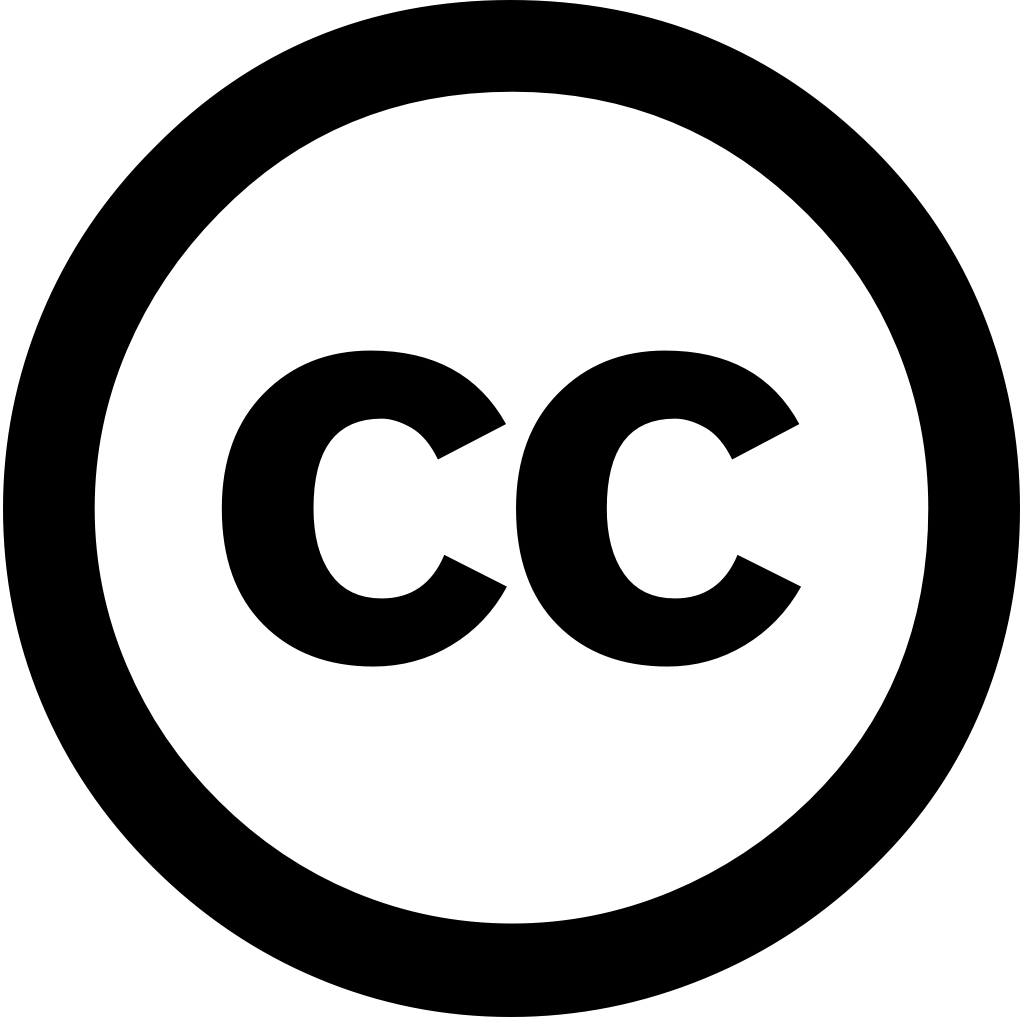
Applied Mathematical Modelling, Год журнала: 2024, Номер 138, С. 115738 - 115738
Опубликована: Окт. 11, 2024
Язык: Английский
Applied Mathematical Modelling, Год журнала: 2024, Номер 138, С. 115738 - 115738
Опубликована: Окт. 11, 2024
Язык: Английский
Thin-Walled Structures, Год журнала: 2024, Номер 205, С. 112495 - 112495
Опубликована: Сен. 24, 2024
Язык: Английский
Процитировано
30Structures, Год журнала: 2025, Номер 71, С. 108167 - 108167
Опубликована: Янв. 1, 2025
Язык: Английский
Процитировано
1Reliability Engineering & System Safety, Год журнала: 2025, Номер unknown, С. 110819 - 110819
Опубликована: Янв. 1, 2025
Язык: Английский
Процитировано
1Structures, Год журнала: 2025, Номер 75, С. 108797 - 108797
Опубликована: Апрель 8, 2025
Язык: Английский
Процитировано
1Scientific Reports, Год журнала: 2025, Номер 15(1)
Опубликована: Март 18, 2025
Topology optimization is a critical tool for modern structural design, yet existing methods often prioritize single objectives (e.g., compliance minimization) and suffer from prohibitive computational costs, especially in multi-objective scenarios. To address these limitations, this paper introduces novel two-stage topology (MOTO) method that uniquely integrates data-driven learning with physics-informed refinement, both stages are implemented within nearly identical network frameworks, ensuring simplicity consistency execution. Firstly, MOTO mathematical model based on the constraint programming considers competing of compliance, stress distribution, material usage was constructed. Secondly, neural incorporates shifted windows attention mechanism lightweight modules developed to enhance feature extraction while maintaining efficiency. Finally, proposed trained two stages: In Stage-1, utilizing adaptive input tensors, predicts near-optimal geometries across variable design domains (including rectangular L-shaped configurations) diverse boundary conditions real time, requiring only 1,650 samples per condition. Stage-2, structures Stage-1 were physically optimized achieve optimal performance. Experimental results demonstrate method's capability generate high-accuracy, computationally efficient solutions robust generalization capabilities. It effectively tackles challenges associated multi-scale non-convex geometries, various even untrained significantly reducing data dependency, advancement optimization. The approach offers new insights promotes advancements practices.
Язык: Английский
Процитировано
0Applied Mathematical Modelling, Год журнала: 2024, Номер 138, С. 115738 - 115738
Опубликована: Окт. 11, 2024
Язык: Английский
Процитировано
1