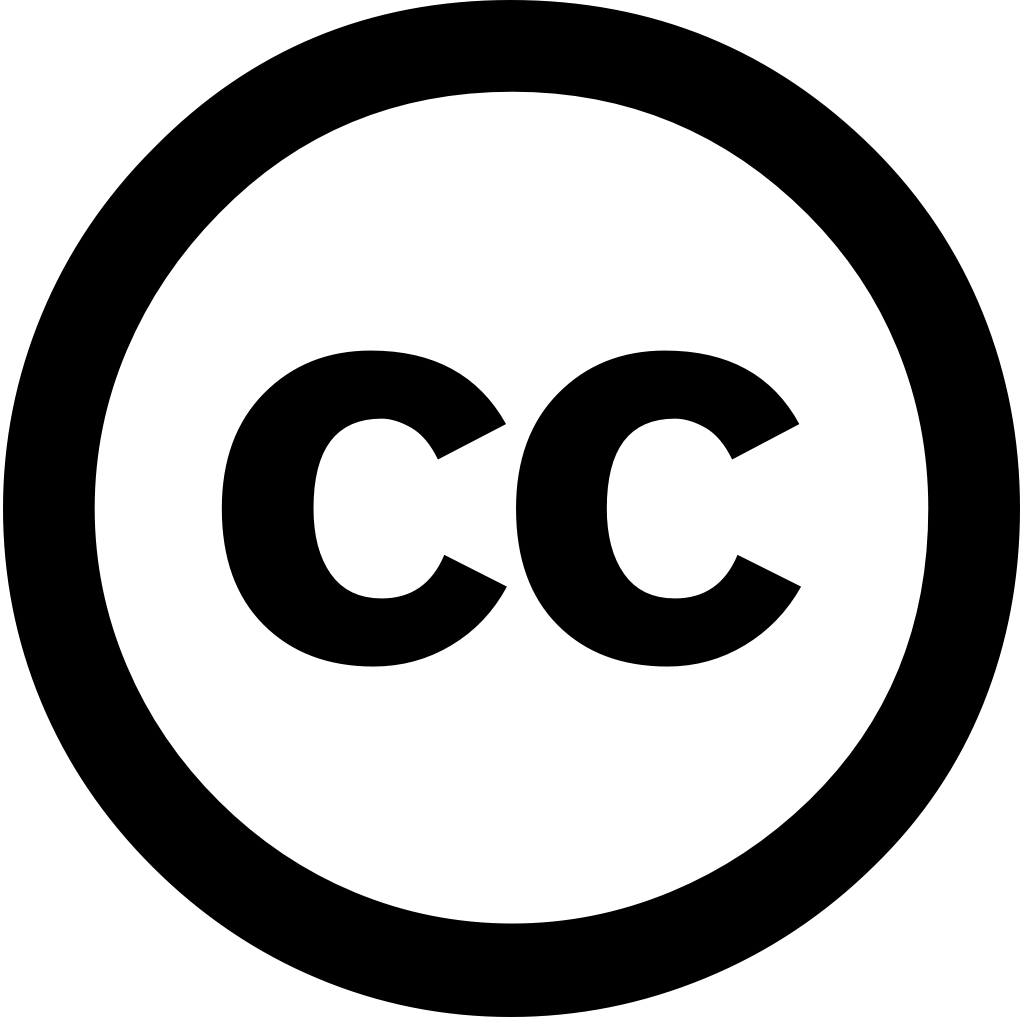
Applied Sciences, Год журнала: 2025, Номер 15(5), С. 2811 - 2811
Опубликована: Март 5, 2025
The impact of immediate emotions on human decision-making has been extensively studied; however, their role within human–machine interactive systems remains underexplored. This study investigates how emotional states influence behaviors in such by introducing a novel two-tiered progressive inquiry model—environment–emotion and emotion–decision. Using Kuhn poker as an experimental context, we developed intelligent decision support system based Counterfactual Regret Minimization algorithms to provide real-time strategic advice participants. Our findings reveal that positive lead individuals be more risk-seeking less inclined collaborate with the AI-driven system, whereas negative result risk-averse tendencies higher compliance recommendations. By systematically uncovering causal mechanisms through which environmental factors regulate subsequently affect decision-making, this research provides critical theoretical empirical insights for optimizing interaction design.
Язык: Английский