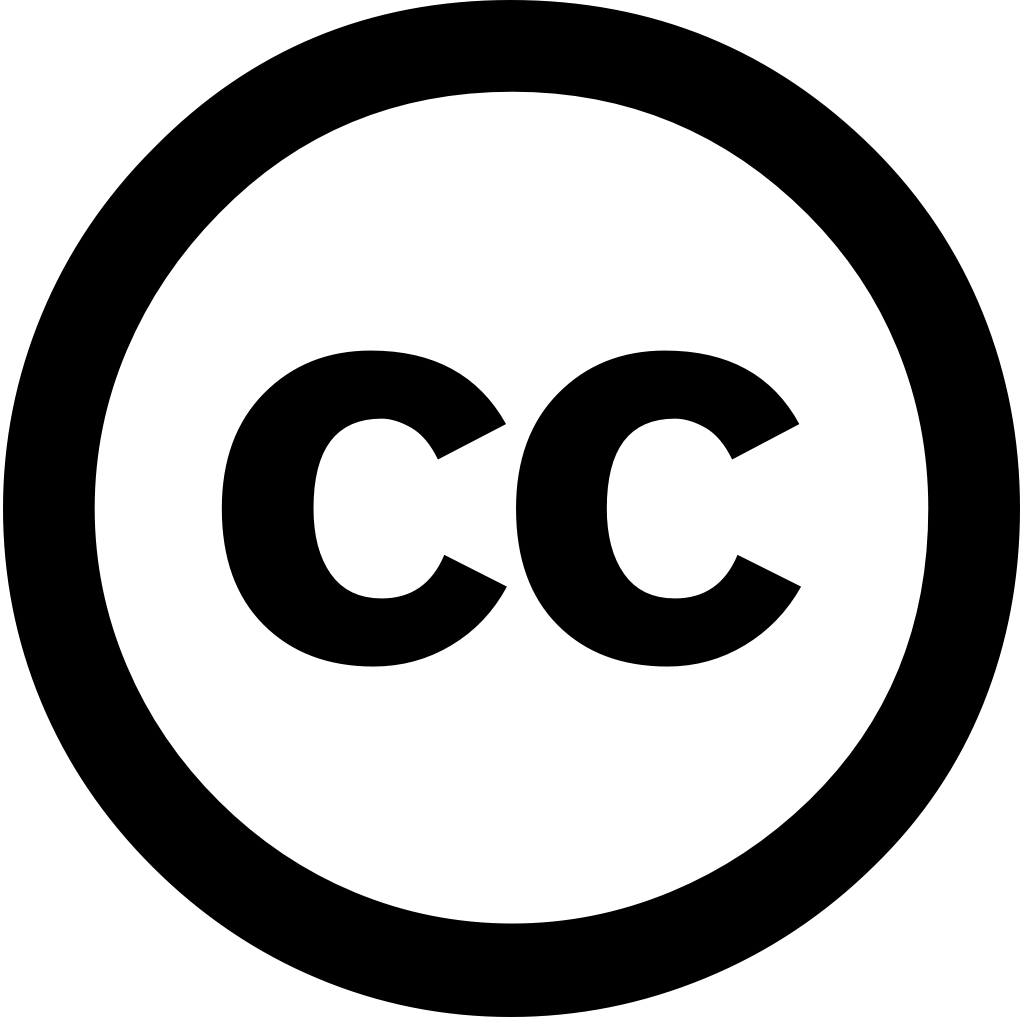
Mathematics, Год журнала: 2025, Номер 13(6), С. 894 - 894
Опубликована: Март 7, 2025
Learning high-dimensional chaos is a complex and challenging problem because of its initial value-sensitive dependence. Based on an echo state network (ESN), we introduce homotopy transformation in topological theory to learn chaos. On the premise maintaining basic properties, our model can obtain key features for learning through continuous between different activation functions, achieving optimal balance nonlinearity linearity enhance generalization capability model. In experimental part, choose Lorenz system, Mackey–Glass (MG) Kuramoto–Sivashinsky (KS) system as examples, verify superiority by comparing it with other models. For some systems, prediction error be reduced two orders magnitude. The results show that addition improve modeling ability spatiotemporal chaotic this demonstrates potential application dynamic time series analysis.
Язык: Английский