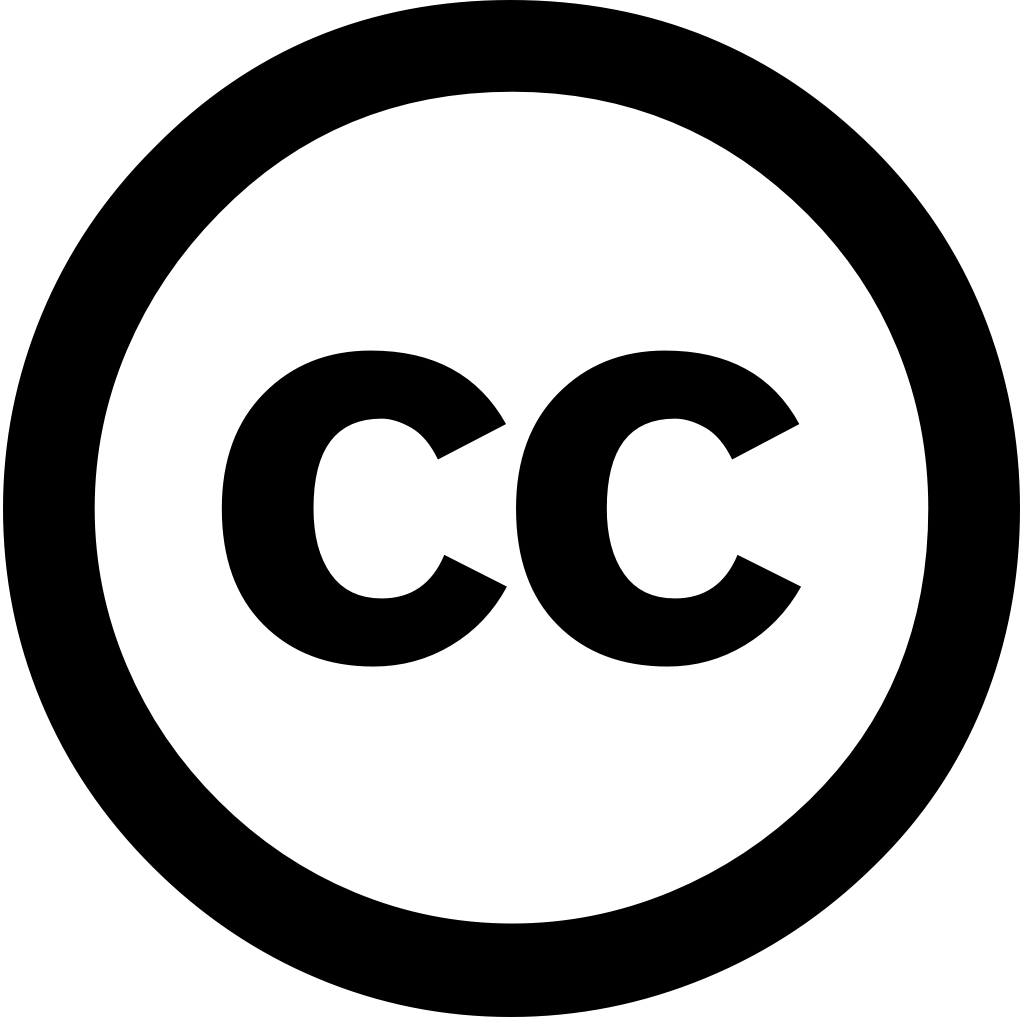
Research Square (Research Square), Год журнала: 2024, Номер unknown
Опубликована: Дек. 13, 2024
Язык: Английский
Research Square (Research Square), Год журнала: 2024, Номер unknown
Опубликована: Дек. 13, 2024
Язык: Английский
Expert Systems with Applications, Год журнала: 2025, Номер 269, С. 126391 - 126391
Опубликована: Янв. 10, 2025
Язык: Английский
Процитировано
0Neural Networks, Год журнала: 2025, Номер 185, С. 107148 - 107148
Опубликована: Янв. 16, 2025
Язык: Английский
Процитировано
0Signal Image and Video Processing, Год журнала: 2025, Номер 19(3)
Опубликована: Янв. 28, 2025
Язык: Английский
Процитировано
0Lecture notes in computer science, Год журнала: 2025, Номер unknown, С. 36 - 53
Опубликована: Янв. 1, 2025
Язык: Английский
Процитировано
0PeerJ Computer Science, Год журнала: 2025, Номер 11, С. e2668 - e2668
Опубликована: Фев. 14, 2025
The utilization of deep learning and invertible networks for image hiding has been proven effective secure. These methods can conceal large amounts information while maintaining high quality security. However, existing often lack precision in selecting the hidden regions primarily rely on residual structures. They also fail to fully exploit low-level features, such as edges textures. issues lead reduced model generation results, a heightened risk network overfitting, diminished generalization capability. In this article, we propose novel method based networks, called MFI-Net. introduces new upsampling convolution block (UCB) combines it with dense that employs parametric rectified linear unit (PReLU) activation function, effectively utilizing multi-level (low-level high-level features) image. Additionally, frequency domain loss (FDL) is introduced, which constrains secret be cover are more suitable concealing data. Extensive experiments DIV2K, COCO, ImageNet datasets demonstrate MFI-Net consistently outperforms state-of-the-art methods, achieving superior metrics. Furthermore, apply proposed digital collection images, significant success.
Язык: Английский
Процитировано
0Neurocomputing, Год журнала: 2025, Номер unknown, С. 130178 - 130178
Опубликована: Апрель 1, 2025
Язык: Английский
Процитировано
0Electronics, Год журнала: 2024, Номер 13(23), С. 4821 - 4821
Опубликована: Дек. 6, 2024
Image steganography involves hiding a secret message within an image for covert communication, allowing only the intended recipient to extract hidden from “stego” image. The can also be itself enable transmission of more information, resulting in applications where one is concealed another. While existing techniques embed similar size into cover with minimal distortion, they often overlook effects lossy compression during transmission, such as when saving images commonly used JPEG format. This oversight hinder extraction To address challenges posed by steganography, we propose Steganography Network (JSN) that leverages reversible deep neural network its backbone, integrated encoding process. We utilize 8×8 Discrete Cosine Transform (DCT) and consider quantization step specified create JPEG-compliant stego discuss various design considerations conduct extensive testing on JSN validate performance practicality real-world applications.
Язык: Английский
Процитировано
1Ingénierie des systèmes d information, Год журнала: 2024, Номер 29(4), С. 1653 - 1665
Опубликована: Авг. 21, 2024
In the modern era, numerous research studies consistently affirm superior performance of Convolutional Neural Networks (CNNs) over traditional machine learning methods in steganalysis, a technique used to detect hidden data through steganography.Deep Learning (DL), particularly CNNs, is powerful tool for steganalysis because it can handle large datasets effectively.Despite CNNs being widely various areas, previous have primarily focused on improving image classification (cover or stego), often neglecting thorough exploration experimental setup.This aims assess sensitivity CNN-based model by investigating impact different pooling layers state-of-the-art models.The experiments involve five recently proposed models.Significantly, choice goes beyond mere improvement; also addresses overfitting.The results reveal significant diversity based selected layers, namely maximum, average, and mixed pooling, emphasizing importance optimizing objectives when choosing particular approach.This highlights evolving nature this field study need careful consideration layer selection effective steganalysis.
Язык: Английский
Процитировано
0Engineering Applications of Artificial Intelligence, Год журнала: 2024, Номер 137, С. 109207 - 109207
Опубликована: Авг. 30, 2024
Процитировано
0Multimedia Tools and Applications, Год журнала: 2024, Номер unknown
Опубликована: Ноя. 13, 2024
Язык: Английский
Процитировано
0