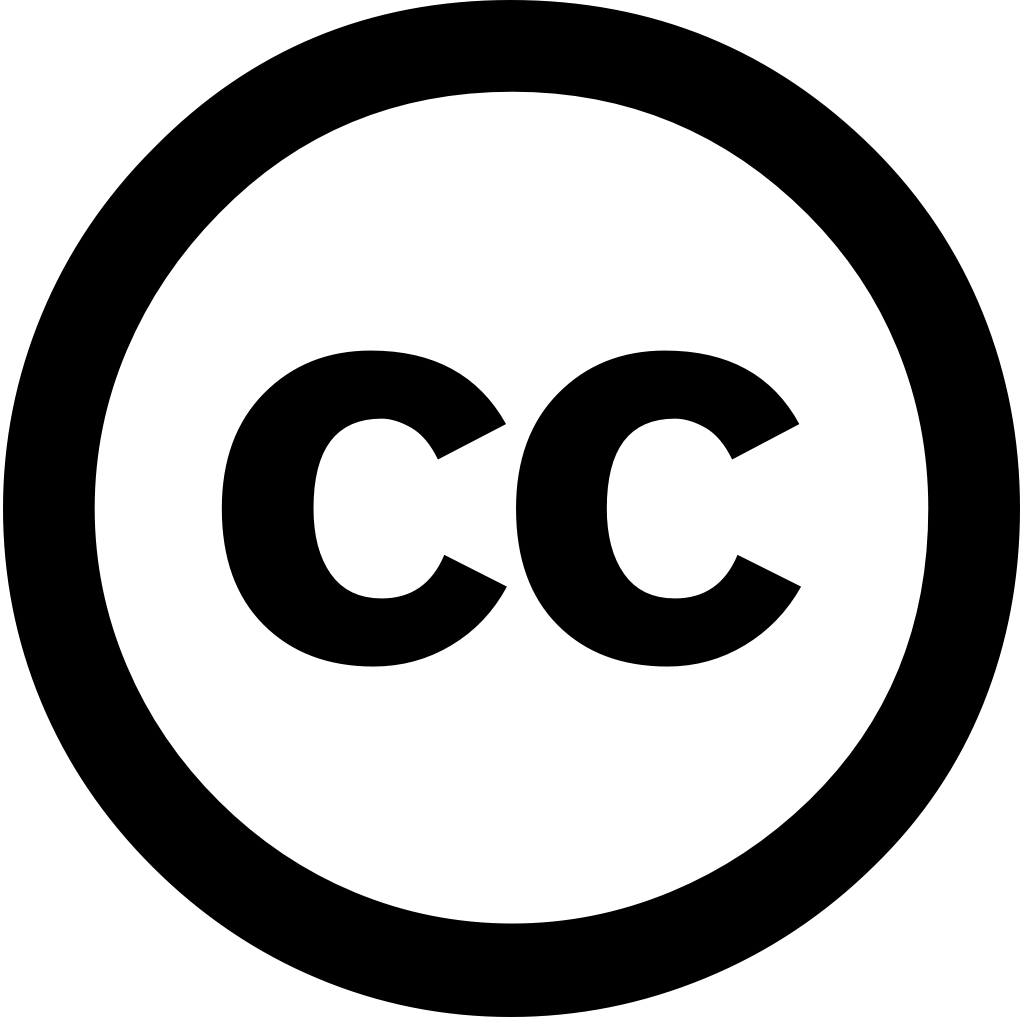
Alexandria Engineering Journal, Год журнала: 2024, Номер unknown
Опубликована: Дек. 1, 2024
Язык: Английский
Alexandria Engineering Journal, Год журнала: 2024, Номер unknown
Опубликована: Дек. 1, 2024
Язык: Английский
Scientific Reports, Год журнала: 2025, Номер 15(1)
Опубликована: Фев. 4, 2025
Wearable technologies enable continuous monitoring of various health metrics, such as physical activity, heart rate, sleep, and stress levels. A key challenge with wearable data is obtaining quality labels. Unlike modalities like video where the videos themselves can be effectively used to label objects or events, do not contain obvious cues about manifestation users usually require rich metadata. As a result, noise become an increasingly thorny issue when labeling data. In this paper, we propose novel solution address noisy learning, entitled Few-Shot Human-in-the-Loop Refinement (FHLR). Our method initially learns seed model using weak Next, it fine-tunes handful expert corrections. Finally, achieves better generalizability robustness by merging fine-tuned models via weighted parameter averaging. We evaluate our approach on four challenging tasks datasets, compare against eight competitive baselines designed deal show that FHLR significantly performance learning from labels state-of-the-art large margin, up $$19\%$$ accuracy improvement under symmetric asymmetric noise. Notably, find particularly robust increased noise, unlike prior works suffer severe degradation. work only generalization in high-stakes sensing benchmarks but also sheds light how affects commonly-used models.
Язык: Английский
Процитировано
1Electronics, Год журнала: 2025, Номер 14(6), С. 1213 - 1213
Опубликована: Март 19, 2025
Massive Online Open Courses (MOOCs) have been growing rapidly in the past few years. Video content is an important carrier for cultural exchange and education popularization, needs to be translated into multiple language versions meet of learners from different countries regions. However, current MOOC video processing solutions rely excessively on manual operations, resulting low efficiency difficulty meeting urgent requirement large-scale translation. Key technical challenges include accurate localization embedded text complex frames, maintaining style consistency across languages, preserving readability visual quality during Existing methods often struggle with handling diverse styles, background interference, language-specific typographic variations. In view this, this paper proposes innovative cross-language transfer algorithm that integrates advanced techniques such as attention mechanisms, latent space mapping, adaptive instance normalization. Specifically, first utilizes mechanisms accurately locate position each image, ensuring subsequent can targeted at specific areas. Subsequently, by extracting features corresponding location information, ensure matching styles features, achieving effective transfer. Additionally, introduces a new color loss function aimed colors before after transfer, further enhancing edited images. Through extensive experimental verification, proposed demonstrated excellent performance both synthetic real-world datasets. Compared existing methods, exhibited significant advantages image evaluation metrics, method achieved 2% improvement FID metric 20% IS relevant datasets compared SOTA methods. introduced dataset, PTTEXT, will made publicly available upon acceptance paper. For additional details, please refer project URL, which public has accepted.
Язык: Английский
Процитировано
1Entropy, Год журнала: 2024, Номер 26(7), С. 589 - 589
Опубликована: Июль 10, 2024
While collecting training data, even with the manual verification of experts from crowdsourcing platforms, eliminating incorrect annotations (noisy labels) completely is difficult and expensive. In dealing datasets that contain noisy labels, over-parameterized deep neural networks (DNNs) tend to overfit, leading poor generalization classification performance. As a result, label learning (NLL) has received significant attention in recent years. Existing research shows although DNNs eventually fit all they first prioritize fitting clean samples, then gradually overfit samples. Mainstream methods utilize this characteristic divide data but face two issues: class imbalance segmented subsets optimization conflict between unsupervised contrastive representation supervised learning. To address these issues, we propose Balanced Partitioning Training framework Pseudo-Label Relaxed loss called BPT-PLR, which includes crucial processes: balanced partitioning process two-dimensional Gaussian mixture model (BP-GMM) semi-supervised oversampling pseudo-label relaxed (SSO-PLR). The former utilizes both semantic feature information prediction results identify introducing balancing strategy maintain balance divided as much possible. latter adopts latest replace loss, reducing conflicts losses improve We validate effectiveness BPT-PLR on four benchmark NLL field: CIFAR-10/100, Animal-10N, Clothing1M. Extensive experiments comparing state-of-the-art demonstrate can achieve optimal or near-optimal
Язык: Английский
Процитировано
6Scientific Reports, Год журнала: 2024, Номер 14(1)
Опубликована: Окт. 28, 2024
Abstract Histopathological examination holds a crucial role in cancer grading and serves as significant reference for devising individualized patient treatment plans clinical practice. Nevertheless, the distinctive features of numerous histopathological image targets frequently contribute to suboptimal segmentation performance. In this paper, we propose UNet-based multi-scale context fusion algorithm medical segmentation, which extracts rich contextual information by extracting semantic at different encoding stages assigns weights scales through TBSFF module improve learning ability network features. Through feature selection networks, richer detailed are extracted. The target can be more accurately segmented without significantly increasing extra overhead. results demonstrate that our achieves superior Dice IoU scores with relatively small parameter count. Specifically, on GlaS dataset, score is 90.56, 83.47. For MoNuSeg 79.07, 65.98.
Язык: Английский
Процитировано
5Scientific Reports, Год журнала: 2024, Номер 14(1)
Опубликована: Дек. 5, 2024
Fire is a dangerous disaster that causes human, ecological, and financial ramifications. Forest fires have increased significantly in recent years due to natural artificial climatic factors. Therefore, accurate early prediction of essential. While significant advancements been made traditional Deep Learning (DL) methods for fire detection, challenges remain accurately pinpointing recognizing regions, especially diverse large environments, prevent damage effectively. To address these challenges, this paper introduces novel Federated (FL)-based method called Indoor-Outdoor FireNet (IOFireNet) detecting localizing regions. The proposed incorporates Bilateral Filter (BF) effectively preprocess images reduce noise artifacts enhance detection clarity. It employs Super Pixel-based Adaptive Clustering (SPAC) precisely segment non-fire A global IOFireNet model developed aggregate parameters from local models, improving accuracy across varied while MobileNet used efficient data processing, enabling predictions on spread, severity, affected areas support warnings. FL-based attains an rate 98.65% 97.14% mean IoU segmentation. SPAC reaches 4.06%, which 2.45% better than the graph cut algorithm CRF model. achieves 0.23%, 4.20%, 3.29%, 10.02%, VGG-19, ResNet-50, Inception, Dense Net, respectively.
Язык: Английский
Процитировано
5Knowledge-Based Systems, Год журнала: 2024, Номер 300, С. 112199 - 112199
Опубликована: Июль 2, 2024
Язык: Английский
Процитировано
4Complex & Intelligent Systems, Год журнала: 2025, Номер 11(3)
Опубликована: Фев. 12, 2025
Язык: Английский
Процитировано
0Journal of Intelligent Information Systems, Год журнала: 2025, Номер unknown
Опубликована: Апрель 3, 2025
Язык: Английский
Процитировано
0Sensors, Год журнала: 2025, Номер 25(8), С. 2613 - 2613
Опубликована: Апрель 20, 2025
Maintaining a Constant False Alarm Rate (CFAR) in the presence of K-distributed sea clutter is vital due to dynamic and unpredictable nature maritime environments. However, conventional CFAR detectors suffer significant performance degradation multi-target scenarios, primarily masking effect caused by interfering targets. To address this challenge, paper introduces an advanced detection scheme that integrates Linear Density-Based Spatial Clustering for Applications with Noise (Lin-DBSCAN) processing. Lin-DBSCAN specifically tailored efficiently identify isolate targets spikes, which typically manifest as outliers symmetric reference windows surrounding Cell Under Test (CUT). By leveraging Lin-DBSCAN, proposed Lin-DBSCAN-CFAR method effectively filters out anomalous signals from background clutter, resulting enhanced accuracy robustness, especially under complex conditions. Extensive simulations varying conditions, including multiple target environments, false alarm rates, different shape parameters, demonstrate significantly outperforms approaches. It noteworthy achieves comparable more computationally intensive DBSCAN-CFAR while reducing computational complexity. Simulation results reveal requires 1 2 dB lower SNR reach probability 0.8 compared nearest traditional techniques, confirming its superiority both efficiency.
Язык: Английский
Процитировано
0Scientific Reports, Год журнала: 2024, Номер 14(1)
Опубликована: Окт. 13, 2024
Abstract Missing pixel imputation presents a critical challenge in image processing and computer vision, particularly applications such as restoration inpainting. The primary objective of this paper is to accurately estimate reconstruct missing values restore complete visual information. This introduces novel model called the Enhanced Connected Pixel Identity GAN with Neutrosophic (ECP-IGANN), which designed address two fundamental issues inherent existing architectures for generation: (1) mode collapse, leads lack diversity generated pixels, (2) preservation integrity within reconstructed images. ECP-IGANN incorporates key innovations improve imputation. First, an identity block integrated into generation process facilitate retention ensure consistency. Second, calculates 8-connected neighbouring pixels around each pixel, thereby enhancing coherence imputed pixels. efficacy was rigorously evaluated through extensive experimentation across five diverse datasets: BigGAN-ImageNet, 2024 Medical Imaging Challenge Dataset, Autonomous Vehicles Satellite Imagery Fashion Apparel Dataset 2024. These experiments assessed model’s performance terms diversity, accuracy, collapse mitigation, results demonstrating significant improvements Inception Score (IS) Fréchet Distance (FID). markedly enhanced segmentation validation phase all datasets. Key metrics, Dice Score, Accuracy, Precision, Recall, were improved substantially various models, including Spatial Attention U-Net, Dense Residual U-Net. For example, U-Net’s increased from 0.84 0.90, while accuracy 0.88 0.93 following application ECP-IGANN. Similar enhancements observed other datasets, highlighting robust generalizability imaging domains.
Язык: Английский
Процитировано
3