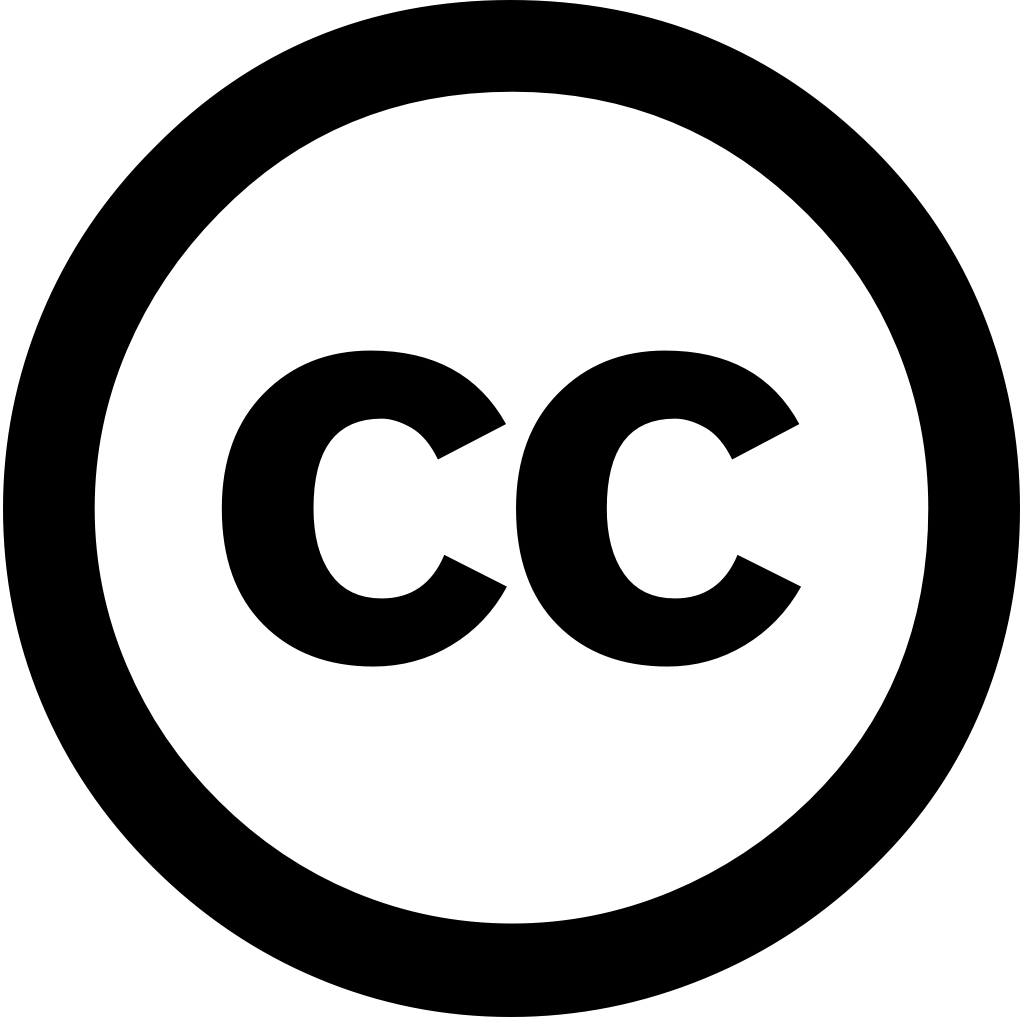
Chemical Engineering Journal, Год журнала: 2025, Номер unknown, С. 162604 - 162604
Опубликована: Апрель 1, 2025
Язык: Английский
Chemical Engineering Journal, Год журнала: 2025, Номер unknown, С. 162604 - 162604
Опубликована: Апрель 1, 2025
Язык: Английский
Sensors, Год журнала: 2025, Номер 25(2), С. 527 - 527
Опубликована: Янв. 17, 2025
To automate the quality control of painted surfaces heating devices, an automatic defect detection and classification system was developed by combining deflectometry bright light-based illumination on image acquisition, deep learning models for non-defective (OK) defective (NOK) that fused dual-modal information at decision level, online network dispatching visualization. Three decision-making algorithms were tested implementation: a new model built trained from scratch transfer pre-trained networks (ResNet-50 Inception V3). The results revealed two modes employed widened type defects could be identified with this system, while maintaining its lower computational complexity performing multi-modal fusion level. Furthermore, achieved higher accuracies compared to self-built network, ResNet-50 displaying accuracy. inspection consistently obtained fast accurate surface classifications because it imposed OK images both modes. then successfully sent server forwarded graphical user interface showed considerable robustness, demonstrating potential as efficient tool industrial control.
Язык: Английский
Процитировано
2Tourism Management, Год журнала: 2025, Номер 110, С. 105180 - 105180
Опубликована: Март 10, 2025
Язык: Английский
Процитировано
2Journal of Environmental Management, Год журнала: 2024, Номер 356, С. 120564 - 120564
Опубликована: Март 12, 2024
Robust quantification of vegetative biomass using satellite imagery one or more forms machine learning (ML) has hitherto been hindered by the extent and quality training data. Here, we showcase how ML predictive demonstrably improves when additional data is used. We collated field datasets pasture obtained via destructive sampling, 'C-Dax' reflective measurements rising plate meters (RPM) from ten livestock farms across four States in Australia. Remotely sensed Sentinel-2 constellation was used to retrieve aboveground a novel paradigm hereafter termed "SPECTRA-FOR" (Spectral Pasture Estimation Combined Techniques Random-forest Algorithm for Features Optimisation Retrieval). Using this framework, show that low temporal resolution high latitude regions with persistent cloud cover leads extensive gaps between cloud-free images, hindering model performance and, thus, contemporaneous ability forecast real-time biomass. By leveraging spectral consistency Planet Lab SuperDove overcome limitation, bands Sentinel-2, as proxy pre-2022 (referred synthetic SSD), actual (ASD), given higher frequent passage compared Sentinel-2. their respective input features SPECRA-FOR, were R
Язык: Английский
Процитировано
9Remote Sensing, Год журнала: 2025, Номер 17(2), С. 215 - 215
Опубликована: Янв. 9, 2025
Most current hyperspectral image classification (HSIC) models require a large number of training samples, and when the sample size is small, performance decreases. To address this issue, we propose an innovative model that combines orthogonal capsule network with meta-reinforcement learning (OCN-MRL) for small HSIC. The OCN-MRL framework employs Meta-RL feature selection CapsNet data sample. module through clustering, augmentation, multiview techniques enables to adapt new HSIC tasks limited samples. Learning meta-policy Q-learner generalizes across different effectively select discriminative features from data. Integrating orthogonality into reduces complexity while maintaining ability preserve spatial hierarchies relationships in 3D convolution layer, suitably capturing complex patterns. Experimental results on four rich Chinese datasets demonstrate model’s competitiveness both higher accuracy less computational cost compared existing CapsNet-based methods.
Язык: Английский
Процитировано
1Опубликована: Март 22, 2024
Язык: Английский
Процитировано
6Alexandria Engineering Journal, Год журнала: 2024, Номер 103, С. 327 - 342
Опубликована: Июнь 18, 2024
Язык: Английский
Процитировано
5Journal of Sensor and Actuator Networks, Год журнала: 2024, Номер 13(5), С. 53 - 53
Опубликована: Сен. 4, 2024
Tool condition monitoring (TCM) systems have evolved into an essential requirement for contemporary manufacturing sectors of Industry 4.0. These employ sensors and diverse techniques to swiftly identify diagnose tool wear, defects, malfunctions computer numerical control (CNC) machines. Their pivotal role lies in augmenting lifespan, minimizing machine downtime, elevating productivity, thereby contributing industry growth. However, the efficacy CNC TCM hinges upon multiple factors, encompassing system type, data precision, reliability, adeptness analysis. Globally, extensive research is underway enhance real-time efficiency. This review focuses on significance attributes proficient turning centers. It underscores TCM’s paramount outlines challenges linked processing Moreover, elucidates various variants, including cutting force, acoustic emission, vibration, temperature systems. Furthermore, integration industrial Internet things (IIoT) learning (ML) are also explored. article concludes by underscoring ongoing necessity development technology empower modern intelligent industries operate at peak
Язык: Английский
Процитировано
5mAbs, Год журнала: 2025, Номер 17(1)
Опубликована: Янв. 8, 2025
In-silico prediction of protein biophysical traits is often hindered by the limited availability experimental data and their heterogeneity. Training on can lead to overfitting poor generalizability sequences distant from those in training set. Additionally, inadequate use scarce disparate introduce biases during evaluation, leading unreliable model performances being reported. Here, we present a comprehensive study exploring various approaches for fitness data, leveraging pre-trained embeddings, repeated stratified nested cross-validation, ensemble learning ensure an unbiased assessment performances. We applied our framework NanoMelt, predictor nanobody thermostability trained with dataset 640 measurements apparent melting temperature, obtained integrating literature 129 new this study. find that stacking multiple regression using diverse sequence embeddings achieves state-of-the-art accuracy predicting thermostability. further demonstrate NanoMelt's potential streamline development guiding selection highly stable nanobodies. make curated freely available NanoMelt accessible as downloadable software webserver.
Язык: Английский
Процитировано
0Neural Computing and Applications, Год журнала: 2025, Номер unknown
Опубликована: Фев. 8, 2025
Язык: Английский
Процитировано
0Electronics, Год журнала: 2025, Номер 14(5), С. 968 - 968
Опубликована: Фев. 28, 2025
Mass spectrometry (MS) data present challenges for machine learning (ML) classification due to their high dimensionality, complex feature distributions, batch effects, and intensity discrepancies, often hindering model generalization efficiency. To address these issues, this study introduces the Efficient Quick 1D Lite Convolutional Neural Network (CNN) Ensemble Classifier (EQLC-EC), integrating convolutional networks with reshape layers dual voting mechanisms enhanced representation performance. Validation was performed on five publicly available MS datasets, each featured in high-impact publications. EQLC-EC underwent comprehensive evaluation against classical models (e.g., support vector (SVM), random forest) leading deep methods reported studies. demonstrated dataset-specific improvements, including accuracy (1–5% increase) reduced standard deviation (1–10% reduction). Performance differences between soft hard were negligible (<1% variation deviation). presents a powerful efficient tool analysis potential applications across metabolomics proteomics.
Язык: Английский
Процитировано
0