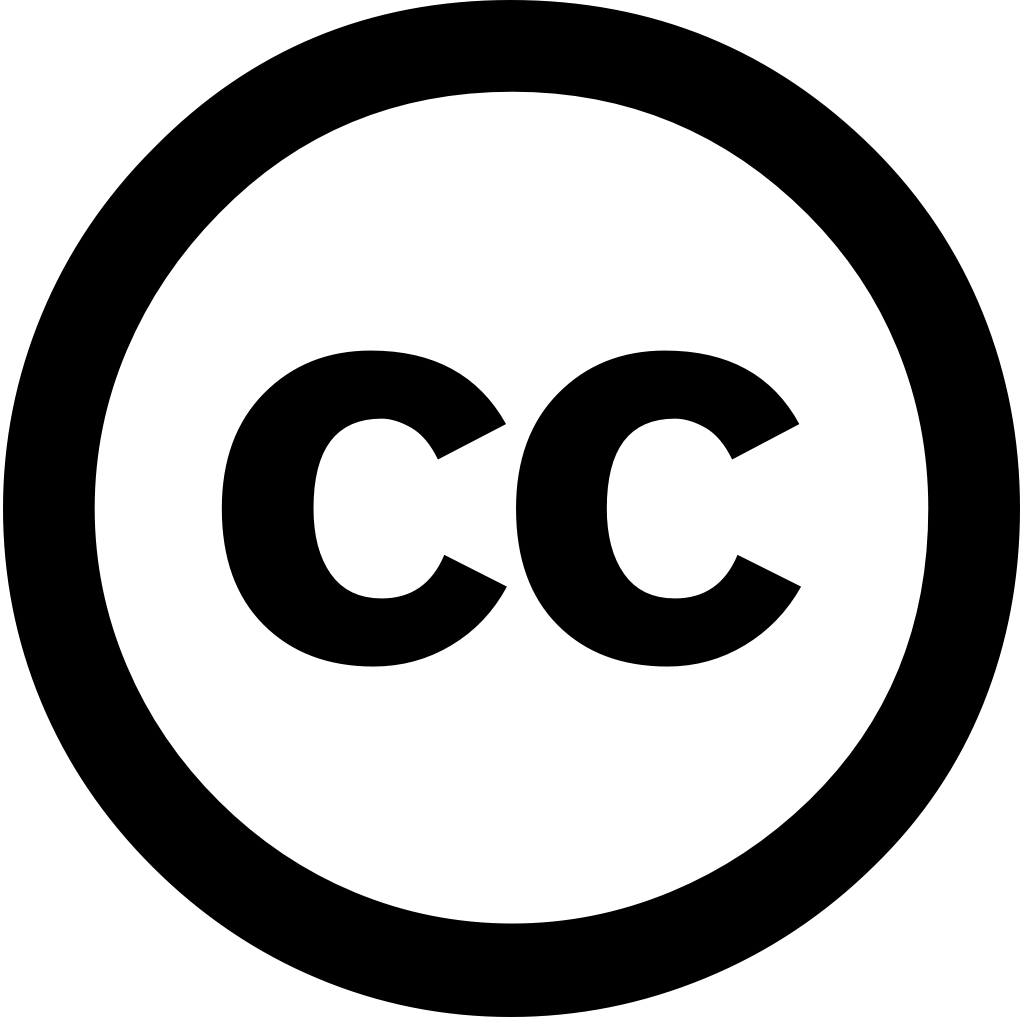
Scientific Reports, Год журнала: 2024, Номер 14(1)
Опубликована: Дек. 30, 2024
Accurate estimation of the soil resilient modulus (M
Язык: Английский
Scientific Reports, Год журнала: 2024, Номер 14(1)
Опубликована: Дек. 30, 2024
Accurate estimation of the soil resilient modulus (M
Язык: Английский
Modeling Earth Systems and Environment, Год журнала: 2025, Номер 11(1)
Опубликована: Янв. 14, 2025
Язык: Английский
Процитировано
1Scientific Reports, Год журнала: 2025, Номер 15(1)
Опубликована: Фев. 11, 2025
Predicting the compressive strength of Compressed Earth Blocks (CEB) is a challenging task due to nonlinear relationships among their diverse components, including cement, clay, sand, silt, and fibers. This study employed PyCaret, an automated machine learning platform, address this complexity by developing evaluating predictive models. The analysis demonstrated that fiber content exhibited strong positive correlation with cement content, coefficient 0.9444, indicating significant influence on strength. Multiple algorithms were tested using metrics such as determination (R2), root mean square error (RMSE), absolute (MAE) assess model performance. Among these, Extra Trees Regressor showed best capability R2 = 0.9444 (highly accurate predictions), RMSE 0.4909 (low variability in prediction errors) MAE 0.1899 (minimal average error). results confirm PyCaret effectively automates workflow, enabling modeling complex material behavior. outperformed other its ability handle highly multivariate datasets, making it particularly well-suited for predicting CEB. approach offers advantage over traditional laboratory testing, which time-consuming resource-intensive. By incorporating techniques, especially PyCaret's streamlined processes, CEB becomes more efficient reliable, providing practical tool engineers researchers science.
Язык: Английский
Процитировано
1Scientific Reports, Год журнала: 2025, Номер 15(1)
Опубликована: Янв. 2, 2025
Soil classification and analysis are essential for understanding soil properties serve as a foundation various engineering projects. Traditional methods of rely heavily on costly time-consuming laboratory in-situ tests. In this study, Support Vector Machine (SVM) models were trained using 649 Cone Penetration Test (CPT) datasets, specifically utilizing cone tip resistance ( $$q_c$$ ) sleeve friction $$f_s$$ input variables. Pearson correlation sensitivity confirmed that these variables highly correlated with the results. To enhance performance, 25 optimization algorithms applied, validated against an independent dataset 208 CPT records. The results revealed 23 successfully improved SVM accuracy. Among these, 18 achieved higher accuracy than current standard, "Code Measurement Railway Engineering Geology." Notably, Thermal Exchange Optimization (TEO) algorithm resulted in most significant improvement, increasing original model by 10% exceeding standard 4.3%. Moreover, thoroughly evaluated Monte Carlo simulations, confusion matrices, ROC curves, 10 key performance metrics. conclusion, integrating evolutionary offers promising approach to enhancing efficiency applications.
Язык: Английский
Процитировано
0Indian geotechnical journal, Год журнала: 2025, Номер unknown
Опубликована: Апрель 24, 2025
Язык: Английский
Процитировано
0Scientific Reports, Год журнала: 2024, Номер 14(1)
Опубликована: Дек. 30, 2024
Язык: Английский
Процитировано
3Environmental Earth Sciences, Год журнала: 2025, Номер 84(5)
Опубликована: Фев. 21, 2025
Язык: Английский
Процитировано
0Agriculture, Год журнала: 2025, Номер 15(5), С. 482 - 482
Опубликована: Фев. 24, 2025
The spectral information extracted from hyperspectral images is characterized by redundancy and complexity, while the morphological features help to simplify data provide rich about material composition. This study based on using quantitatively detect water content of winter jujubes, it extends research scope composite effect basis previous research. Firstly, a multiple linear regression analysis was carried out different characteristic bands. Secondly, terms with high significance levels were used as variables be fused wavelength for fusion. Finally, partial least squares model established jujubes. results show that quantitative relationship can between morphology characteristics coefficients determination equations under bands center wavelengths 1024 nm, 1146 1348 1405 nm 0.8449, 0.7944, 0.7479, 0.9477, respectively. After fusing features, modeling effects all improved. optimal fusion at correlation coefficient 0.9942 calibration set 0.8698 prediction set. overall showed wave valley more reflective fruit quality, are suitable than those peak detection moisture
Язык: Английский
Процитировано
0Multiscale and Multidisciplinary Modeling Experiments and Design, Год журнала: 2025, Номер 8(5)
Опубликована: Март 19, 2025
Язык: Английский
Процитировано
0Scientific Reports, Год журнала: 2025, Номер 15(1)
Опубликована: Апрель 26, 2025
In this research, our objective was to utilize different machine learning techniques, such as XGBoost, Extra Trees, CatBoost, and Multiple Linear Regression (MLR), model the heating values of municipal solid waste. The input parameters considered for constructed models included weight dry sample (kg) content carbon (C), hydrogen (H), oxygen (O), nitrogen (N), sulfur (S), ash in kg. Trees model, fine-tuned hyperparameters, demonstrated outstanding performance, achieving R2 0.999 training set 0.979 testing set. Notably, has shown robust accuracy, evidenced by a low Mean Squared Error (MSE) 77,455.92 on dataset. Furthermore, Absolute (MAE) Percentage (MAPE) were 245.886 16.22%, respectively, further proving model's substantial predictive accuracy reliability. Although XGBoost CatBoost strong capabilities with high values, outperformed them significantly lower error metrics. On contrary, MLR, utilized conventional technique, moderate suggesting distinct trade-off between explanatory power accuracy. feature importance examination optimal emerged most impactful factor, succeeded content, descending hierarchy significance.
Язык: Английский
Процитировано
0Emergent Materials, Год журнала: 2025, Номер unknown
Опубликована: Май 9, 2025
Язык: Английский
Процитировано
0