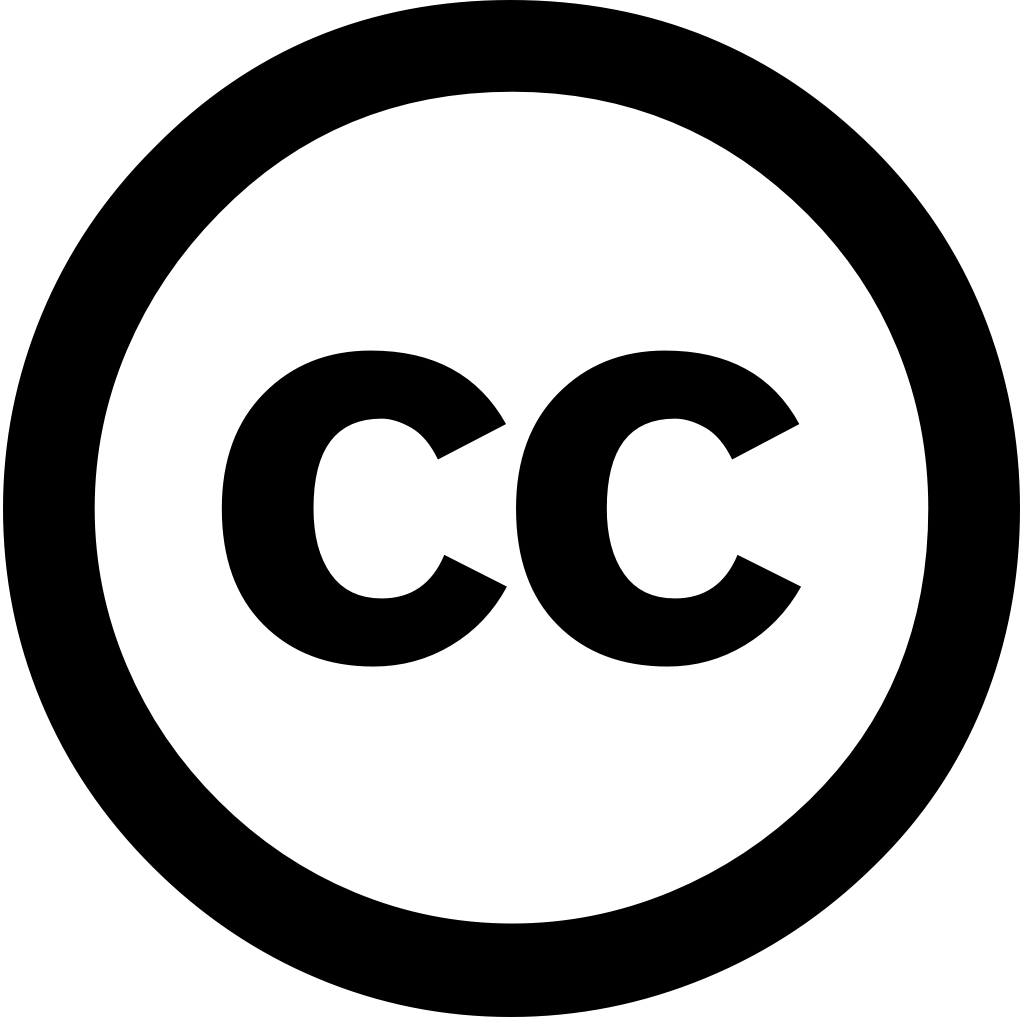
Research Square (Research Square), Год журнала: 2024, Номер unknown
Опубликована: Июнь 26, 2024
Язык: Английский
Research Square (Research Square), Год журнала: 2024, Номер unknown
Опубликована: Июнь 26, 2024
Язык: Английский
Current Problems in Diagnostic Radiology, Год журнала: 2024, Номер 53(6), С. 728 - 737
Опубликована: Июль 9, 2024
The rise of transformer-based large language models (LLMs), such as ChatGPT, has captured global attention with recent advancements in artificial intelligence (AI). ChatGPT demonstrates growing potential structured radiology reporting—a field where AI traditionally focused on image analysis. A comprehensive search MEDLINE and Embase was conducted from inception through May 2024, primary studies discussing ChatGPT's role reporting were selected based their content. Of the 268 articles screened, eight ultimately included this review. These explored various applications generating reports unstructured reports, extracting data free text, impressions findings creating imaging data. All demonstrated optimism regarding to aid radiologists, though common critiques privacy concerns, reliability, medical errors, lack medical-specific training. assistive have significant transform reporting, enhancing accuracy standardization while optimizing healthcare resources. Future developments may involve integrating dynamic few-shot prompting, Retrieval Augmented Generation (RAG) into diagnostic workflows. Continued research, development, ethical oversight are crucial fully realize AI's radiology.
Язык: Английский
Процитировано
12European Radiology, Год журнала: 2024, Номер unknown
Опубликована: Окт. 23, 2024
Abstract Structured reporting (SR) has long been a goal in radiology to standardize and improve the quality of reports. Despite evidence that SR reduces errors, enhances comprehensiveness, increases adherence guidelines, its widespread adoption limited. Recently, large language models (LLMs) have emerged as promising solution automate facilitate SR. Therefore, this narrative review aims provide an overview LLMs for beyond. We found current literature on is limited, comprising ten studies generative pre-trained transformer (GPT)-3.5 ( n = 5) and/or GPT-4 8), while two additionally examined performance Perplexity Bing Chat or IT5. All reported results acknowledged potential SR, with six out demonstrating feasibility multilingual applications. Building upon these findings, we discuss limitations, regulatory challenges, further applications report processing, encompassing four main areas: documentation, translation summarization, clinical evaluation, data mining. In conclusion, underscores transformative efficiency accuracy processing. Key Points Question How can help make more ubiquitous ? Findings Current leveraging sparse but shows results, including . Clinical relevance transform processing enable However, their future role practice depends overcoming limitations opaque algorithms training
Язык: Английский
Процитировано
8medRxiv (Cold Spring Harbor Laboratory), Год журнала: 2024, Номер unknown
Опубликована: Авг. 12, 2024
Background: Generative Large language models (LLMs) represent a significant advancement in natural processing, achieving state-of-the-art performance across various tasks. However, their application clinical settings using real electronic health records (EHRs) is still rare and presents numerous challenges. Objective: This study aims to systematically review the use of generative LLMs, effectiveness relevant techniques patient care-related topics involving EHRs, summarize challenges faced, suggest future directions. Methods: A Boolean search for peer-reviewed articles was conducted on May 19th, 2024 PubMed Web Science include research published since 2023, which one month after release ChatGPT. The results were deduplicated. Multiple reviewers, including biomedical informaticians, computer scientists, physician, screened publications eligibility data extraction. Only studies utilizing LLMs analyze EHR included. We summarized prompt engineering, fine-tuning, multimodal data, evaluation matrices. Additionally, we identified current applying as reported by included proposed Results: initial 6,328 unique studies, with 76 screening. Of these, 67 (88.2%) employed zero-shot prompting, five them 100% accuracy specific Nine used advanced prompting strategies; four tested these strategies experimentally, finding that engineering improved performance, noting non-linear relationship between number examples improvement. Eight explored fine-tuning all improvements tasks, but three noted potential degradation certain two utilized LLM-based decision-making enabled accurate disease diagnosis prognosis. 55 different metrics 22 purposes, such correctness, completeness, conciseness. Two investigated LLM bias, detecting no bias other male patients received more appropriate suggestions. Six hallucinations, fabricating names structured thyroid ultrasound reports. Additional not limited impersonal tone consultations, made uncomfortable, difficulty had understanding responses. Conclusion: Our indicates few have computational enhance performance. diverse highlight need standardization. currently cannot replace physicians due
Язык: Английский
Процитировано
4Expert Systems with Applications, Год журнала: 2025, Номер unknown, С. 126585 - 126585
Опубликована: Янв. 1, 2025
Язык: Английский
Процитировано
0Deleted Journal, Год журнала: 2025, Номер unknown
Опубликована: Фев. 4, 2025
Aufgrund des andauernden, rapiden Fortschritts künstlicher Intelligenz (KI) inklusive Large Language Models (LLMs) werden Radiolog*innen in absehbarer Zeit vor die Herausforderung der verantwortungsvollen klinischen Integration dieser Modelle gestellt. Ziel Arbeit ist es, einen Überblick über aktuelle Entwicklungen zum Thema LLMs, mögliche Einsatzgebiete Radiologie sowie ihre (zukünftige) Relevanz und Limitationen zu liefern. In Übersichtsarbeit wurden Publikationen LLMs für spezifische Anwendungen Medizin analysiert. Zusätzlich wurde Literatur den Herausforderungen im Zusammenhang mit einer LLM-Nutzung gesichtet zusammengefasst. Neben einem generellen radiologischen Anwendungsbeispielen von verschiedene besonders spannende Arbeiten empfohlen. Um anstehende klinische ermöglichen, müssen sich Thematik auseinandersetzen, Anwendungsgebiete möglicher kennen, um Hinblick auf Patientensicherheit, Ethik Datenschutz bewältigen können.
Процитировано
0Diagnostics, Год журнала: 2025, Номер 15(4), С. 497 - 497
Опубликована: Фев. 19, 2025
Background/Objectives: Despite the growing popularity of large language models (LLMs), there remains a notable lack research examining their role in workplace management. This study aimed to address this gap by evaluating performance ChatGPT-3.5, ChatGPT-4.0, Gemini, and Gemini Advanced as famous LLMs responding management questions specific radiology. Methods: ChatGPT-3.5 ChatGPT-4.0 (both OpenAI, San Francisco, CA, USA) Google Deep Mind, Mountain View, generated answers 31 pre-selected on four different areas radiology: (1) patient management, (2) imaging radiation (3) learning personal development, (4) administrative department Two readers independently evaluated provided LLM chatbots. Three 4-point scores were used assess quality responses: overall score (OQS), understandabilityscore (US), implementability (IS). The mean (MQS) was calculated from these three scores. Results: inter-rater reliability (IRR) good for (IRR 79%), 78%), 65%), moderate 54%). MQS averaged 3.36 (SD: 0.64) 3.75 0.43) 3.29 3.51 0.53) Advanced. highest OQS, US, IS, achieved all categories, followed most consistently superior performer outperformed other chatbots (p < 0.001-0.002). performed significantly better than = 0.003) showed non-significant trend toward outperforming 0.056). cases compared with None rated "insufficient". Conclusions: All well Our revealed that have potential improve radiology assisting various tasks, making processes more efficient without requiring specialized skills.
Язык: Английский
Процитировано
0Clinical and Translational Science, Год журнала: 2025, Номер 18(4)
Опубликована: Апрель 1, 2025
ABSTRACT Large language models (LLMs) have emerged as powerful tools in many fields, including clinical pharmacology and translational medicine. This paper aims to provide a comprehensive primer on the applications of LLMs these disciplines. We will explore fundamental concepts LLMs, their potential drug discovery development processes ranging from facilitating target identification aiding preclinical research trial analysis, practical use cases such assisting with medical writing accelerating analytical workflows quantitative pharmacology. By end this paper, pharmacologists scientists clearer understanding how leverage enhance efforts.
Язык: Английский
Процитировано
0Scientific Reports, Год журнала: 2025, Номер 15(1)
Опубликована: Апрель 26, 2025
Язык: Английский
Процитировано
0Endocrine, Год журнала: 2024, Номер unknown
Опубликована: Окт. 11, 2024
Abstract Purpose Large language models (LLMs) are pivotal in artificial intelligence, demonstrating advanced capabilities natural understanding and multimodal interactions, with significant potential medical applications. This study explores the feasibility efficacy of LLMs, specifically ChatGPT-4o Claude 3-Opus, classifying thyroid nodules using ultrasound images. Methods included 112 patients a total 116 nodules, comprising 75 benign 41 malignant cases. Ultrasound images these were analyzed 3-Opus to diagnose or nature nodules. An independent evaluation by junior radiologist was also conducted. Diagnostic performance assessed Cohen’s Kappa receiver operating characteristic (ROC) curve analysis, referencing pathological diagnoses. Results demonstrated poor agreement results ( = 0.116), while showed even lower 0.034). The exhibited moderate 0.450). achieved an area under ROC (AUC) 57.0% (95% CI: 48.6–65.5%), slightly outperforming (AUC 52.0%, 95% 43.2–60.9%). In contrast, significantly higher AUC 72.4% 63.7–81.1%). unnecessary biopsy rates 41.4% for ChatGPT-4o, 43.1% 12.1% radiologist. Conclusion While LLMs such as show promise future applications imaging, their current use clinical diagnostics should be approached cautiously due limited accuracy.
Язык: Английский
Процитировано
3La radiologia medica, Год журнала: 2024, Номер unknown
Опубликована: Ноя. 7, 2024
Язык: Английский
Процитировано
3