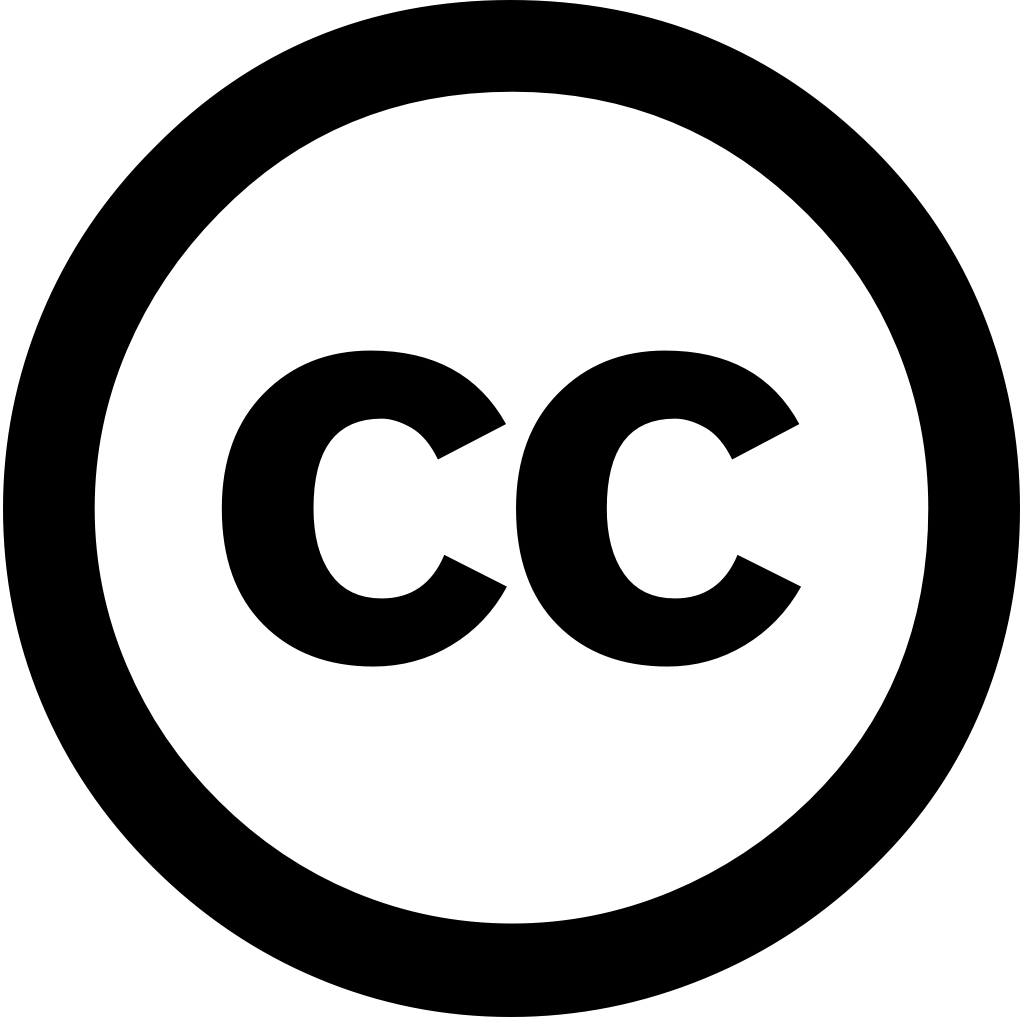
Lecture notes in civil engineering, Год журнала: 2025, Номер unknown, С. 801 - 811
Опубликована: Янв. 1, 2025
Язык: Английский
Lecture notes in civil engineering, Год журнала: 2025, Номер unknown, С. 801 - 811
Опубликована: Янв. 1, 2025
Язык: Английский
Cleaner Engineering and Technology, Год журнала: 2023, Номер 13, С. 100604 - 100604
Опубликована: Фев. 13, 2023
Replacing Ordinary Portland Cement (OPC) with industrial waste like Ground Granulated Blast Furnace Slag (GGBFS) has been proven to have remarkable benefits regarding the mechanical properties of concrete and environment. The main objectives this research, as a result, are (a) develop generalized, accurate, optimized Machine Learning (ML)-based model for predicting compressive strength incorporating GGBFS (b) propose equations easier calculation containing GGBFS. To aim, various ML-based methods, namely Decision Tree (DT), Random Forest (RF), Support Vector (SVM), K-nearest Neighbors (KNN), Artificial Neural Network (ANN) were considered An extensive dataset including 625 results experimental studies was collected from international peer-reviewed publications. divided into two sub-datasets: training (85%), used train models on relationship between input output parameters, testing (15%), evaluate accuracy models. most influential ordinary cement, grade, cement ratio, water, coarse aggregate, fine age, variables proposing prediction predicted actual values compared in each model. also using common performance metrics (RMSE, MSE, MAE, MAPE, R, R2-score) Taylor diagram. Eventually, sensitivity analysis conducted at end study explore influence ratio grade strength, consequently, suggested based results.
Язык: Английский
Процитировано
53Transportation Research Part D Transport and Environment, Год журнала: 2024, Номер 133, С. 104276 - 104276
Опубликована: Июнь 5, 2024
Язык: Английский
Процитировано
22Construction and Building Materials, Год журнала: 2023, Номер 400, С. 132814 - 132814
Опубликована: Авг. 10, 2023
Язык: Английский
Процитировано
32Ecological Informatics, Год журнала: 2023, Номер 77, С. 102253 - 102253
Опубликована: Авг. 9, 2023
Язык: Английский
Процитировано
25Building and Environment, Год журнала: 2024, Номер 264, С. 111922 - 111922
Опубликована: Авг. 8, 2024
Life Cycle Assessment is necessary for evaluating the environmental impacts of buildings throughout their life cycle, considering factors such as energy consumption, emissions, and resource utilization. However, Dynamic introduces a temporal dimension, acknowledging that building's performance evolves due to technological advancements, occupancy behavior, changing conditions. This paper reviews DLCA, focusing on uncertainties arising from parameter, scenario, model variability, emphasizes integration technologies like Building Information Modeling, Internet Things, machine learning enhance real-time data collection predictive analytics. An extensive review 430 papers, refined 180, reveals 55 % publications are in sciences, with significant contributions United Kingdom (27.8 %), France (24.1 China (18.1 %). Key findings include variations embodied greenhouse gas emissions materials aluminum dynamic aspects transportation impacts, which extend beyond traditional metrics operational efficiency over time. Uncertainties all LCA stages (A1 D) addressed, service life, water use, needs. Advanced methodologies, including proposed framework hybrid approach integrates process-based input-output methods, suggested comprehensiveness assessments. The monitoring analytics further improves adaptability precision models, emphasizing necessity continuous updates scenario analyses capture future conditions accurately. study paves way research aimed at mitigating major sources uncertainty, promoting more sustainable building practices, advancing field LCA.
Язык: Английский
Процитировано
14Management Systems in Production Engineering, Год журнала: 2024, Номер 32(1), С. 33 - 44
Опубликована: Фев. 26, 2024
Abstract Environmental management systems (EMS) are essential in promoting sustainable practices and mitigating the adverse effects of human activities on environment. As technology continues to advance, there is an increasing opportunity utilize advanced technologies improve environmental systems. This article examines potential different technologies, such as artificial intelligence (AI), blockchain, big data, Internet Things (IoT), within context intends offer valuable insights researchers, practitioners, policymakers by examining uses AI, IoT The goal demonstrate how these can be leveraged enhance sustainability, boost performance, yield favourable results across sectors industries.
Язык: Английский
Процитировано
10Infrastructures, Год журнала: 2024, Номер 9(12), С. 225 - 225
Опубликована: Дек. 7, 2024
This study explores the growing influence of artificial intelligence (AI) on structural health monitoring (SHM), a critical aspect infrastructure maintenance and safety. begins with bibliometric analysis to identify current research trends, key contributing countries, emerging topics in AI-integrated SHM. We examine seven core areas where AI significantly advances SHM capabilities: (1) data acquisition sensor networks, highlighting improvements technology collection; (2) processing signal analysis, techniques enhance feature extraction noise reduction; (3) anomaly detection damage identification using machine learning (ML) deep (DL) for precise diagnostics; (4) predictive maintenance, optimize scheduling prevent failures; (5) reliability risk assessment, integrating diverse datasets real-time analysis; (6) visual inspection remote monitoring, showcasing role AI-powered drones imaging systems; (7) resilient adaptive infrastructure, enables systems respond dynamically changing conditions. review also addresses ethical considerations societal impacts SHM, such as privacy, equity, transparency. conclude by discussing future directions challenges, emphasizing potential efficiency, safety, sustainability systems.
Язык: Английский
Процитировано
8Lecture notes in civil engineering, Год журнала: 2025, Номер unknown, С. 589 - 598
Опубликована: Янв. 1, 2025
Язык: Английский
Процитировано
1International Journal on Interactive Design and Manufacturing (IJIDeM), Год журнала: 2025, Номер unknown
Опубликована: Янв. 15, 2025
Язык: Английский
Процитировано
1Cleaner Engineering and Technology, Год журнала: 2025, Номер unknown, С. 100899 - 100899
Опубликована: Янв. 1, 2025
Язык: Английский
Процитировано
1