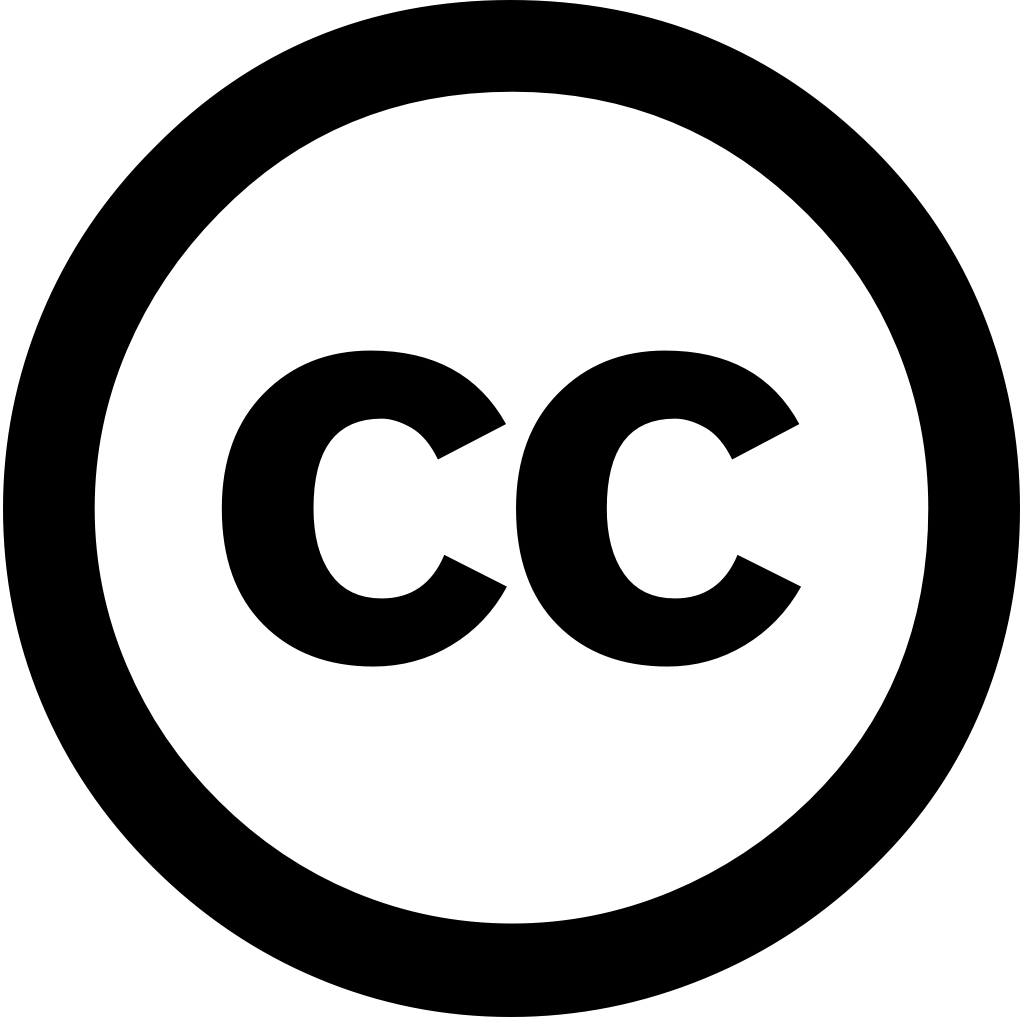
IEEE Transactions on Neural Systems and Rehabilitation Engineering, Год журнала: 2025, Номер 33, С. 522 - 531
Опубликована: Янв. 1, 2025
Accurate understanding of muscle activation and forces plays an essential role in neuro-rehabilitation musculoskeletal disorder treatments. Computational modeling has been widely used as a powerful non-invasive tool to estimate them through inverse dynamics using static optimization, but the inherent computational complexity results time-consuming analysis. In this paper, we propose knowledge-based deep learning framework for time-efficient dynamic analysis, which can predict from joint kinematic data directly while not requiring any label information during model training. The Bidirectional Gated Recurrent Unit (BiGRU) neural network is selected backbone our due its proficient handling time-series data. Prior physical knowledge forward pre-selected based physiological criteria are integrated into loss function guide training networks. Experimental validations on two datasets, including one benchmark upper limb movement dataset self-collected lower six healthy subjects, performed. experimental have shown that BiGRU architecture outperforms other models when trained specifically designed function, illustrates effectiveness robustness proposed framework.
Язык: Английский