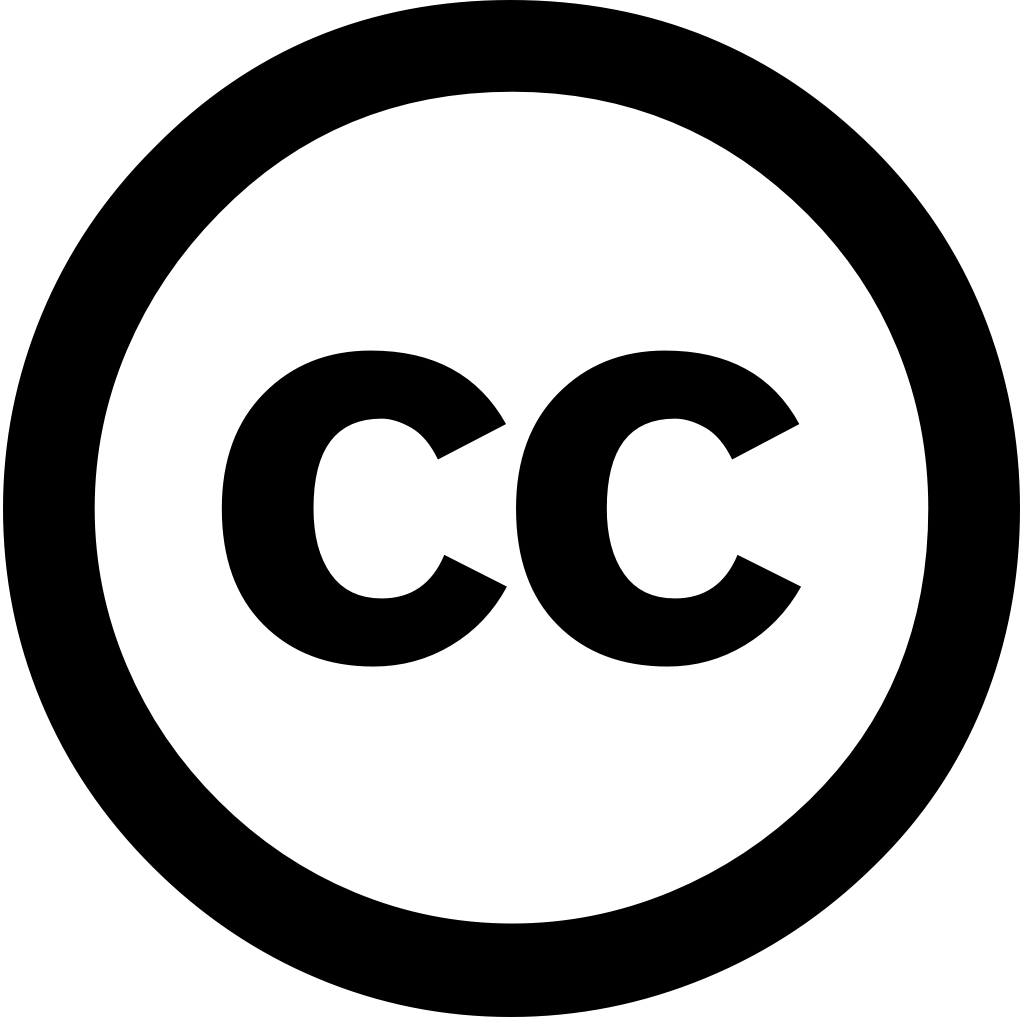
Energies, Год журнала: 2025, Номер 18(5), С. 1200 - 1200
Опубликована: Фев. 28, 2025
Artificial intelligence (AI), particularly supervised machine learning, has revolutionized the biofuel industry by enhancing feedstock selection, predicting fluid compositions, optimizing operations, and streamlining decision-making. These algorithms outperform traditional models accurately handling complex, high-dimensional data more efficiently cost-effectively. This study assesses effectiveness of various learning in engineering, focusing on a comparative analysis artificial neural networks (ANNs), support vector machines (SVMs), tree-based models, regularized regression models. The results show that random forest (RF) excel syngas composition its lower heating value (LHV), achieving high precision with training testing RMSE values below 0.2 R-squared close to 1. A detailed SHAP identified steam-to-biomass ratio (SBR) as most critical factor these predictions while also noting significant impact temperature conditions. underscores importance thermal parameters gasification supports systematic integration AI production enhance predictive accuracy.
Язык: Английский