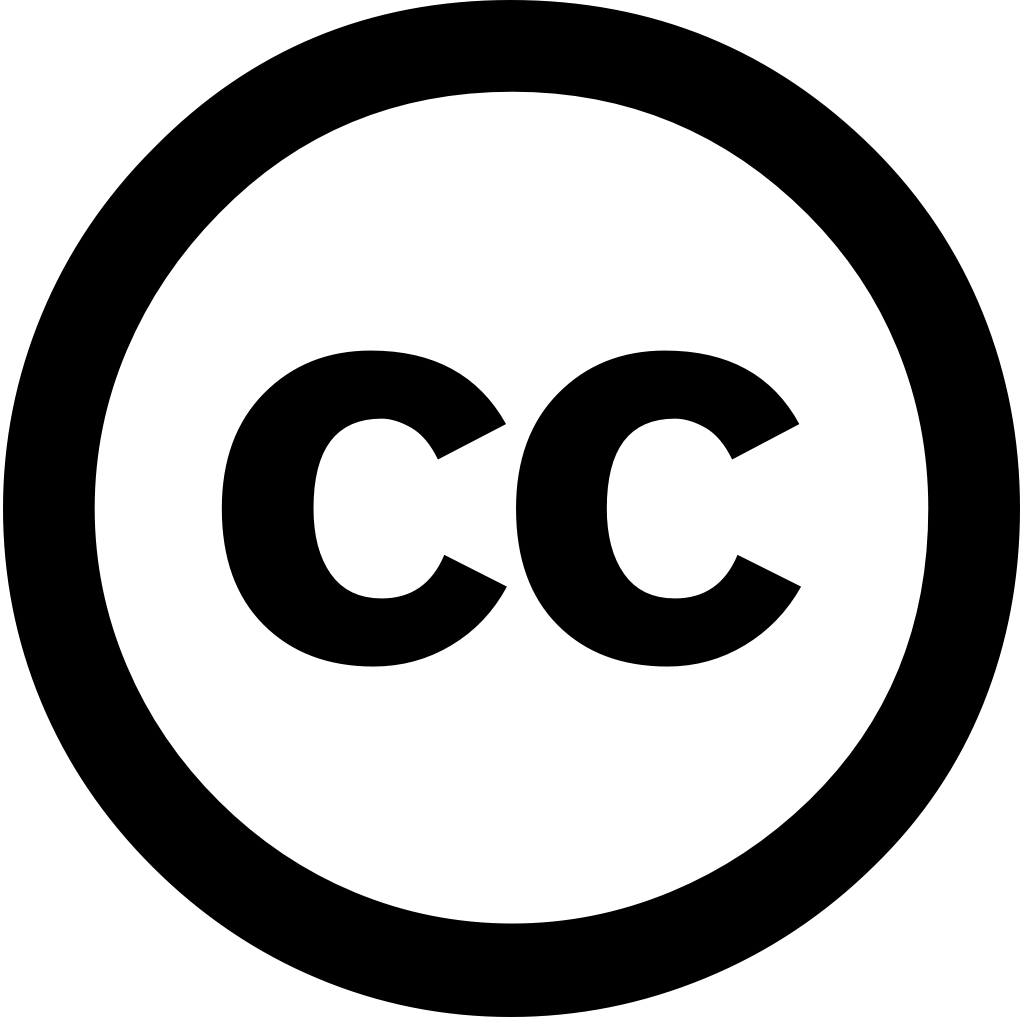
International Journal of Global Economics and Management, Год журнала: 2024, Номер 5(1), С. 215 - 227
Опубликована: Ноя. 11, 2024
As capital markets evolve, corporate financial misconduct garners more scrutiny. This study, using data from China's A-share companies (2006-2023), develops a model to predict irregularities with the random forest algorithm and SHAP value analysis. It analyzes influence of governance executive party traits on non-compliance their predictive roles. Findings indicate that characteristics significantly impact predictions, while have lower influence. The model's AUC improves inclusion characteristics. analysis highlights feature importance direction. results offer practical insights for regulators, companies, investors, aiding regulatory efficiency, optimization, investment decisions, guide strategies market health.
Язык: Английский