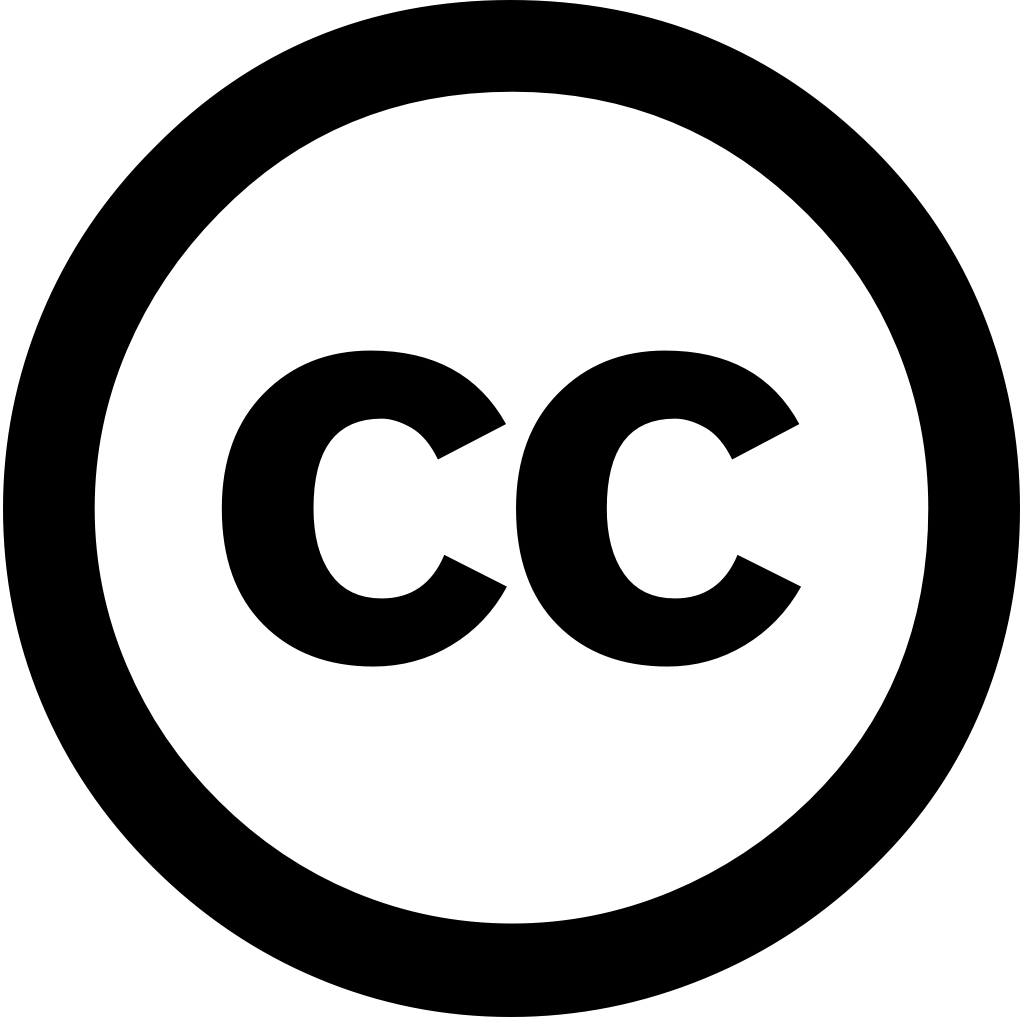
Animals, Год журнала: 2025, Номер 15(7), С. 913 - 913
Опубликована: Март 22, 2025
This study classified cows' foraging behaviors using machine learning (ML) models evaluated through random test split (RTS) and cross-validation (CV) data partition methods. Models included Perceptron, Logistic Regression, Support Vector Machine, K-Nearest Neighbors, Random Forest (RF), XGBoost (XGB). These activity states (active vs. static), (grazing (GR), resting (RE), walking (W), ruminating (RU)), posture (standing up (SU) lying down (LD)), combinations with rumination (RU_SU, RU_LD, RE_SU, RE_LD). XGB achieved the highest accuracy for state classification (74.5% RTS, 74.2% CV) behavior (69.4% CV). RF outperformed in other classifications, including GR, RE, RU (62.9% CV 56.4% RTS), (83.9% 79.4% behaviors-by-posture (58.8% RTS). Key predictors varied: speed Actindex were crucial GR W when increasing RE decreasing. X low values linked to RE_SU RU_SU, while Z influenced RE_LD more. RTS showed higher by classification. results emphasize RF's reliability managing complex behavioral patterns importance of continuous recording devices movement monitor cattle accurately.
Язык: Английский