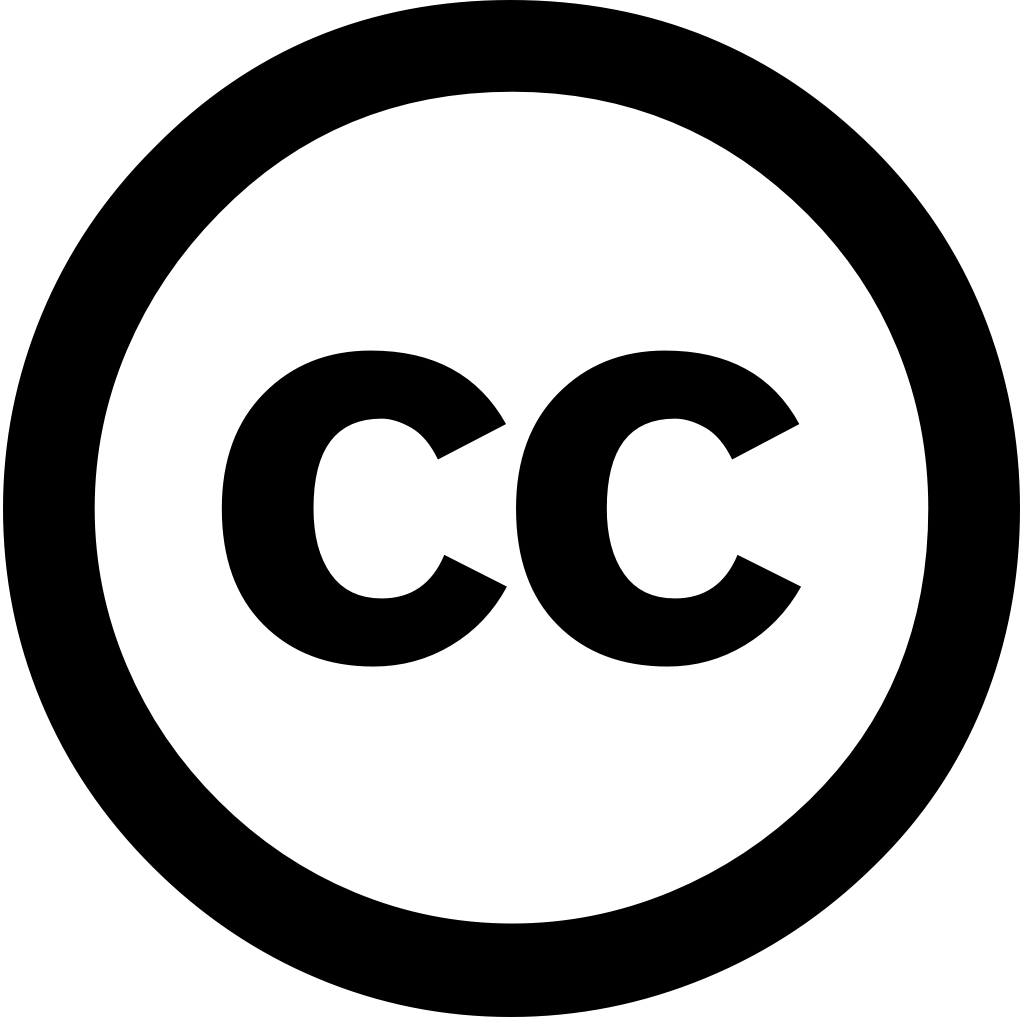
Energy and Buildings, Год журнала: 2024, Номер 307, С. 113964 - 113964
Опубликована: Фев. 2, 2024
Язык: Английский
Energy and Buildings, Год журнала: 2024, Номер 307, С. 113964 - 113964
Опубликована: Фев. 2, 2024
Язык: Английский
Energy and AI, Год журнала: 2023, Номер 14, С. 100306 - 100306
Опубликована: Окт. 1, 2023
The quickening propensity of growth within the areas information and communications technology energy networks has triggered emergence a central idea termed as Internet Energy (IoE). Such concept relates internet things, which is term used in describing usage sophisticated digital control devices with capability transmitting data via IT systems. an interconnected network being formed upcoming days through upgrades field intelligent systems to real-time optimization management. industry had sustained expansion, reaching IoE milestone continuing this cutting-edge power system, next generation IoE. A directional pathway from traditional system been conducted research work by addressing importance integrating smart transmission communication infrastructure, metering, pricing management scheme. detailed investigation respect technical angles, such architecture, on supply & demand side, protocol based fundamental elements essential technologies carried out indicate blueprint jurisdiction complexity. integration, security challenges may deviate performance that focused proper issues solutions. Finally, framework establish next-generation constructed future scopes insure higher resiliency, cyber-security stability.
Язык: Английский
Процитировано
10Energy and Buildings, Год журнала: 2023, Номер 301, С. 113738 - 113738
Опубликована: Ноя. 10, 2023
Язык: Английский
Процитировано
10Engineering Applications of Artificial Intelligence, Год журнала: 2023, Номер 128, С. 107568 - 107568
Опубликована: Ноя. 26, 2023
Язык: Английский
Процитировано
10Heliyon, Год журнала: 2024, Номер 10(4), С. e25848 - e25848
Опубликована: Фев. 1, 2024
The assessment of energy performance in smart buildings has emerged as a prominent area research driven by the increasing consumption trends worldwide. Analyzing attributes using optimized machine learning models been highly effective approach for estimating cooling load (CL) and heating (HL) buildings. In this study, an artificial neural network (ANN) is used basic predictor that undergoes optimization five metaheuristic algorithms, namely coati algorithm (COA), gazelle (GOA), incomprehensible but intelligible-in-time logics (IbIL), osprey (OOA), sooty tern (STOA) to predict CL HL residential building. are trained tested via Energy Efficiency dataset (downloaded from UCI Repository). A score-based ranking system built upon three accuracy evaluators including mean absolute percentage error (MAPE), root square (RMSE), percentage-Pearson correlation coefficient (PPCC) compare prediction models. Referring results, all demonstrated high (e.g., PPCCs >89%) predicting both HL. However, calculated final scores (43, 20, 39, 38, 10 36, 42, STOA, OOA, IbIL, GOA, COA, respectively) indicated STOA perform better than COA OOA. Moreover, comparison with various algorithms earlier literature showed provide more accurate solution. Therefore, use ANN these recommended practical early forecast optimizing design systems.
Язык: Английский
Процитировано
3Energy and Buildings, Год журнала: 2024, Номер 307, С. 113964 - 113964
Опубликована: Фев. 2, 2024
Язык: Английский
Процитировано
3