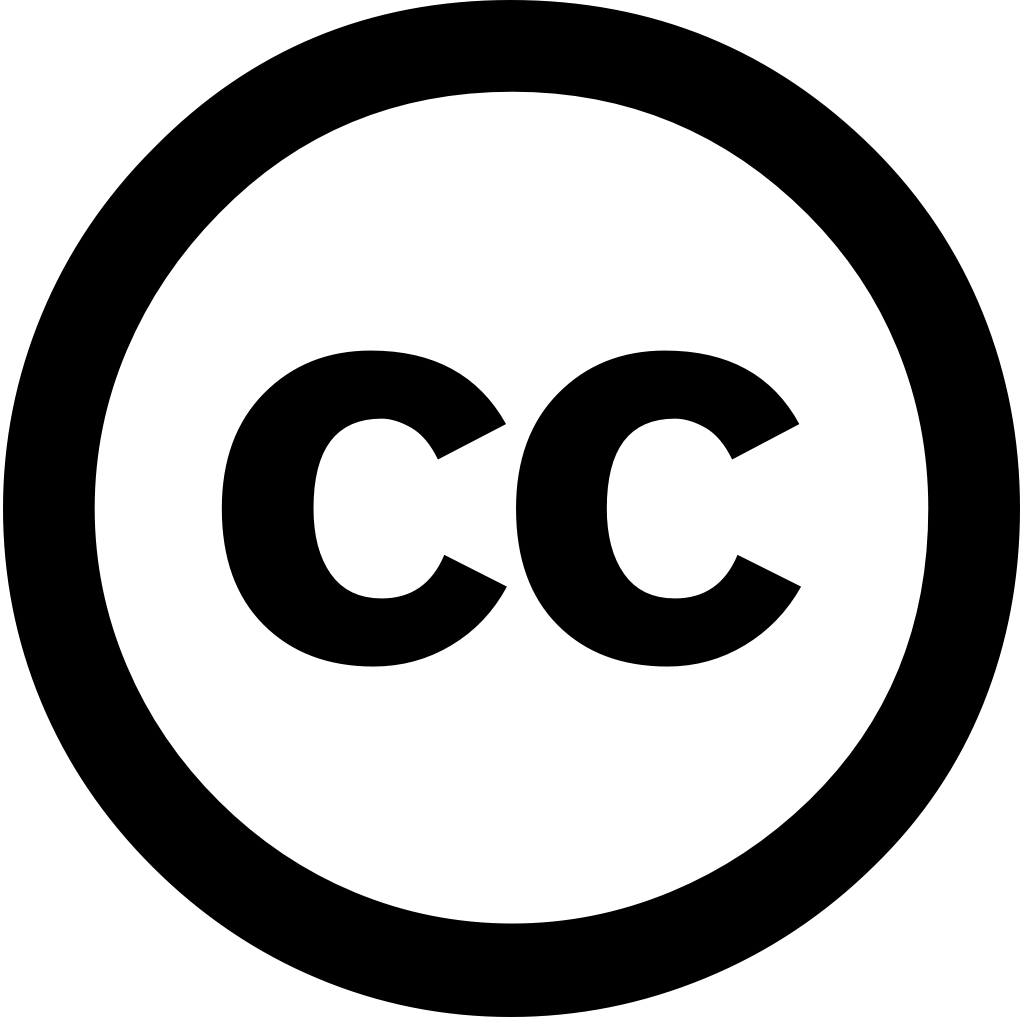
Ecological Indicators, Год журнала: 2024, Номер 169, С. 112915 - 112915
Опубликована: Дек. 1, 2024
Язык: Английский
Ecological Indicators, Год журнала: 2024, Номер 169, С. 112915 - 112915
Опубликована: Дек. 1, 2024
Язык: Английский
Water, Год журнала: 2024, Номер 16(19), С. 2748 - 2748
Опубликована: Сен. 27, 2024
Groundwater salinization poses a critical threat to sustainable development in arid and semi-arid rurbanizing regions, exemplified by Kerman Province, Iran. This region experiences groundwater ecosystem degradation as result of the rapid conversion rural agricultural land urban areas under chronic drought conditions. study aims enhance Pollution Risk (GwPR) mapping integrating DRASTIC index with machine learning (ML) models, including Random Forest (RF), Boosted Regression Trees (BRT), Generalized Linear Model (GLM), Support Vector Machine (SVM), Multivariate Adaptive Splines (MARS), alongside hydrogeochemical investigations, promote water management Province. The RF model achieved highest accuracy an Area Under Curve (AUC) 0.995 predicting GwPR, outperforming BRT (0.988), SVM (0.977), MARS (0.951), GLM (0.887). RF-based map identified new high-vulnerability zones northeast northwest showed expanded moderate vulnerability zone, covering 48.46% area. Analysis revealed exceedances WHO standards for total hardness (TH), sodium, sulfates, chlorides, electrical conductivity (EC) these areas, indicating contamination from mineralized aquifers unsustainable practices. findings underscore model’s effectiveness prediction highlight need stricter monitoring management, regulating extraction improving use efficiency riverine aquifers.
Язык: Английский
Процитировано
9Process Safety and Environmental Protection, Год журнала: 2025, Номер unknown, С. 106781 - 106781
Опубликована: Янв. 1, 2025
Язык: Английский
Процитировано
1Environmental Monitoring and Assessment, Год журнала: 2025, Номер 197(2)
Опубликована: Янв. 6, 2025
Язык: Английский
Процитировано
0Applied Geomatics, Год журнала: 2025, Номер unknown
Опубликована: Янв. 14, 2025
Язык: Английский
Процитировано
0Stochastic Environmental Research and Risk Assessment, Год журнала: 2025, Номер unknown
Опубликована: Фев. 26, 2025
Язык: Английский
Процитировано
0Frontiers in Built Environment, Год журнала: 2024, Номер 10
Опубликована: Авг. 30, 2024
The escalating challenges of global climate change have made the development low-carbon cities—urban areas committed to reducing carbon emissions through sustainable energy use, enhanced building efficiency, and transport solutions—a critical area study. However, there remains a significant gap in systematic review thematic evolution emerging frontiers within this field. This study addresses by analyzing data from Web Science database, initially retrieving 1,743 articles articles. Following PRISMA guidelines, we refined selection 1,648 high-quality publications. Using tools such as CiteSpace VOSviewer, conducted an in-depth analysis identify core authors, prolific countries/regions, leading institutions, key journals. Our revealed three evolutionary stages research on international city development. Additionally, identified seven predominant topics recent studies: land emissions, ecological environment quality, ecosystem services, human health, consumption, economic costs. These findings contribute clearer more comprehensive framework for cities, serving valuable reference scholars practitioners involved both theoretical practical aspects
Язык: Английский
Процитировано
2Modeling Earth Systems and Environment, Год журнала: 2024, Номер 10(4), С. 5257 - 5271
Опубликована: Июнь 14, 2024
Язык: Английский
Процитировано
1Geographia Technica, Год журнала: 2024, Номер 19(2/2024), С. 13 - 32
Опубликована: Май 15, 2024
Population growth, urbanization and rapid industrial development increase the demand for water resources.Groundwater is an important resource in sustainable socio-economic development.The identification of regions with probability existence groundwater necessary helping decision makers to propose effective strategies management this resource.The objective study construct maps potential groundwater, based on machine learning algorithms, namely deep neural networks (DNNs), XGBoost (XGB), CatBoost (CB), Gia Lai province Vietnam.In study, 12 conditioning factors, elevation, aspect, curvature, slope, soil type, river density, distance road, land use/land cover (LULC), Normalized Difference Vegetation Index (NDVI), Normal Built-up (NDBI), Water (NDWI), rainfall were used, along 181 inventory points, models.The proposed models evaluated using receiver operating characteristic (ROC) curve, area under curve (AUC), root-mean-square error (RMSE), mean absolute (MAE).The results showed that predictions most accurate XGB model; CB came second, DNN was performed least well.About 4,990 km² found be category very low potential; 3,045 category; 2,426 classified as moderate, 2,665 high, 2,007 high.The methodology used creating maps.This approach, can provide valuable information factors influencing assist decisionmakers or developers managing resources sustainably.It also supports territory, including tourism.This other geographic a small change input data.
Язык: Английский
Процитировано
0Physics and Chemistry of the Earth Parts A/B/C, Год журнала: 2024, Номер 136, С. 103728 - 103728
Опубликована: Сен. 6, 2024
Язык: Английский
Процитировано
0Journal of Environmental Management, Год журнала: 2024, Номер 371, С. 123155 - 123155
Опубликована: Ноя. 2, 2024
Язык: Английский
Процитировано
0