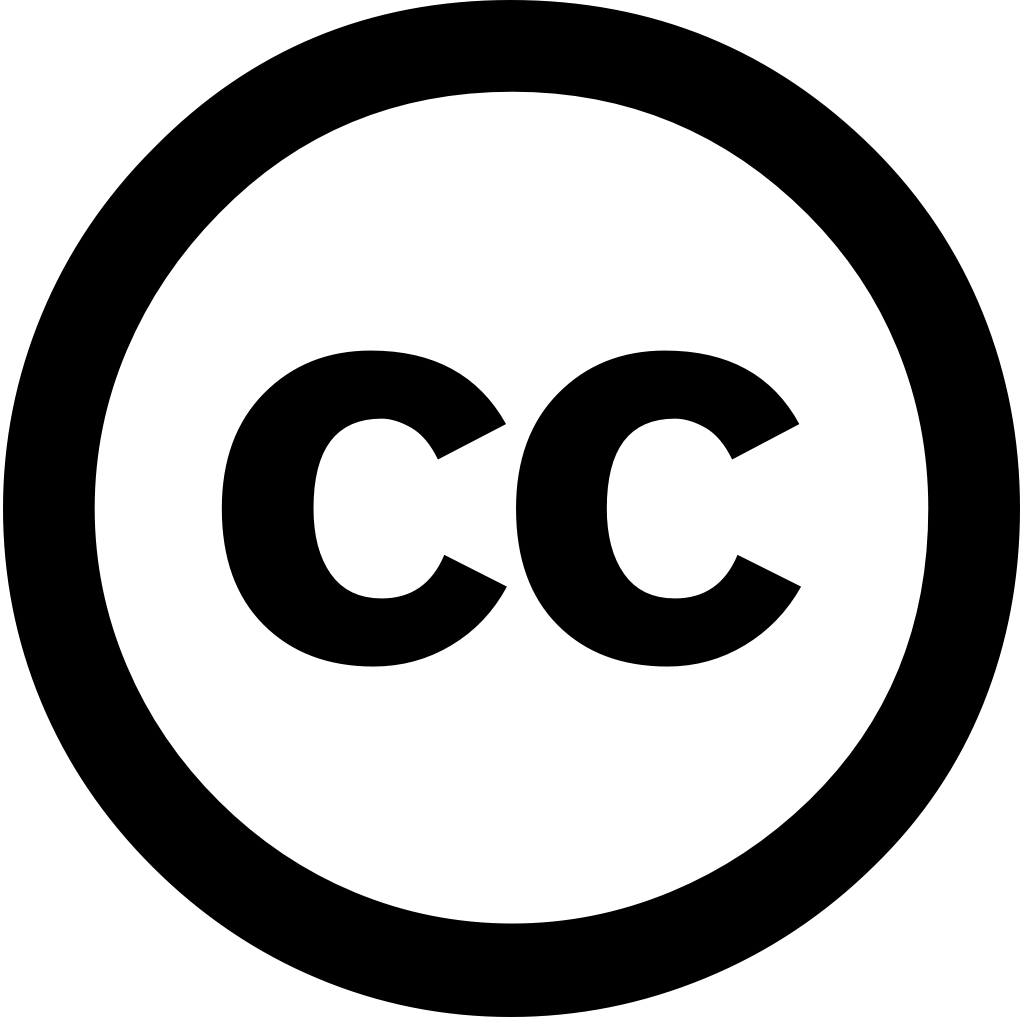
Results in Engineering, Год журнала: 2024, Номер 24, С. 103369 - 103369
Опубликована: Ноя. 8, 2024
Язык: Английский
Results in Engineering, Год журнала: 2024, Номер 24, С. 103369 - 103369
Опубликована: Ноя. 8, 2024
Язык: Английский
Knowledge-Based Systems, Год журнала: 2024, Номер 295, С. 111850 - 111850
Опубликована: Апрель 22, 2024
Язык: Английский
Процитировано
35The Journal of Supercomputing, Год журнала: 2024, Номер 80(15), С. 22913 - 23017
Опубликована: Июль 1, 2024
Язык: Английский
Процитировано
34Scientific Reports, Год журнала: 2024, Номер 14(1)
Опубликована: Март 5, 2024
Abstract This study presents the K-means clustering-based grey wolf optimizer, a new algorithm intended to improve optimization capabilities of conventional optimizer in order address problem data clustering. The process that groups similar items within dataset into non-overlapping groups. Grey hunting behaviour served as model for however, it frequently lacks exploration and exploitation are essential efficient work mainly focuses on enhancing using weight factor concepts increase variety avoid premature convergence. Using partitional clustering-inspired fitness function, was extensively evaluated ten numerical functions multiple real-world datasets with varying levels complexity dimensionality. methodology is based incorporating concept purpose refining initial solutions adding diversity during phase. results show performs much better than standard discovering optimal clustering solutions, indicating higher capacity effective solution space. found able produce high-quality cluster centres fewer iterations, demonstrating its efficacy efficiency various datasets. Finally, demonstrates robustness dependability resolving issues, which represents significant advancement over techniques. In addition addressing shortcomings algorithm, incorporation innovative establishes further metaheuristic algorithms. performance around 34% original both test problems problems.
Язык: Английский
Процитировано
29Scientific Reports, Год журнала: 2024, Номер 14(1)
Опубликована: Фев. 2, 2024
Abstract The advancement of Photovoltaic (PV) systems hinges on the precise optimization their parameters. Among numerous techniques, effectiveness each often rests inherent This research introduces a new methodology, Reinforcement Learning-based Golden Jackal Optimizer (RL-GJO). approach uniquely combines reinforcement learning with to enhance its efficiency and adaptability in handling various problems. Furthermore, incorporates an advanced non-linear hunting strategy optimize algorithm’s performance. proposed algorithm is first validated using 29 CEC2017 benchmark test functions five engineering-constrained design Secondly, rigorous testing PV parameter estimation datasets, including single-diode model, double-diode three-diode representative module, was carried out highlight superiority RL-GJO. results were compelling: root mean square error values achieved by RL-GJO markedly lower than those original other prevalent methods. synergy between GJO this facilitates faster convergence improved solution quality. integration not only improves performance metrics but also ensures more efficient process, especially complex scenarios. With average Freidman’s rank 1.564 for numerical engineering problems 1.742 problems, performing better peers. stands as reliable tool estimation. By seamlessly combining golden jackal optimizer, it sets optimization, indicating promising avenue future applications.
Язык: Английский
Процитировано
23IEEE Access, Год журнала: 2024, Номер 12, С. 45879 - 45903
Опубликована: Янв. 1, 2024
This paper introduces a robust approach, integrating Virtual Inertia Controller (VIC) with modified demand response controller for an islanded Multi-Microgrid (MMG) system, accommodating high levels of Renewable Energy Sources (RESs). In these MGs, the low inertia in system has undesirable impact on stability MG frequency. As result, it leads to weakening MGs overall performance. A novel fractional derivative virtual is integrated into VIC loop address this issue. enhancement aims fortify MG's and performance, particularly when facing contingencies. Furthermore, been incorporated proposed control technique mitigate frequency fluctuations reduce stress energy storage (ESS). Fractional Order Proportional Integral Derivative (FOPID) controllers have employed regulate active power output biodiesel generators Geothermal station MG. The hybrid sparrow search mountain gazelle optimizer algorithm (SSAMGO) optimizes parameters three-loop controller. Time-domain simulations assess effectiveness enhancing stability. SSAMGO's performance was comprehensively evaluated, comparing various optimization algorithms diverse scenarios. results obtained from MMG demonstrate that utilizing technique, optimized SSAMGO parameters, yields notable improvements settling time by 24.68%, 46.20%, 7.52%, 61.01%, steady-state error values 72.56%, 98.18%, 98.73%, 6.67%, undershoot 105.76%, 144.23%, 19.23%, 7.69% compared other state-of-the-art presented literature. Finally, technique's robustness are assessed comparison conventional across These scenarios encompass random load fluctuations, real-time changes RES, wide spectrum operations, including situations reduced damping variation.
Язык: Английский
Процитировано
16Results in Engineering, Год журнала: 2025, Номер 25, С. 103933 - 103933
Опубликована: Янв. 5, 2025
Язык: Английский
Процитировано
6Computer Methods in Applied Mechanics and Engineering, Год журнала: 2025, Номер 436, С. 117718 - 117718
Опубликована: Янв. 9, 2025
Язык: Английский
Процитировано
3Results in Engineering, Год журнала: 2025, Номер unknown, С. 103822 - 103822
Опубликована: Янв. 1, 2025
Язык: Английский
Процитировано
2Water, Год журнала: 2025, Номер 17(4), С. 552 - 552
Опубликована: Фев. 14, 2025
The precise acquisition of water depth data in nearshore shallow waters bears considerable strategic significance for marine environmental monitoring, resource stewardship, navigational infrastructure development, and military security. Conventional bathymetric survey methodologies are constrained by their spatial temporal limitations, thus failing to satisfy the requirements large-scale, real-time surveillance. While satellite remote sensing technologies present a novel approach inversion waters, attaining high-precision areas characterized elevated levels suspended sediments diminished transparency remains formidable challenge. To tackle this issue, study introduces an enhanced XGBoost model grounded Newton–Raphson optimizer (NRBO–XGBoost) successfully applies it investigations Beibu Gulf. research amalgamates Sentinel-2B multispectral imagery, nautical chart data, situ measurements. By ingeniously integrating with framework, realizes automatic configuration training parameters, markedly elevating accuracy. findings reveal that NRBO–XGBoost attains coefficient determination (R2) 0.85 when compared alongside scatter index (SI) 21%, substantially surpassing conventional models. Additional validation analyses indicate achieves 0.86 field-measured mean absolute error (MAE) 1.60 m, root square (RMSE) 2.13 13%. Moreover, exhibits exceptional performance extended applications within Zhanjiang Port (R2 = 0.90), unequivocally affirming its dependability practicality intricate environments. This not only provides fresh solution remotely complex settings but also imparts valuable technical insights into associated underwater surveys exploitation.
Язык: Английский
Процитировано
2Applied Energy, Год журнала: 2025, Номер 385, С. 125539 - 125539
Опубликована: Фев. 17, 2025
Язык: Английский
Процитировано
2