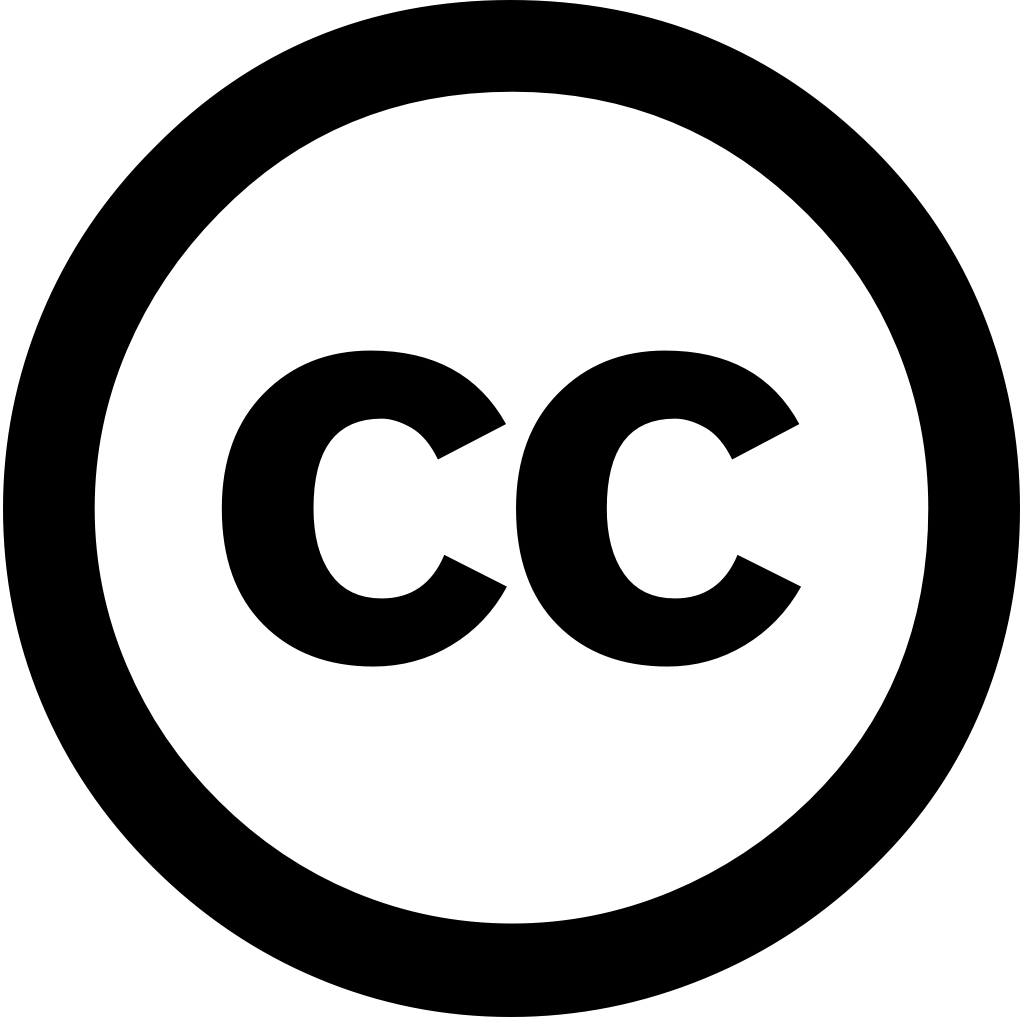
Big Data and Cognitive Computing, Год журнала: 2025, Номер 9(4), С. 82 - 82
Опубликована: Март 31, 2025
Traditional image denoising algorithms often struggle with real-world complexities such as spatially correlated noise, varying illumination conditions, sensor-specific noise patterns, motion blur, and structural distortions. This paper presents an enhanced residual network, R-REDNet, which stands for Reinforced Residual Encoder–Decoder Network. The proposed architecture incorporates deeper convolutional layers in the encoder replaces additive skip connections averaging operations to improve feature extraction suppression. Additionally, method leverages iterative refinement approach, further enhancing its performance. Experiments conducted on two noisy datasets demonstrate that R-REDNet outperforms current state-of-the-art approaches. Specifically, it attained a peak signal-to-noise ratio of 44.01 dB similarity index 0.9931 Dataset 1, obtained 46.15 0.9955 2. These findings confirm efficiency our delivering high-quality restoration while preserving fine details.
Язык: Английский