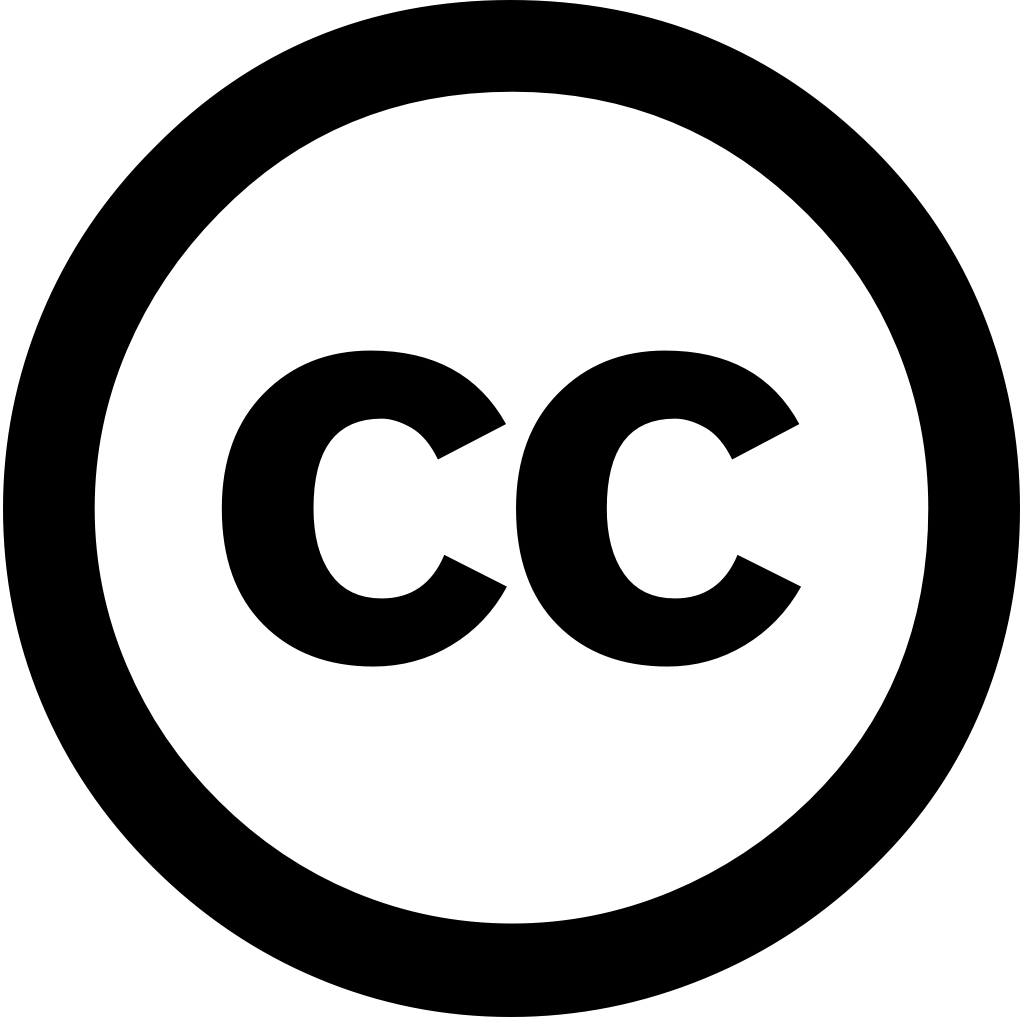
Groundwater for Sustainable Development, Год журнала: 2024, Номер 28, С. 101389 - 101389
Опубликована: Дек. 3, 2024
Язык: Английский
Groundwater for Sustainable Development, Год журнала: 2024, Номер 28, С. 101389 - 101389
Опубликована: Дек. 3, 2024
Язык: Английский
Water Resources Research, Год журнала: 2024, Номер 60(7)
Опубликована: Июль 1, 2024
Abstract GRACE (Gravity Recovery and Climate Experiment) has been widely used to evaluate terrestrial water storage (TWS) groundwater (GWS). However, the coarse‐resolution of data limited ability identify local vulnerabilities in changes associated with climatic anthropogenic stressors. This study employs high‐resolution (1 km 2 ) generated through machine learning (ML) based statistical downscaling illuminate TWS GWS dynamics across twenty sub‐regions Indus Basin. Monthly anomalies obtained from a geographically weighted random forest (RF gw model maintained good consistency original at 25 grid scale. The downscaled 1 resolution illustrate spatial heterogeneity depletion within each sub‐region. Comparison in‐situ 2,200 monitoring wells shows that significantly improves agreement data, evidenced by higher Kling‐Gupta Efficiency (0.50–0.85) correlation coefficients (0.60–0.95). Hotspots highest decline rate between 2002 2023 were Dehli Doab (−442, −585 mm/year), BIST (−367, −556 Rajasthan (−242, −381 BARI (−188, −333 mm/year). Based on general additive model, 47%–83% was stressors mainly due increasing trends crop sown area, consumption, human settlements. lower (i.e., −25 −75 mm/year) upstream (e.g., Yogo, Gilgit, Khurmong, Kabul) where factors (downward shortwave radiations, air temperature, sea surface temperature) explained 72%–91% TWS/GWS changes. relative influences varied sub‐regions, underscoring complex interplay natural‐human activities basin. These findings inform place‐based resource management Basin advancing understanding vulnerabilities.
Язык: Английский
Процитировано
12Earth Science Informatics, Год журнала: 2025, Номер 18(1)
Опубликована: Янв. 1, 2025
Язык: Английский
Процитировано
0Hydrological Processes, Год журнала: 2025, Номер 39(2)
Опубликована: Фев. 1, 2025
ABSTRACT Climate change significantly impacts natural hydrological systems worldwide, affecting water availability and sedimentation dynamics. The upper Indus Basin is one of the most crucial basins in South Asia, which undergoing severe climatic variations, resulting extreme flooding. This study examines impact climate on cycle, availability, dynamics Shyok River basin Karakoram region. focuses investigating increasing outflows a seasonal basis, as found previous studies, by utilising daily river discharge data between 2003 2014 at Yugo hydrographic station operated WAPDA with geographic information (GIS), SWAT+ (Soil Water Assessment Tool), remote sensing, statistical techniques. To analyse variables, using only available ground weather stations inside basin, we have utilised ERA 5 reanalysis dataset to evaluate precipitation temperature trends. significance ERA5‐derived variables has been assessed Mann‐Kendall test, Sen's slope analysis Coefficient Determination R 2 from 2000 2020 monthly basis. Analysis reveals decline flow July October general upward trend during last 20 years, such significant decrease streamflow simulated trend, was 293 m 3 /s 2005 dropped 158 2017. In contrast, maximum actual vs. decreased 10 2010 2019, respectively. increased approximately 1.5°C. Seasonal an winter, while other seasons shown fluctuating Where trends were be non‐significant, p > 0.05. An sediment load model output suggests active erosion channel rate 40 megatons/ha. highlights It attempts contribute existing literature evaluation climate‐induced changes morphology. also necessity continuous scale.
Язык: Английский
Процитировано
0Water Resources Research, Год журнала: 2025, Номер 61(2)
Опубликована: Фев. 1, 2025
Abstract Hydrological Models face limitations in simulating the water cycle due to deficiencies process representation and such problems also weaken their forecasting skills. Here, we use Machine Learning (ML) forecast Gravity Recovery Climate Experiment (GRACE) derived total storage anomaly (TWSA) up 1 year ahead over Europe with near real‐time meteorological observations as predictors. Subsequently, assimilate forecasted GRACE TWSA into Community Land Model (CLM) enhance its performance both reanalysis forecast. As found five hindcast experiments, ML for following fits quite well actual Europe, an average correlation of 0.91, 0.92, 0.94 Iberian peninsula, Danube, Volga basins. Validation by data suggests that assimilating can improve CLM's capacity not only hydrological states but droughts. Additionally, is a viable alternative terms enhancing on seasonal annual scales through Data assimilation (DA). We highlight contribution DA generating CLM based overcomes purely model‐based TWSA. This study drought or resource services might consider integrate would benefit from constraining models ML‐forecasted At shorter timescales, forecasts could be useful quick‐look analysis processing suggested upcoming satellite gravity missions.
Язык: Английский
Процитировано
0Journal of Hydrology Regional Studies, Год журнала: 2025, Номер 58, С. 102277 - 102277
Опубликована: Март 8, 2025
Язык: Английский
Процитировано
0Journal of Environmental Management, Год журнала: 2025, Номер 381, С. 125215 - 125215
Опубликована: Апрель 9, 2025
Язык: Английский
Процитировано
0ENVIRONMENTAL SYSTEMS RESEARCH, Год журнала: 2024, Номер 13(1)
Опубликована: Сен. 3, 2024
The continued threat from climate change and human impacts on water resources demands high-resolution continuous hydrological data accessibility for predicting trends availability. This study proposes a novel threefold downscaling method based machine learning (ML) which integrates: normalization; interaction of hydrometeorological variables; the application time series split cross-validation that produces high spatial resolution groundwater storage anomaly (GWSA) dataset Gravity Recovery Climate Experiment (GRACE) its successor mission, GRACE Follow-On (GRACE-FO). In study, relationship between terrestrial (TWSA) other land surface variables (e.g., vegetation coverage, temperature, precipitation, in situ level data) is leveraged to downscale GWSA. predicted downscaled GWSA datasets were tested using monthly observations, results showed model satisfactorily reproduced temporal variations area, with Nash-Sutcliffe efficiency (NSE) correlation coefficient values 0.8674 (random forest) 0.7909 (XGBoost), respectively. Evapotranspiration was most influential predictor variable random forest model, whereas it rainfall XGBoost model. particular, excelled aligning closely observed patterns, as evidenced by positive correlations lower error metrics (Mean Absolute Error (MAE) 54.78 mm; R-squared (R²) 0.8674). 5 km (based decreasing trend associated variability pattern. An increase drought severity during El Niño lengthened full recovery historical trends. Furthermore, lag occurrence precipitation recharge likely controlled intensity characteristics aquifer. Projected increases could further times response droughts changing climate, resetting new tipping condition. Therefore, adaptation strategies must recognise less will be available supplement supply droughts.
Язык: Английский
Процитировано
2The Science of The Total Environment, Год журнала: 2024, Номер 953, С. 176024 - 176024
Опубликована: Сен. 4, 2024
Язык: Английский
Процитировано
1Groundwater for Sustainable Development, Год журнала: 2024, Номер 25, С. 101171 - 101171
Опубликована: Апрель 3, 2024
Язык: Английский
Процитировано
0Hydrology, Год журнала: 2024, Номер 11(11), С. 186 - 186
Опубликована: Ноя. 1, 2024
In situ groundwater monitoring is critical for irrigated agroecosystems and informs land cover changes. Yet, such data can pose management challenges confound agroecological relationships. Correspondingly, satellite-based approaches, including the GRACE-constellation, are increasing. Although in GRACE-derived comparisons occur, limited research considers dependencies. Herein, we examined differences approaches (observed [in situ, O] vs. predicted [GRACE-derived, P]) within Yazoo–Mississippi Delta (YMD), an agroecosystem southeastern USA. We compared variations modeled hydrology, cover, irrigation dynamics of YMD upper-quartile (UQ) area interest (AOI) (highest levels) lower-quartile (LQ) AOI (lowest every year from 2008 to 2020. Spatially, OUQ PUQ were northern portions YMD, with OLQ PLQ southern portions. Groundwater levels between OUQ:PUQ OLQ:PLQ each had correlations > 0.85. Regarding most categories varied ±2.50% model estimates over time. Relatedly, documented 14 instances where use category level inverted across models (OLQ:PLQ (5), OUQ:OLQ (6), PUQ:PLQ (3)). Irrigation results not statistically different among all models. Overall, our highlight importance quantifying incongruences management.
Язык: Английский
Процитировано
0