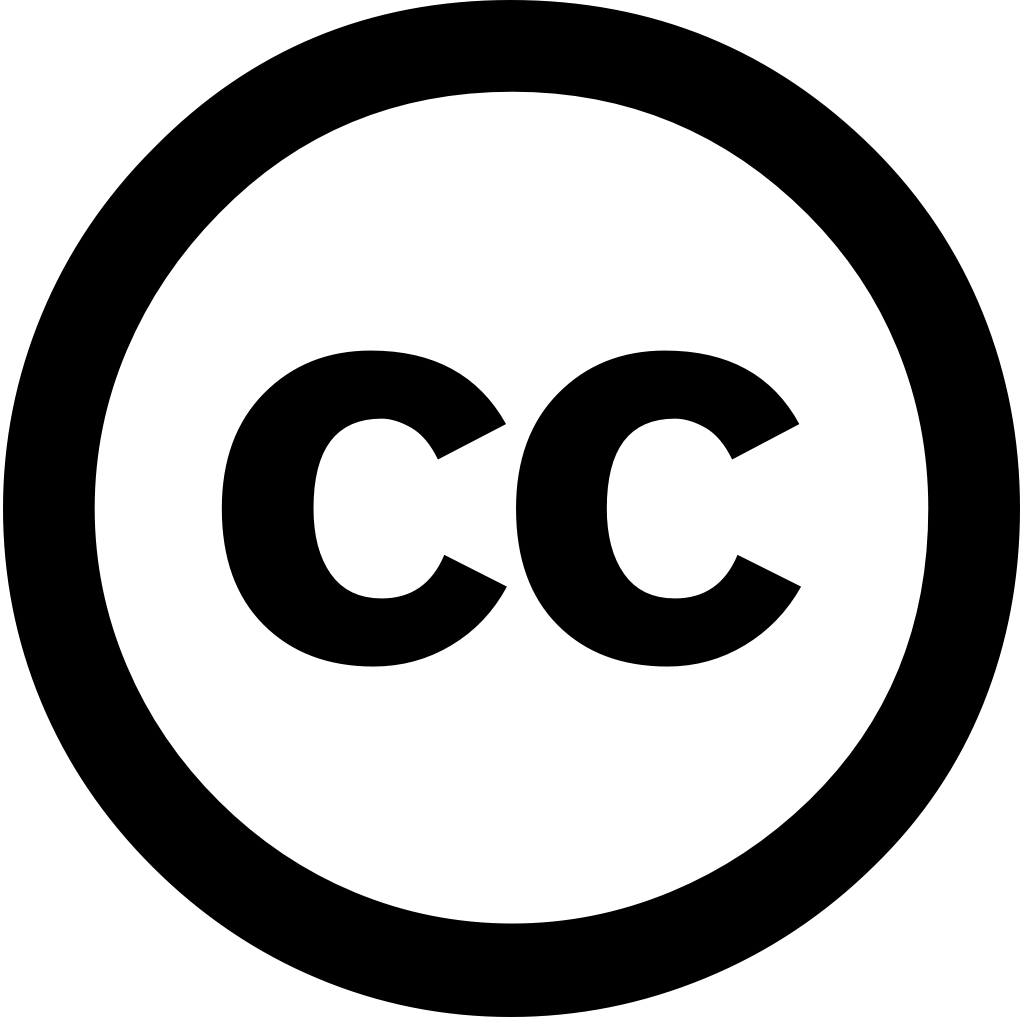
Land, Год журнала: 2025, Номер 14(1), С. 69 - 69
Опубликована: Янв. 2, 2025
Nitrate leaching from soil presents a significant threat to health, as it can result in nutrient loss, acidification, and structural damage. It is crucial quantify the spatial heterogeneity of nitrate its drivers. A total 509 observational data points regarding northern China were collected, capturing temporal variations across crops such winter wheat, maize, greenhouse vegetables. machine learning (ML) model for predicting was then developed, with random forest (RF) outperforming support vector (SVM), extreme gradient boosting (XGBoost), convolutional neural network (CNN) models, achieving an R2 0.75. However, performance improved significantly after integrating four models Bayesian optimization (all had > 0.56), which realized quantitative prediction capabilities loss concentrations. Moreover, XGBoost exhibited highest fitting accuracy smallest error estimating losses, value 0.79 average absolute (MAE) 3.87 kg/ha. Analyses feature importance SHAP values optimal identified organic matter, chemical nitrogen fertilizer input, water input (including rainfall irrigation) main indicators loss. The ML-based modeling method developed overcomes difficulty determination functional relationship between intensity influencing factors, providing data-driven solution nitrate–nitrogen farmlands North strengthening sustainable agricultural practices.
Язык: Английский