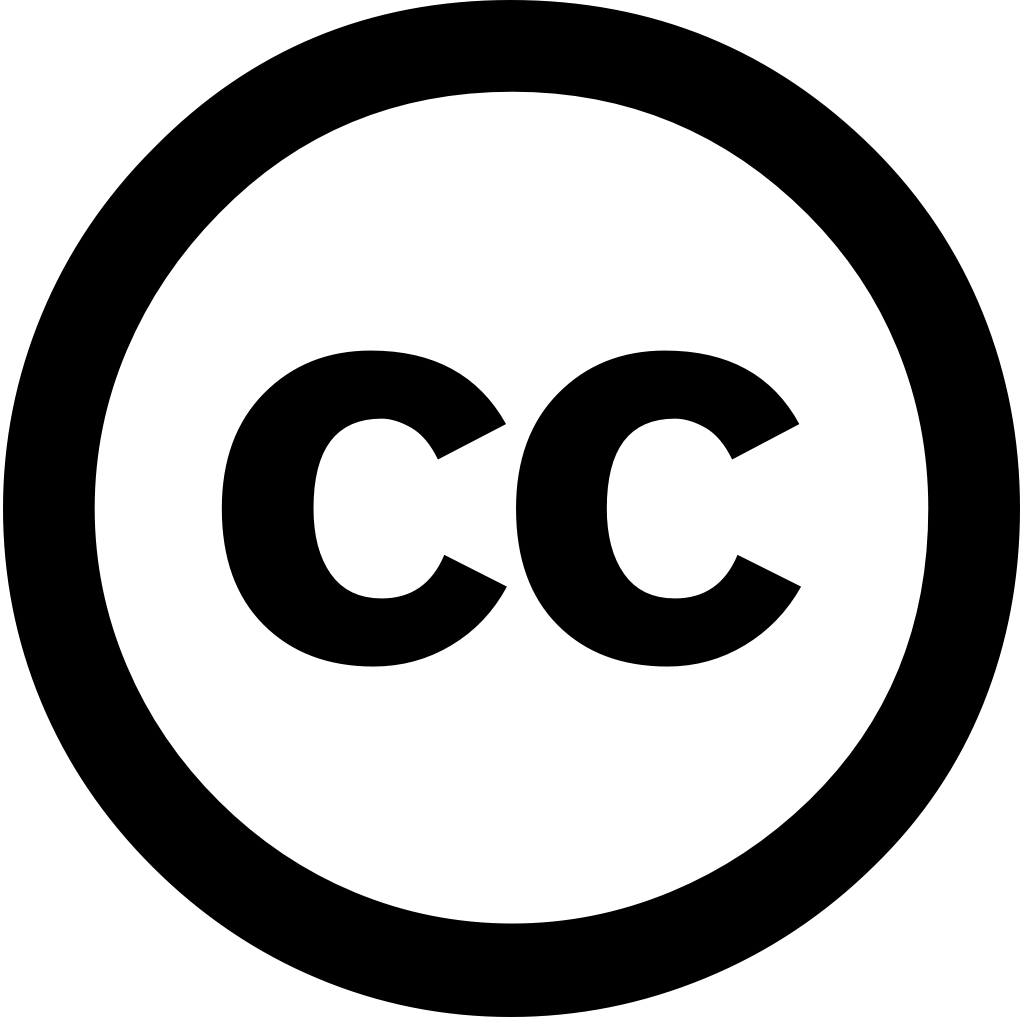
Computer Science Review, Год журнала: 2024, Номер 56, С. 100717 - 100717
Опубликована: Дек. 20, 2024
Язык: Английский
Computer Science Review, Год журнала: 2024, Номер 56, С. 100717 - 100717
Опубликована: Дек. 20, 2024
Язык: Английский
Computers & Electrical Engineering, Год журнала: 2025, Номер 123, С. 110046 - 110046
Опубликована: Янв. 4, 2025
Язык: Английский
Процитировано
1Sensors, Год журнала: 2025, Номер 25(3), С. 967 - 967
Опубликована: Фев. 6, 2025
Traffic flow prediction can guide the rational layout of land use. Accurate traffic provide an important basis for urban expansion planning. This paper introduces a personalized lightweight federated learning framework (PLFL) prediction. has been improved and enhanced to better accommodate data. It is capable collaboratively training unified global model without compromising privacy individual datasets. Specifically, spatiotemporal fusion graph convolutional network (MGTGCN) established as initial learning. Subsequently, shared parameter mechanism employed training. Customized weights are allocated each client based on their data features enhance personalization during this process. In order improve communication efficiency learning, dynamic pruning (DMP) introduced side reduce number parameters that need be communicated. Finally, PLFL proposed in experimentally validated using LPR from Changsha city. The results demonstrate still achieve favorable outcomes even when certain clients lack Moreover, under while preserving distinct characteristics client, significant interference other clients.
Язык: Английский
Процитировано
0Опубликована: Янв. 1, 2025
Язык: Английский
Процитировано
0Computer Science Review, Год журнала: 2024, Номер 56, С. 100717 - 100717
Опубликована: Дек. 20, 2024
Язык: Английский
Процитировано
0