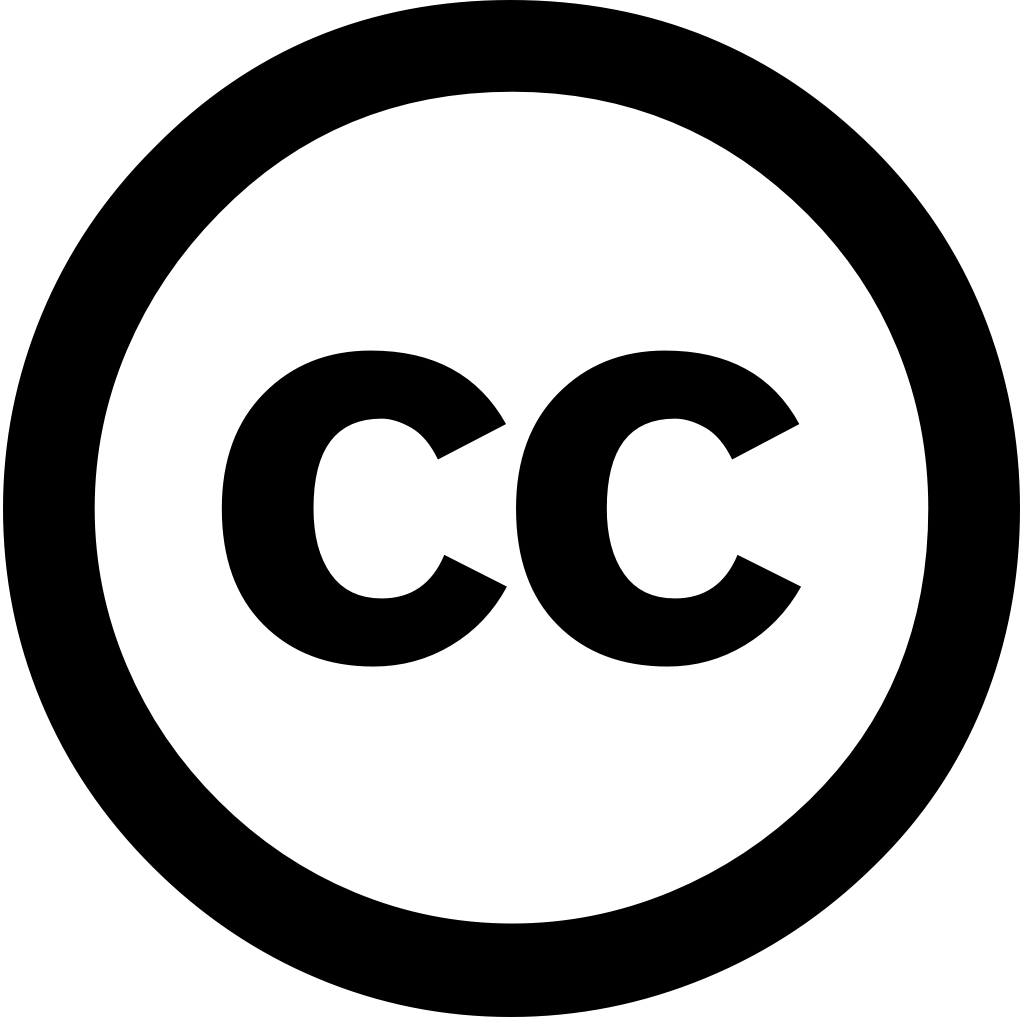
IEEE Access, Год журнала: 2024, Номер 12, С. 89968 - 89985
Опубликована: Янв. 1, 2024
Язык: Английский
IEEE Access, Год журнала: 2024, Номер 12, С. 89968 - 89985
Опубликована: Янв. 1, 2024
Язык: Английский
Computers in Biology and Medicine, Год журнала: 2025, Номер 186, С. 109659 - 109659
Опубликована: Янв. 22, 2025
Язык: Английский
Процитировано
1Neurocomputing, Год журнала: 2025, Номер unknown, С. 129731 - 129731
Опубликована: Фев. 1, 2025
Язык: Английский
Процитировано
0Archives of Computational Methods in Engineering, Год журнала: 2025, Номер unknown
Опубликована: Март 13, 2025
Язык: Английский
Процитировано
0Computer Science Review, Год журнала: 2025, Номер 57, С. 100751 - 100751
Опубликована: Апрель 4, 2025
Язык: Английский
Процитировано
0International Journal of experimental research and review, Год журнала: 2024, Номер 42, С. 120 - 132
Опубликована: Авг. 30, 2024
This research has been globally impacted by COVID-19 virus, which was a very uncommon, highly contagious & dangerous respiratory illness demanding early detection for effective containment and further spread. In this research, we proposed an innovative methodology that utilizes images of X-rays at stage. By employing convolution neural network, enhance the accuracy performance via using ResNet50v2 hyperparameter. The achieves remarkable with average 99.12%. surpasses other available models based on different deep learning like VGG, Xception DenseNet COVID identification help X-ray images. scans are now preferably used modality COVID-19, given its widespread utilization effectiveness. However, manual treatment examination is challenging, specifically in field facing limitation skilled medical staff. Utilization demonstrated significant potential results automating diagnosis timely films. suggested architecture developed prediction analysis cases It firmly believes study holds alleviating workload frontline radiologists, expediting patient treatment, facilitating pandemic control efforts.
Язык: Английский
Процитировано
3Computers in Biology and Medicine, Год журнала: 2024, Номер 185, С. 109461 - 109461
Опубликована: Дек. 3, 2024
Язык: Английский
Процитировано
1bioRxiv (Cold Spring Harbor Laboratory), Год журнала: 2024, Номер unknown
Опубликована: Апрель 22, 2024
Abstract SARS-CoV-2 can infect alveoli, inducing a lung injury and thereby impairing the function. Healthy alveolar type II (AT2) cells play major role in repair as well keeping alveoli space free from fluids, which is not case for infected AT2 cells. Unlike previous studies, this novel study aims to automatically differentiate between healthy with through using efficient AI-based models, aid disease control treatment. Therefore, we introduce highly accurate deep transfer learning (DTL) approach that works follows. First, downloaded processed 286 images pertaining human (hAT2) cells, obtained electron microscopy public image archive. Second, provided two DTL computations induce ten models. The first computation employs five pre-trained models (including DenseNet201 ResNet152V2) trained on more than million ImageNet database extract features hAT2 images. Then, flattening providing output feature vectors densely connected classifier Adam optimizer. second similar manner minor difference freeze layers extraction while unfreezing training next layers. Compared TFtDenseNet201, experimental results five-fold cross-validation demonstrate TFeDenseNet201 12.37 × faster superior, yielding highest average ACC of 0.993 (F1 0.992 MCC 0.986) statistical significance ( p < 2.2 10 −16 t -test).
Язык: Английский
Процитировано
0Iran Journal of Computer Science, Год журнала: 2024, Номер 7(3), С. 699 - 721
Опубликована: Май 2, 2024
Язык: Английский
Процитировано
0Mathematics, Год журнала: 2024, Номер 12(10), С. 1573 - 1573
Опубликована: Май 17, 2024
Transmission electron microscopy imaging provides a unique opportunity to inspect the detailed structure of infected lung cells with SARS-CoV-2. Unlike previous studies, this novel study aims investigate COVID-19 classification at cellular level in response Particularly, differentiating between healthy and human alveolar type II (hAT2) Hence, we explore feasibility deep transfer learning (DTL) introduce highly accurate approach that works as follows: First, downloaded processed 286 images pertaining hAT2 obtained from public image archive. Second, provided two DTL computations induce ten models. The first computation employs five pre-trained models (including DenseNet201 ResNet152V2) trained on more than one million ImageNet database extract features images. Then, it flattens output feature vectors trained, densely connected classifier Adam optimizer. second similar manner, minor difference freeze layers for extraction while unfreezing jointly training next layers. results using five-fold cross-validation demonstrated TFeDenseNet201 is 12.37× faster superior, yielding highest average ACC 0.993 (F1 0.992 MCC 0.986) statistical significance (P<2.2×10−16 t-test) compared an 0.937 0.938 0.877) counterpart (TFtDenseNet201), showing no (P=0.093 t-test).
Язык: Английский
Процитировано
0IEEE Access, Год журнала: 2024, Номер 12, С. 89968 - 89985
Опубликована: Янв. 1, 2024
Язык: Английский
Процитировано
0