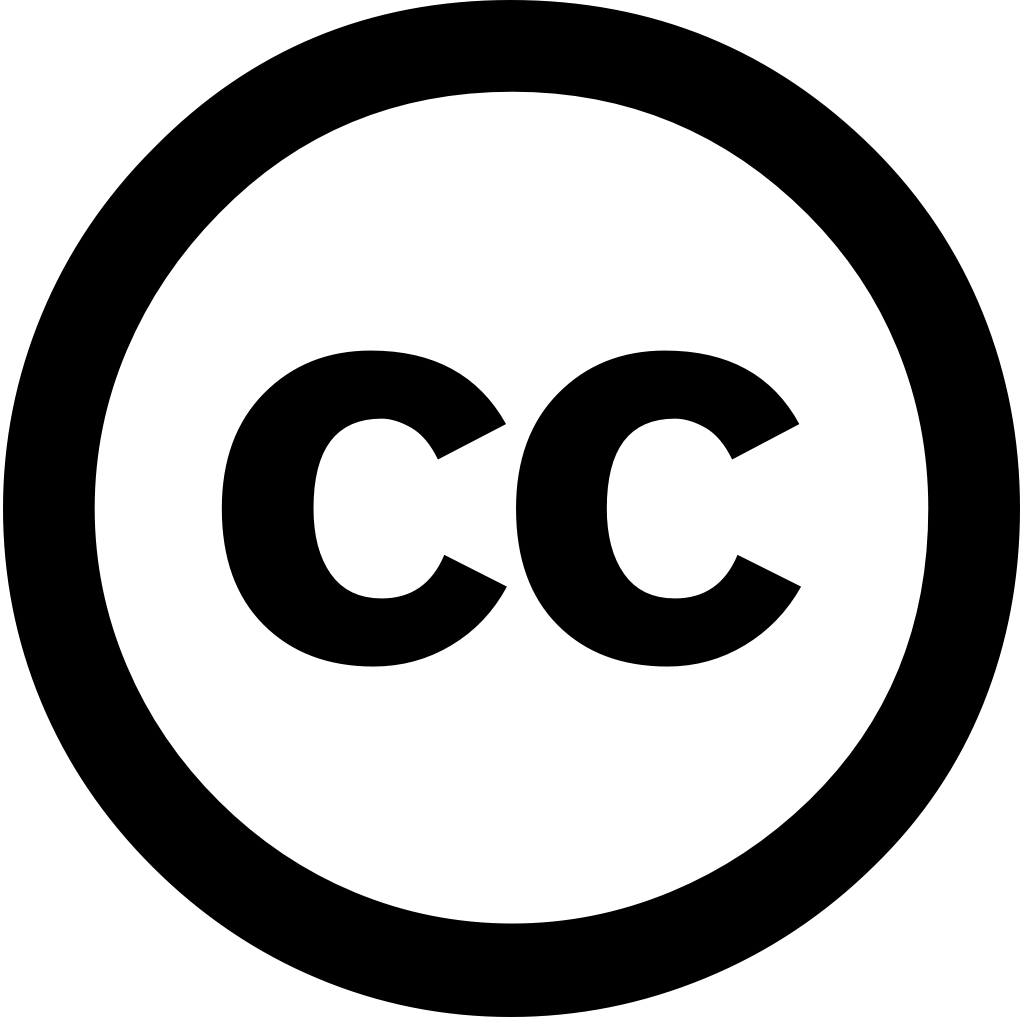
IEEE Access, Год журнала: 2024, Номер 12, С. 168904 - 168924
Опубликована: Янв. 1, 2024
Язык: Английский
IEEE Access, Год журнала: 2024, Номер 12, С. 168904 - 168924
Опубликована: Янв. 1, 2024
Язык: Английский
Applied Energy, Год журнала: 2024, Номер 369, С. 123467 - 123467
Опубликована: Июнь 5, 2024
Solar forecasting from ground-based sky images has shown great promise in reducing the uncertainty solar power generation. With more and image datasets available recent years, development of accurate reliable deep learning-based methods using diverse multi-location data seen a huge growth potential. From that perspective, joint utilization these heterogeneous – such as captured with different camera setups, sensor measurements (i.e., irradiance versus photovoltaic output) varying scale distribution is both unique opportunity critical challenge. This study explores ways to cope heterogeneity compares three strategies for training models based on collected across continents by research groups. Specifically, location interest, we compare performance (1) local trained individually single dataset standard methodology literature); (2) global jointly fusion multiple datasets; (3) locally fine-tuned via transfer learning pre-trained model. The results suggest that, current modeling strategy, work well when deployed locally, but significant errors are observed applied offsite. model, proper normalization prediction targets, can adapt individual locations at cost potential increase efforts. Pre-training large diversified source transferring target generally achieves superior over other two strategies. 80% less data, model performs similarly baseline entire dataset. Overall, algorithms built have be accurate, robust, faster new than location.
Язык: Английский
Процитировано
13Solar RRL, Год журнала: 2025, Номер unknown
Опубликована: Янв. 10, 2025
Accurate very short‐term solar irradiance forecasting is crucial for optimizing the integration of energy into power systems. Herein, an image‐based deep learning framework minute‐scale prediction presented. The locally developed model benchmarked against two commercial solutions deployed at same experimental site, demonstrating superior accuracy and adaptability. A key contribution introduction a skill‐driven sampling algorithm based on clear sky index persistence error, which optimizes training dataset by excluding low‐utility samples while retaining essential physical features like zenith azimuth angles. This enables exclusion up to 30% original data, resulting in ≈16% savings computational resources without affecting forecast validated using test set 324 991 observations. achieves skill score 7.63%, significantly outperforming models, exhibit negative scores under conditions.
Язык: Английский
Процитировано
1Advances in Applied Energy, Год журнала: 2024, Номер 14, С. 100172 - 100172
Опубликована: Апрель 10, 2024
The variability of solar photovoltaic (PV) power output, driven by rapidly changing cloud dynamics, hinders the transition to reliable renewable energy systems. Information on future sky conditions, especially coverage, hold promise for improving PV output forecasting. Leveraging recent advances in generative artificial intelligence (AI), we introduce SkyGPT, a physics-constrained stochastic video prediction model, which predicts plausible images using historical images. We show that SkyGPT can accurately capture producing highly realistic and diverse further demonstrate its efficacy 15-minute-ahead probabilistic forecasting real-world generation data from 30-kW rooftop system. By coupling with U-Net-based observe superior reliability sharpness compared several benchmark methods. propose approach achieves continuous ranked probability score (CRPS) 2.81 kW, outperforming classic convolutional neural network (CNN) baseline 13% smart persistence model 23%. findings this research could aid efficient resilient management electricity generation, particularly as renewable-heavy grids. study also provides valuable insights into modeling broad community, encompassing fields such meteorology atmospheric sciences.
Язык: Английский
Процитировано
8IEEE Access, Год журнала: 2024, Номер 12, С. 168904 - 168924
Опубликована: Янв. 1, 2024
Язык: Английский
Процитировано
0