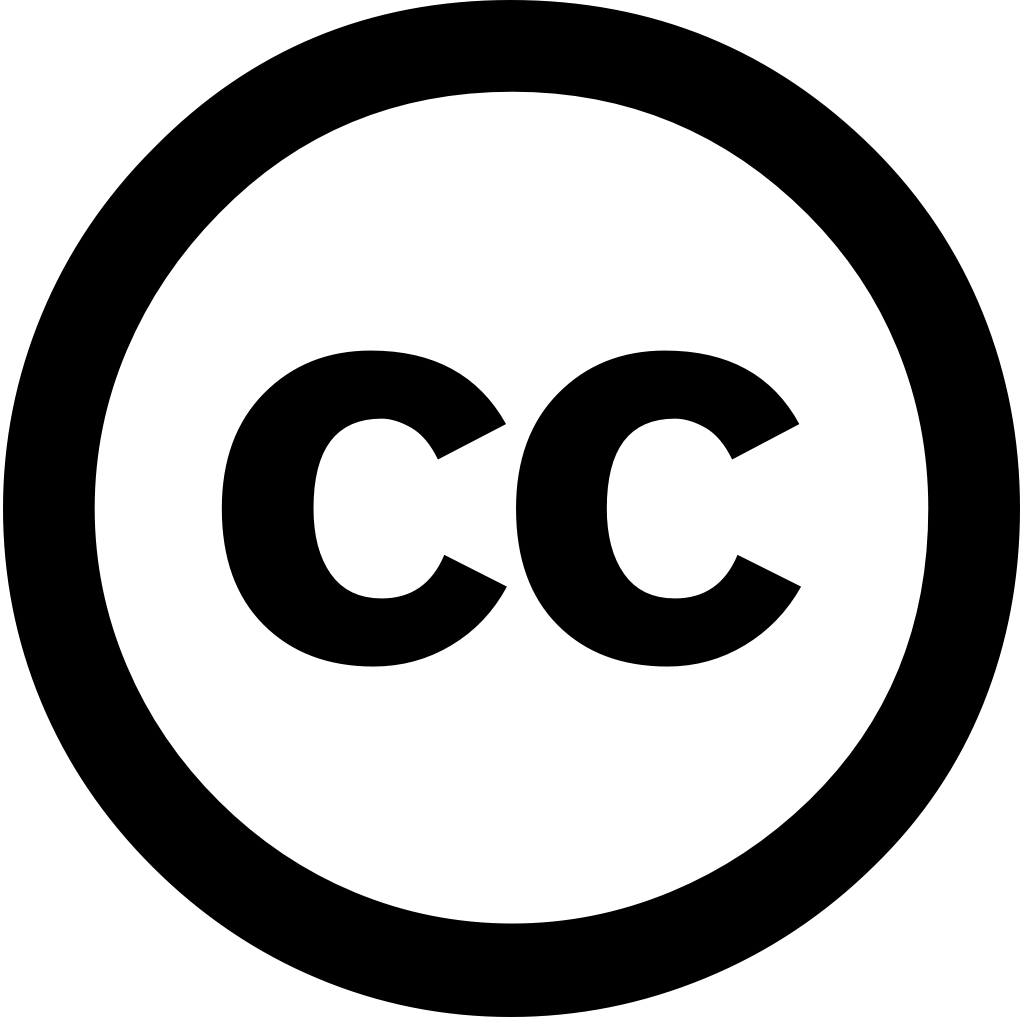
Discover Sustainability, Год журнала: 2024, Номер 5(1)
Опубликована: Дек. 21, 2024
Язык: Английский
Discover Sustainability, Год журнала: 2024, Номер 5(1)
Опубликована: Дек. 21, 2024
Язык: Английский
e-Prime - Advances in Electrical Engineering Electronics and Energy, Год журнала: 2024, Номер 9, С. 100674 - 100674
Опубликована: Июль 4, 2024
The increasing demand for sustainable and renewable energy solutions reflects the critical importance of advancing photovoltaic (PV) technology its operational efficiency. In response, this study introduces a novel application k-Nearest Neighbor (k-NN) algorithm to assess reliability applicability solar panel simulation data which aimed classify current states partial shading, open, short circuit conditions, alongside regression-based analysis predicting specific operating parameters. research, published dataset that involved various PV module configurations under different environmental conditions was tested evaluated. k-NN technique applied both status predict performance metrics modules. diagnosis model demonstrated an accuracy 99.2 % F1 score %, indicating high degree in identifying Concurrently, regression exhibited Root Mean Square Error (RMSE) 0.036 R2 value unity showcased effectiveness parameters based on data. concluded results are further enriching simulation-based generation be endorsed implemented before jumping into real experimental applications, addition highlighting potential machine learning cells productivity statistical analysis.
Язык: Английский
Процитировано
6Journal of Water Process Engineering, Год журнала: 2025, Номер 71, С. 107363 - 107363
Опубликована: Фев. 26, 2025
Язык: Английский
Процитировано
0Desalination and Water Treatment, Год журнала: 2025, Номер unknown, С. 101179 - 101179
Опубликована: Апрель 1, 2025
Язык: Английский
Процитировано
0Separation and Purification Technology, Год журнала: 2025, Номер unknown, С. 133031 - 133031
Опубликована: Апрель 1, 2025
Язык: Английский
Процитировано
0Desalination and Water Treatment, Год журнала: 2024, Номер 320, С. 100685 - 100685
Опубликована: Авг. 3, 2024
This study capitalizes on a dataset, originally including 280 sensory measurements from laboratory-scale water distribution system, to advance the concept of leakage diagnosis and localization. The test rig are formulated in two configurations, namely looped branched layouts. paper processed time-domain data accelerometers dynamic pressure sensors into advanced statistical features of: Autocorrelation Coefficient (Au-C), Signal Energy (Sig-E), detect localize leakage. By Employment these features, research developed an expert system Artificial Neural Network (ANN) model designed with optimal parameters, neurons, hidden layers classify presence pinpoint location leaks within rig. effectiveness current approach is quantitatively evaluated using F1-scores accuracy metrics. A robust capability for both detecting localizing under varying conditions was established highest F1-score 86.5 % 86.2 %, respectively. findings underscore potential integrating Intelligence (AI) enhancing reliability dependability management systems. contributes broader application AI managing resources infrastructure resilience its support improve whereabouts.
Язык: Английский
Процитировано
3Desalination and Water Treatment, Год журнала: 2024, Номер 320, С. 100683 - 100683
Опубликована: Авг. 3, 2024
The integration of renewable energy sources with multi-energy systems present challenges and opportunities to enhance sustainability. Among these, solar stills have emerged as a solution for water desalination. With the advent expert system technologies, avenues are opened improving operational efficiency distillers. This paper presents an innovative approach utilizing correlation analysis, ReliefF feature selection, k-Nearest Neighbor (kNN) algorithm forecasting cumulative distillate output double slope still. analysis is based on 6-cases-based dataset, which includes variations in relative different operational-environmental conditions. Key features that significantly impact overall performance were identified manage distiller productivity. findings reveal maximum was 1610 ML/m2.day due incorporating reflective materials phase change (PCM) enhancing distillation rates. kNN model evaluated its R2, RMSE, CVRMSE, best models achieving scores 0.995, 0.0033, 0.1666, respectively. These metrics underscore effectiveness proposed machine learning predicting output, thereby enabling informed management processes. Combining technologies computational intelligence holds significant promise sustainable environmental management, study presented.
Язык: Английский
Процитировано
3Desalination and Water Treatment, Год журнала: 2024, Номер 320, С. 100735 - 100735
Опубликована: Авг. 25, 2024
Язык: Английский
Процитировано
3Computers in Biology and Medicine, Год журнала: 2024, Номер 182, С. 109241 - 109241
Опубликована: Окт. 2, 2024
Язык: Английский
Процитировано
2Journal of Failure Analysis and Prevention, Год журнала: 2024, Номер unknown
Опубликована: Ноя. 15, 2024
Язык: Английский
Процитировано
2Results in Engineering, Год журнала: 2024, Номер unknown, С. 103823 - 103823
Опубликована: Дек. 1, 2024
Язык: Английский
Процитировано
2