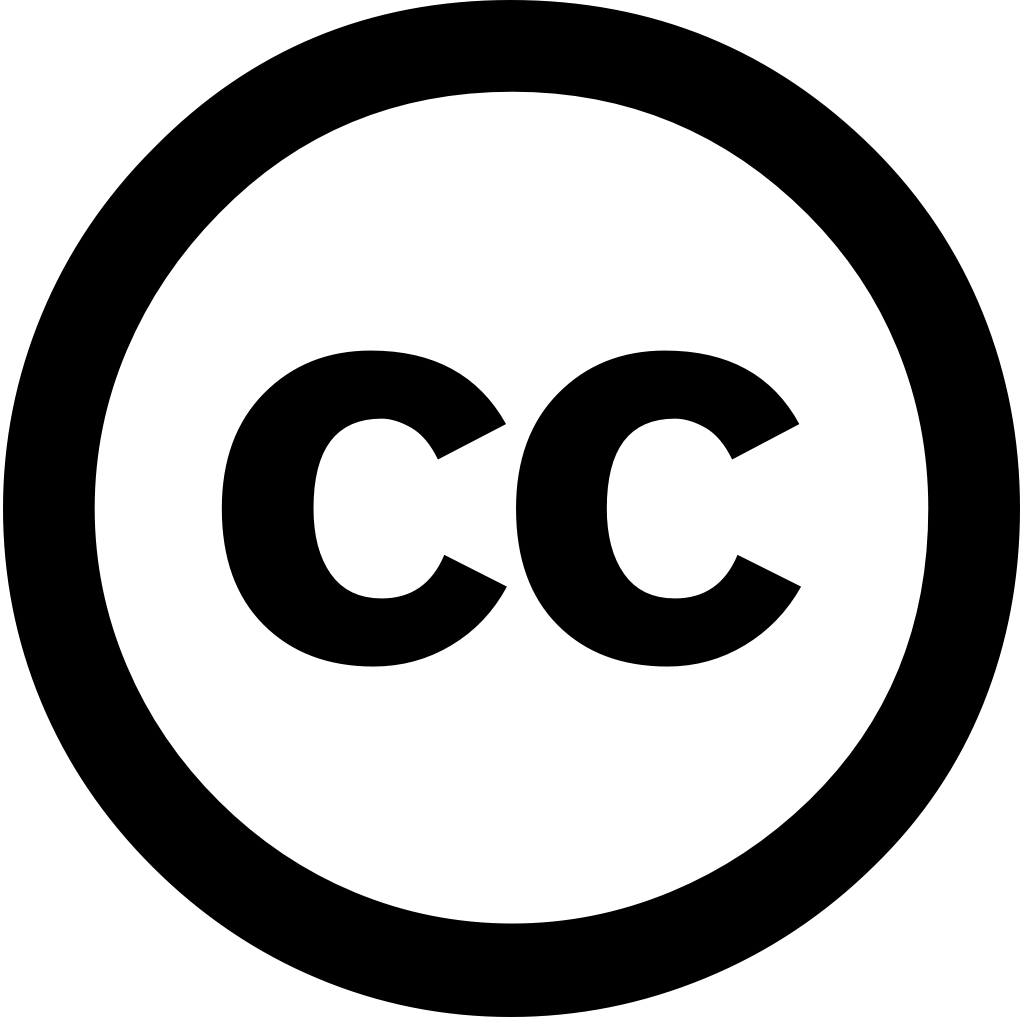
Agronomy, Год журнала: 2024, Номер 14(12), С. 3039 - 3039
Опубликована: Дек. 19, 2024
The accurate prediction of the spatial variability for soil water content (SWC) in farmland is essential resource management and sustainable agricultural development. However, natural factors introduce uncertainty result poor alignment when predicting SWC, leading to low accuracy. To address this, this study introduced a novel indicator: landscape indices. These indices include largest patch index (LPI), edge density (ED), aggregation (AI), cohesion (COH), contagion (CON), division (DIV), percentage like adjacencies (PLA), Shannon evenness (SHEI), diversity (SHDI). A Bayesian optimization–deep forest (BO–DF) model was developed leverage these SWC. Statistical analysis revealed that exhibited skewed distributions weak linear correlations with SWC (r < 0.2). Despite incorporating variables into BO–DF significantly improved accuracy, R2 increasing by 35.85%. This demonstrated robust nonlinear fitting capability Spatial mapping using indicated high-value areas were predominantly located eastern southern regions Yellow River Delta China. Furthermore, SHapley additive explanation (SHAP) highlighted key drivers findings underscore potential as valuable prediction, supporting regional strategies
Язык: Английский