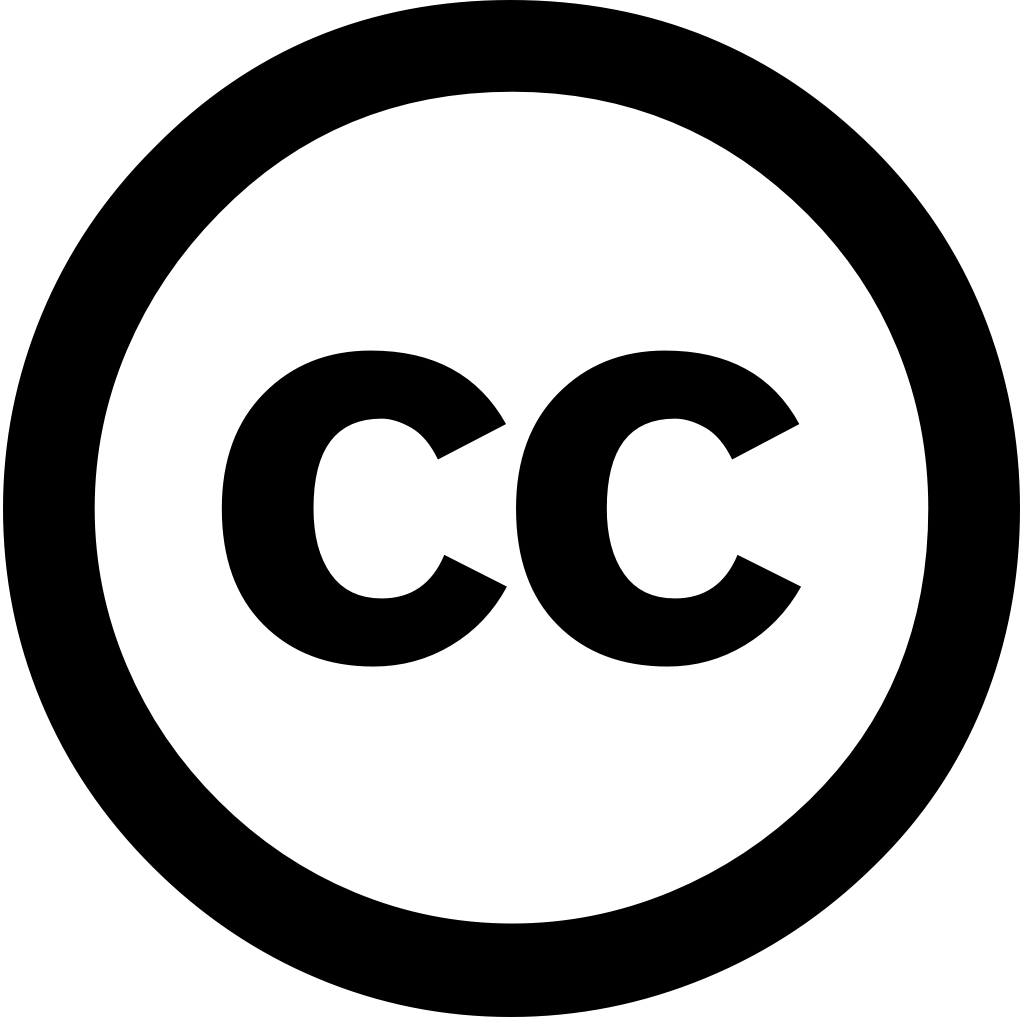
Internet of Things, Год журнала: 2024, Номер unknown, С. 101456 - 101456
Опубликована: Ноя. 1, 2024
Язык: Английский
Internet of Things, Год журнала: 2024, Номер unknown, С. 101456 - 101456
Опубликована: Ноя. 1, 2024
Язык: Английский
Computerized Medical Imaging and Graphics, Год журнала: 2025, Номер 120, С. 102493 - 102493
Опубликована: Янв. 20, 2025
Язык: Английский
Процитировано
0Internet of Things, Год журнала: 2025, Номер unknown, С. 101598 - 101598
Опубликована: Апрель 1, 2025
Язык: Английский
Процитировано
0Mehran University Research Journal of Engineering and Technology, Год журнала: 2025, Номер 44(2), С. 1 - 12
Опубликована: Апрель 9, 2025
Thyroid diseases, including hypothyroidism, hyperthyroidism, thyroid nodules, thyroiditis, and cancer, are among the most prevalent endocrine disorders, posing significant health risks, which need to be diagnosed treated promptly. Traditional diagnostic approaches, reliant on manual interpretation of medical images, time-consuming prone errors. This study introduces a novel deep learning framework utilizing advanced Convolutional Neural Networks (CNNs), specifically modified ResNet InceptionV3 architectures, improve accuracy efficiency disease diagnosis. We present Dual-OptNet, new hybrid architecture that effectively merges skip connections with multi-scale feature extraction based for lung classification tasks. Dual-OptNet shows accurate generalizability results in classifying an average best 97% from dual-step optimized using Adam SGD. Future work will focus developing real-time tool demonstrate potential utility this model clinical context. also enhancing dataset cover wider range uncommon cases, incorporating explainable AI methods, so decisions more interpretable. Further research explore ultrasound analysis multi-modal data integration, such as combining images patient history, enhance accuracy. Deploying system environments key validating its impact scalability, ultimately contributing efficient healthcare solutions
Язык: Английский
Процитировано
0Sensors, Год журнала: 2024, Номер 24(6), С. 1939 - 1939
Опубликована: Март 18, 2024
With the development of deep learning and sensors sensor collection methods, computer vision inspection technology has developed rapidly. The deep-learning-based classification algorithm requires acquisition a model with superior generalization capabilities through utilization substantial quantity training samples. However, due to issues such as privacy, annotation costs, sensor-captured images, how make full use limited samples become major challenge for practical deployment. Furthermore, when simulating models transferring them actual image scenarios, discrepancies often arise between common sets target domain (domain offset). Currently, meta-learning offers promising solution few-shot problems. supporting set data on remains limited, leading cross-domain effectiveness. To address this challenge, we have self-distillation mixing (SDM) method utilizing Teacher–Student framework. This effectively transfers knowledge from source by applying techniques mixed augmentation, better representations relatively abundant datasets, achieving fine-tuning in domain. In comparison nine classical models, experimental results demonstrate that SDM excels terms time accuracy. domain, even number
Язык: Английский
Процитировано
0Опубликована: Янв. 1, 2024
Язык: Английский
Процитировано
0Internet of Things, Год журнала: 2024, Номер unknown, С. 101456 - 101456
Опубликована: Ноя. 1, 2024
Язык: Английский
Процитировано
0